Data Science And AI
Explore the foundations, applications, and future of Data Science and AI, illustrating its significant impact on technology and industry. Deep dive into real-world case studies and emerging trends.
Data Science and Artificial Intelligence (AI) have become cornerstones of technological innovation, driving significant change across various sectors. Data Science refers to the multidisciplinary approach of extracting insights and knowledge from structured and unstructured data through a blend of statistics, machine learning, and computer science. It involves collecting, processing, analyzing, and interpreting vast amounts of data to solve complex problems. Meanwhile, AI is the simulation of human intelligence processes by machines, especially computer systems. This encompasses everything from natural language processing and speech recognition to robotics and computer vision.
The integration of these two fields is proving revolutionary in many industries. For instance, in the healthcare sector, AI-powered diagnostic tools are enhancing the accuracy and speed of disease detection, thereby improving patient outcomes. In finance, AI algorithms are being used for fraud detection and to provide personalized banking experiences. In manufacturing, predictive analytics, a subset of Data Science, is optimizing supply chain management and reducing downtime through predictive maintenance.
The importance of Data Science and AI in today's tech landscape cannot be overstated. According to a report by the International Data Corporation (IDC), worldwide spending on AI systems is expected to reach $110 billion by 2024, highlighting the significant investments being made into these technologies. Furthermore, organizations that leverage Data Science and AI effectively can experience up to a 20% increase in profitability, as noted by a study from McKinsey Global Institute.
The symbiotic relationship between Data Science and AI is foundational to their impact. Data Science provides the data and the analytics necessary to train AI systems, while AI automates and enhances Data Science processes, enabling more sophisticated analysis and data-driven decision-making. This synergy is driving unprecedented innovation, making it crucial for professionals and project managers to understand how to deploy these technologies effectively. By integrating Data Science and AI into their strategic planning, leaders can unlock new levels of efficiency, customer insight, and competitive edge.
Build powerful workflows with Meegle for free!
Historical background of data science and ai
The journey of Data Science and AI from their inception to the present day is marked by a series of groundbreaking milestones that have collectively shaped the digital era. Understanding this historical context enriches our appreciation of the current innovations and helps project managers anticipate future trends and applications.
The origins of Data Science can be traced back to the 1960s when the term "data science" itself was not yet coined. During this period, statisticians began exploring methods to analyze and interpret data more effectively. The advent of computing power in the 1970s and 1980s allowed for more sophisticated data analysis techniques, paving the way for modern Data Science. The term "Data Science" gained prominence in the early 2000s as a way to describe the emerging field that combined statistics with computer science to handle large data sets.
Conversely, the roots of AI date back to the 1950s when computer scientists like Alan Turing and John McCarthy began to theorize about machines that could perform tasks requiring human intelligence. The 1956 Dartmouth Conference is often credited as the birth of AI as a field, where it was defined as the science and engineering of making intelligent machines. The subsequent decades saw significant advancements, including the development of expert systems in the 1980s and the emergence of machine learning algorithms in the 1990s.
Several milestones have marked the evolution of both fields. In 1997, IBM's Deep Blue made headlines by defeating world chess champion Garry Kasparov, demonstrating AI's potential to outperform human intelligence in specific domains. In 2012, the introduction of deep learning—a subset of machine learning involving neural networks with many layers—ushered in a new era of AI capabilities. This breakthrough has since underpinned technologies like voice assistants and autonomous vehicles.
The historical trajectory of Data Science and AI underscores a pattern of continuous evolution driven by technological advancements and increased data availability. The convergence of these fields has accelerated innovation, leading to today's powerful AI systems capable of performing complex data analyses far beyond the capabilities of traditional statistical methods. For project managers, understanding this history is crucial for navigating the current landscape and strategically positioning their organizations for future success.
Keep Reading
Key technologies and methodologies in data science and ai
The core of Data Science and AI lies in the technologies and methodologies that enable these fields to drive innovation and solve complex problems. Understanding these elements is essential for project managers who seek to integrate Data Science and AI into their operations effectively.
One of the most critical technologies in this domain is Machine Learning (ML), a branch of AI that focuses on building systems that can learn from and make decisions based on data. ML algorithms are designed to identify patterns and relationships within data, enabling predictive and prescriptive analytics. For example, in e-commerce, recommendation systems use ML to analyze user behavior and suggest products that customers are likely to purchase.
