Artificial Intelligence In Battery Health Monitoring
Explore the critical role of Artificial Intelligence in Battery Health Monitoring in electric vehicle manufacturing, offering insights into technological impacts, industry challenges, and future trends.
The rise of electric vehicles (EVs) is reshaping the transportation landscape, offering a cleaner, more sustainable alternative to traditional internal combustion engines. As governments worldwide push for greener initiatives and consumers become increasingly eco-conscious, the demand for EVs is soaring. However, the backbone of this revolution lies in the batteries powering these vehicles. The health and efficiency of these batteries are crucial for the performance, range, and longevity of EVs. A battery's life can significantly impact the overall cost of ownership and the environmental footprint of an electric vehicle. In this context, Artificial Intelligence (AI) emerges as a transformative force in battery health monitoring. By providing real-time insights and predictive analytics, AI not only enhances battery management but also paves the way for innovations in EV manufacturing. This article delves into the integration of AI in battery health monitoring, exploring its potential to revolutionize the electric vehicle industry.
All You Need for Project Management is in Meegle!
Understanding artificial intelligence in battery health monitoring
Defining AI in the Context of EV Battery Management
In the realm of battery health monitoring, Artificial Intelligence refers to the suite of advanced technologies that enable machines to simulate human intelligence processes. This includes learning, reasoning, and self-correction. Within EVs, AI is employed to continuously monitor various parameters of the battery, such as temperature, voltage, and current, to assess its health and performance. By analyzing this data, AI systems can predict potential issues before they occur, ensuring optimal performance and extending the life of the battery.
The importance of battery health in electric vehicles cannot be overstated. Poor battery health can lead to reduced range, increased charging times, and even battery failure, which can be costly to repair or replace. Moreover, the efficiency of an EV is directly tied to its battery health; a well-maintained battery ensures that the vehicle operates at peak performance, maximizing both range and energy efficiency. This is where AI proves indispensable, providing continuous monitoring and real-time insights that are impossible to achieve through manual processes.
AI enhances battery health monitoring by employing predictive analytics and machine learning algorithms to analyze vast amounts of data. These technologies can identify patterns and anomalies that may indicate a potential problem, allowing for proactive maintenance and reducing the risk of unexpected failures. For example, AI can predict when a battery cell is likely to fail based on its usage patterns and historical data, enabling manufacturers to address the issue before it affects the vehicle's performance.
The integration of AI in EV battery management offers significant economic and environmental benefits. Economically, AI-driven battery management can reduce the cost of ownership by extending battery life and minimizing maintenance needs. Environmentally, improved battery health can enhance the sustainability of EVs by reducing the need for battery replacements and minimizing waste. According to a report by McKinsey, the use of AI in battery management can reduce maintenance costs by up to 25% and increase battery lifespan by 30%. These benefits make a compelling case for the broader adoption of AI technologies in the electric vehicle industry.
Historical development
Evolution of AI in Battery Health Monitoring for EVs
The journey of battery health monitoring technologies in the automotive sector has been marked by significant advancements, culminating in the integration of Artificial Intelligence. Initially, battery monitoring systems relied on basic measurements like voltage and temperature, providing limited insights into the battery's condition. As the demand for electric vehicles grew, so did the need for more sophisticated monitoring solutions. This led to the development of advanced battery management systems (BMS) capable of measuring and analyzing a broader range of parameters.
The integration of AI within battery monitoring systems represents a key milestone in this evolution. AI technologies have enabled a shift from reactive to proactive battery management. Instead of relying solely on periodic checks and manual assessments, AI-driven systems continuously analyze real-time data to predict potential issues before they arise. This proactive approach not only enhances battery performance but also extends its lifespan, reducing the need for costly replacements and minimizing downtime.
AI has played a crucial role in the evolution of EV technology and manufacturing processes. By providing deeper insights into battery health, AI has enabled manufacturers to optimize battery design, improve energy efficiency, and enhance overall vehicle performance. For example, companies like Tesla have leveraged AI to develop predictive battery management systems that adjust charging and discharging patterns based on the vehicle's usage, thereby maximizing battery efficiency and longevity.
The shift from traditional to AI-driven battery health monitoring in the EV industry has been transformative. Traditional methods relied heavily on manual inspections and basic diagnostic tools, which were often time-consuming and prone to human error. In contrast, AI-driven systems offer real-time monitoring and predictive capabilities, enabling manufacturers to identify and address potential issues before they impact the vehicle's performance. This shift has not only improved the reliability and efficiency of EVs but also paved the way for innovations in battery design and manufacturing.
Click here to read our expertly curated top picks!