Neural Networks, particularly deep neural networks, are another pivotal technology. These are modeled after the human brain's neural networks and are highly effective at recognizing patterns, making them ideal for complex tasks like image and speech recognition. Deep learning, which involves neural networks with many layers, has been instrumental in achieving significant advancements in AI, such as self-driving cars and real-time language translation.
Big Data Analytics is another cornerstone technology, allowing organizations to process and analyze vast amounts of data from diverse sources. This capability is crucial for extracting valuable insights that drive decision-making. With the proliferation of data generated by the Internet of Things (IoT) devices, social media, and other digital channels, effectively leveraging big data has become a competitive necessity.
The methodologies employed in Data Science and AI are equally important. Supervised learning involves training a model on a labeled dataset, meaning the data comes with the correct answers. This approach is widely used in applications like spam detection and credit scoring. In contrast, unsupervised learning deals with unlabeled data and is used for tasks like clustering and anomaly detection, such as identifying fraudulent transactions.
Data mining is the process of discovering patterns in large datasets, often used for market basket analysis and customer segmentation. Meanwhile, predictive modeling uses statistical techniques to forecast future outcomes based on historical data, playing a crucial role in areas like demand forecasting and risk management.
These technologies and methodologies are continually evolving, driven by advancements in computing power, algorithms, and data availability. As they advance, they offer new capabilities and opportunities for organizations to enhance efficiency, innovate, and gain a competitive edge. For project managers, staying abreast of these developments is crucial for leveraging Data Science and AI to meet their strategic objectives.
Case studies: data science and ai in action
Healthcare Revolution through AI
The healthcare sector has been one of the most significant beneficiaries of the integration of Data Science and AI. These technologies are transforming patient care and diagnostics, resulting in improved outcomes and efficiencies. For example, IBM's Watson Health uses AI to analyze large volumes of medical literature and patient data to assist doctors in diagnosing diseases and developing treatment plans. This AI-driven approach helps reduce diagnostic errors and ensures that patients receive the most effective treatments promptly. Furthermore, AI-powered imaging tools are enhancing radiologists' ability to detect abnormalities in medical scans, such as X-rays and MRIs, with higher precision and speed than traditional methods.
AI in Finance
The finance industry has embraced AI and Data Science to enhance security, personalization, and decision-making. One of the most notable applications is in fraud detection, where AI algorithms analyze transaction patterns to identify suspicious activities and prevent fraudulent transactions in real time. For instance, JPMorgan Chase uses AI to monitor millions of transactions daily, identifying anomalies that could indicate fraud. Additionally, AI is revolutionizing personalized banking by analyzing customer data to offer tailored financial advice and products, improving customer satisfaction and loyalty. Robo-advisors, which leverage AI to provide automated investment advice, are another example of how AI is making financial services more accessible and efficient.
Manufacturing Optimization
In manufacturing, Data Science and AI are driving significant improvements in production efficiency and quality control. Predictive maintenance, enabled by AI, allows manufacturers to monitor equipment in real-time, predict failures before they occur, and schedule maintenance activities proactively. This approach reduces downtime and maintenance costs while extending the lifespan of machinery. For example, General Electric (GE) uses AI-powered analytics to predict when parts of their jet engines will need replacement, optimizing maintenance schedules and minimizing disruptions. AI is also enhancing quality control by analyzing product data to identify defects and ensuring that only high-quality products reach the market.
The tangible outcomes of these implementations include cost savings, increased operational efficiency, and enhanced customer experiences. As these case studies illustrate, the integration of Data Science and AI into various sectors is not only revolutionizing operations but also providing a blueprint for future innovations. For project managers, understanding these applications and the benefits they bring is crucial for identifying opportunities to leverage these technologies in their projects.
Keep Reading
Challenges and limitations of data science and ai
Despite their transformative potential, the deployment of Data Science and AI technologies is not without its challenges and limitations. Understanding these hurdles is crucial for project managers seeking to implement these technologies effectively and ethically.
One of the most common challenges in deploying AI and Data Science is technical complexity. Developing and implementing AI models requires a high level of expertise in data science, machine learning, and software engineering. Organizations often face difficulties finding skilled professionals and building the necessary infrastructure to support AI initiatives. Additionally, the deployment of AI systems can be resource-intensive, requiring significant investments in technology and talent.
Ethical and operational challenges also pose significant hurdles. Data privacy concerns are paramount, as AI systems often rely on large volumes of personal data to function effectively. Ensuring compliance with data protection regulations, such as the General Data Protection Regulation (GDPR) in the EU, is a critical consideration for organizations. Moreover, AI systems can inadvertently perpetuate biases present in the data they are trained on, leading to unfair or discriminatory outcomes. Addressing these ethical concerns is essential to maintaining public trust and ensuring the responsible use of AI.
Another limitation of current AI capabilities is their reliance on data quality and quantity. AI models require vast amounts of high-quality data to function accurately, and poor data quality can result in unreliable or biased predictions. Furthermore, AI systems often struggle with tasks that require understanding context or dealing with ambiguous situations, as they lack the nuanced comprehension of human cognition.
Operational challenges include the integration of AI systems into existing workflows and the need for ongoing monitoring and maintenance. AI models are not static; they require continuous updates and optimization to remain effective as new data and circumstances arise. Organizations must establish robust processes for monitoring AI performance and addressing any issues that arise.
By acknowledging these challenges and limitations, project managers can develop strategies to mitigate risks and ensure the successful implementation of Data Science and AI technologies. This involves investing in talent development, prioritizing data quality and ethics, and establishing strong governance frameworks to oversee AI initiatives.
Future of data science and ai
The future of Data Science and AI is bright, with a host of emerging trends and technologies set to shape the landscape in the coming years. For project managers and organizations alike, staying informed of these developments is essential for leveraging these technologies to their fullest potential.
One of the most promising trends on the horizon is the rise of quantum computing. This technology has the potential to revolutionize AI by offering unprecedented processing power, enabling the handling of complex calculations and large datasets at speeds previously unimaginable. Quantum computing could significantly accelerate AI research and development, leading to breakthroughs in areas like drug discovery, climate modeling, and cybersecurity.
Another key trend is the increasing automation of tasks through AI-driven solutions. As AI systems become more sophisticated, they are expected to take on a broader range of tasks, from customer service and logistics to decision-making and strategic planning. This shift towards AI-driven automation has the potential to enhance productivity, reduce costs, and allow human workers to focus on more complex and creative tasks.
Advanced analytics, powered by AI, is also poised to play a significant role in the future. The ability to analyze and interpret data in real-time will provide organizations with deeper insights and enable more proactive decision-making. This capability will be invaluable in sectors like healthcare, finance, and manufacturing, where timely and data-driven decisions can have a significant impact on outcomes.
As Data Science and AI continue to evolve, their trajectory is likely to lead to new applications and innovations. Potential future applications include AI-driven personalization in education, where adaptive learning systems tailor educational content to individual students' needs and learning styles. In the realm of smart cities, AI could optimize traffic management, energy consumption, and public safety, creating more efficient and sustainable urban environments.
For project managers, understanding these trends and potential applications is crucial for positioning their organizations to capitalize on the opportunities that Data Science and AI present. By embracing these technologies and fostering a culture of innovation, organizations can drive growth, enhance competitiveness, and prepare for the future.
Keep Reading
Economic and social impact of data science and ai
The economic and social impact of Data Science and AI is profound, reshaping industries and society in ways that were once the realm of science fiction. Understanding these impacts is essential for project managers and professionals seeking to navigate this rapidly evolving landscape.
Economically, Data Science and AI offer numerous benefits, including enhanced productivity, cost savings, and the creation of new business models. By automating routine tasks and optimizing processes, these technologies can significantly boost efficiency and reduce operational costs. For example, AI-powered chatbots and virtual assistants are streamlining customer service operations, reducing the need for human intervention and lowering costs. Additionally, AI-driven analytics enable more informed decision-making, allowing organizations to identify opportunities for growth and innovation.
The potential for job creation and displacement is a significant consideration in the economic impact of Data Science and AI. While these technologies have the potential to automate certain tasks, they also create demand for new skills and roles, such as data scientists, AI engineers, and machine learning specialists. As a result, there is a growing need for reskilling and upskilling programs to prepare the workforce for the changing job landscape. Organizations that invest in training and development initiatives can better position themselves to leverage the opportunities presented by Data Science and AI.
Socially, the impact of Data Science and AI extends beyond the economic sphere. These technologies have the potential to transform healthcare, education, and public services, improving quality of life and increasing access to essential services. For instance, AI-powered diagnostic tools are enhancing the accuracy and speed of disease detection, leading to better patient outcomes and more efficient healthcare delivery. In education, adaptive learning systems are providing personalized learning experiences, helping students achieve their full potential.
However, the social implications of Data Science and AI also raise important ethical and societal considerations. Public perception of these technologies is shaped by concerns over privacy, bias, and job displacement. Addressing these concerns is crucial for maintaining public trust and ensuring the responsible deployment of AI solutions. Organizations must prioritize transparency, accountability, and ethical considerations in their AI initiatives to foster trust and acceptance among stakeholders.
By understanding the economic and social impact of Data Science and AI, project managers can better anticipate the challenges and opportunities these technologies present. By embracing innovation and fostering a culture of responsibility and ethics, organizations can harness the transformative power of Data Science and AI to drive positive change.
Regulatory and ethical considerations of data science and ai
As Data Science and AI continue to permeate various aspects of society and industry, the need for robust regulatory and ethical frameworks has become increasingly apparent. Navigating these considerations is essential for project managers and organizations committed to deploying AI responsibly and maintaining public trust.
The current regulatory landscape for Data Science and AI is complex and varies across regions and industries. In the European Union, the General Data Protection Regulation (GDPR) sets stringent requirements for data privacy and protection, impacting how organizations collect, process, and store personal data. Similar regulations are emerging globally, emphasizing the need for organizations to prioritize data privacy and security in their AI initiatives. Compliance with these regulations is essential to avoid legal repercussions and maintain customer trust.
Ethical dilemmas are a significant consideration in the deployment of AI technologies. One of the primary ethical concerns is the balance between innovation and privacy. AI systems often rely on large volumes of personal data to function effectively, raising concerns about data privacy and the potential for misuse. Organizations must implement robust data governance frameworks to ensure responsible data handling and protect individuals' privacy rights.
Bias and fairness are additional ethical concerns in AI deployment. AI systems can inadvertently perpetuate biases present in the data they are trained on, leading to unfair or discriminatory outcomes. Addressing these biases requires organizations to prioritize diversity and inclusivity in their data collection and model training processes. Additionally, organizations should implement fairness audits and monitoring mechanisms to identify and mitigate biases in AI systems.
Transparency and accountability are also critical ethical considerations. Organizations must ensure that their AI systems are transparent and explainable, allowing stakeholders to understand how decisions are made. This transparency fosters trust and enables stakeholders to hold organizations accountable for their AI initiatives. Implementing mechanisms for oversight and accountability, such as ethics committees and independent audits, can further enhance trust and ensure responsible AI deployment.
By prioritizing regulatory compliance and ethical considerations, project managers can navigate the complexities of Data Science and AI deployment effectively. This involves fostering a culture of responsibility and ethics within organizations and prioritizing transparency, fairness, and accountability in AI initiatives. By doing so, organizations can harness the transformative power of Data Science and AI while maintaining public trust and ensuring sustainable and responsible innovation.
Keep Reading
Tips for success: do's and don'ts
Do's | Don'ts |
---|---|
Start with a clear problem definition | Avoid jumping into AI without a plan |
Invest in quality data infrastructure | Neglect data quality and integrity |
Continuously monitor AI models | Assume AI models are set-and-forget |
Engage stakeholders early | Ignore ethical implications |
Stay updated with industry trends | Resist change and innovation |
Conclusion
Throughout this comprehensive guide, we've explored the profound impact of Data Science and AI across various sectors, illustrating their potential to drive innovation and transform industries. From understanding their historical evolution to dissecting key technologies and methodologies, the insights provided here serve as a roadmap for project managers and professionals navigating this complex landscape. The economic, social, and ethical implications of these technologies underscore the importance of responsible and strategic deployment. As we look to the future, the potential of Data Science and AI to revolutionize industries remains vast, with emerging trends like quantum computing and AI-driven automation paving the way for new applications and opportunities. For professionals and organizations ready to embrace these technologies, the strategic advantage is clear: Data Science and AI are not just tools but vital components of a forward-thinking approach to business and innovation. By staying informed, addressing challenges proactively, and fostering a culture of responsibility, project managers can harness the full potential of Data Science and AI to drive positive change and achieve their strategic goals.
Build powerful workflows with Meegle for free!
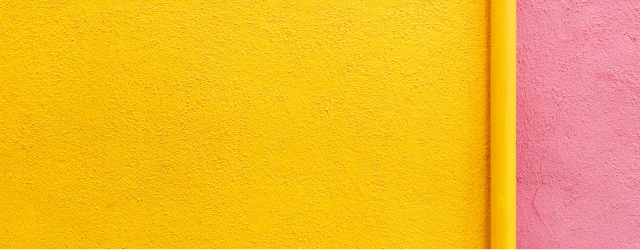
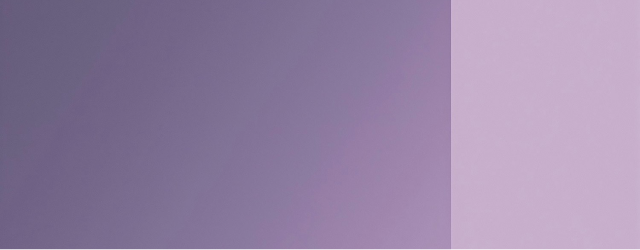
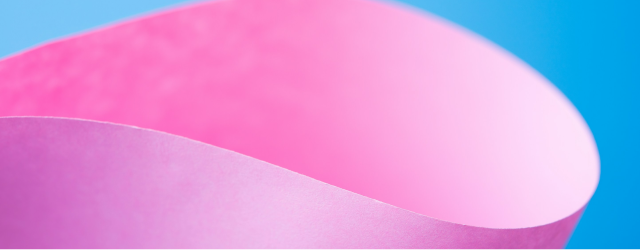
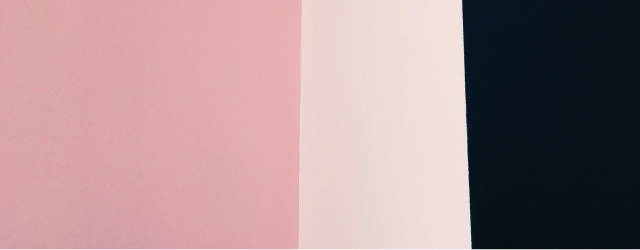
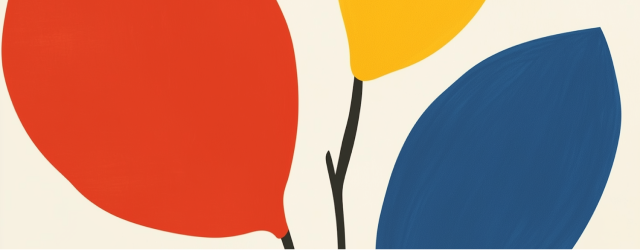
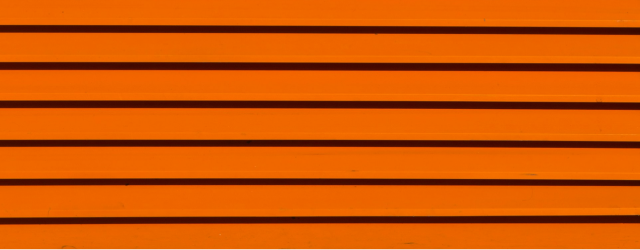
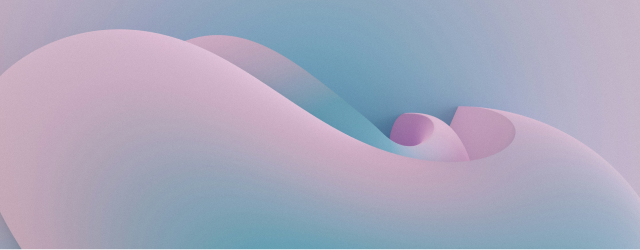
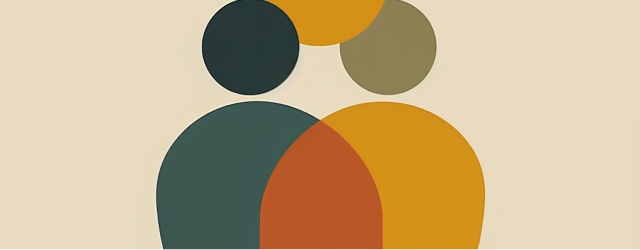