Key technologies
Technological Backbone of AI in Battery Health Monitoring
The integration of AI in battery health monitoring is supported by a range of critical technologies, including machine learning, neural networks, and the Internet of Things (IoT). Machine learning algorithms enable systems to analyze vast amounts of data, identifying patterns and trends that can predict potential issues. Neural networks, a subset of machine learning, are particularly effective in processing complex data sets and making accurate predictions. These technologies are integral to the development of AI-driven battery management systems, providing the foundation for real-time monitoring and predictive analytics.
The integration of AI algorithms in battery management systems involves the use of advanced computational models to analyze data from various sensors embedded within the battery. These sensors collect information on parameters such as temperature, voltage, and current, which is then processed by AI algorithms to assess the battery's health and performance. By continuously analyzing this data, AI systems can detect anomalies and predict potential failures, enabling proactive maintenance and extending the battery's lifespan.
Advancements in sensor technology have also played a crucial role in facilitating precise data collection for AI processing. Modern sensors are capable of providing high-resolution data on various aspects of the battery's condition, allowing AI systems to make more accurate predictions. For example, thermal sensors can detect even slight variations in temperature, which can indicate potential issues such as overheating or thermal runaway. This enhanced data collection capability is essential for the effective functioning of AI-driven battery health monitoring systems.
Cloud computing and big data further enhance AI capabilities in monitoring battery health. Cloud-based platforms provide the computational power needed to process large volumes of data, while big data analytics enable the extraction of meaningful insights from this data. By leveraging these technologies, AI systems can perform complex analyses in real-time, providing manufacturers with actionable insights that can improve battery performance and longevity. The combination of AI, cloud computing, and big data represents a powerful toolset for advancing battery health monitoring and revolutionizing the electric vehicle industry.
Market dynamics
AI's Influence on Battery Health Monitoring and the EV Sector
The impact of AI-driven battery health monitoring on the electric vehicle market is profound, influencing both current trends and future developments. As consumers become more aware of the benefits of efficient battery management, the demand for EVs equipped with advanced AI technologies is on the rise. This trend is fueled by the increasing emphasis on sustainability and the need for reliable, long-lasting battery systems that enhance vehicle performance and reduce maintenance costs.
Consumer demands for efficient and reliable battery management systems are shaping the competitive landscape of the EV market. Manufacturers that adopt AI technologies are better positioned to meet these demands, offering vehicles that deliver superior performance, longer range, and enhanced durability. As a result, companies that invest in AI-driven battery health monitoring are gaining a competitive edge, attracting environmentally conscious consumers who prioritize sustainability and efficiency.
The competitive landscape of EV manufacturers is evolving as more companies recognize the potential of AI technologies. Industry leaders like Tesla, Nissan, and BMW have already integrated AI-driven battery management systems into their vehicles, setting a benchmark for others to follow. These companies are leveraging AI to improve battery performance, extend lifespan, and reduce the overall cost of ownership. As more manufacturers embrace AI, the competition to innovate and develop more advanced battery health monitoring solutions will intensify, driving further advancements in the industry.
AI plays a pivotal role in driving innovation and sustainability in the EV market. By providing real-time insights and predictive analytics, AI enables manufacturers to optimize battery design, improve energy efficiency, and reduce waste. This not only enhances the sustainability of EVs but also contributes to a more efficient and environmentally friendly transportation ecosystem. As the industry continues to evolve, the integration of AI in battery health monitoring will be a key driver of innovation, shaping the future of electric vehicle manufacturing and promoting a more sustainable and efficient transportation network.
Click here to read our expertly curated top picks!
Case studies
Successful Applications of AI in Battery Health Monitoring
The transformative potential of AI in battery health monitoring is best illustrated through the success stories of leading automotive companies. These case studies provide valuable insights into how AI technologies are being leveraged to enhance battery performance, extend lifespan, and improve overall vehicle efficiency.
Tesla's Predictive Battery Management Systems
Tesla is at the forefront of integrating AI into battery health monitoring, utilizing predictive battery management systems that offer real-time monitoring and predictive maintenance. Through machine learning algorithms, Tesla's system continuously analyzes data from the battery, identifying patterns that could indicate potential issues. This proactive approach allows Tesla to optimize battery performance and extend the lifespan of its vehicles. By predicting battery degradation and adjusting charging patterns, Tesla has significantly improved battery longevity and vehicle performance, setting a standard for the industry.
Nissan's Machine Learning Algorithms for Battery Efficiency
Nissan has harnessed machine learning algorithms to enhance battery performance and efficiency. By analyzing data collected from its vehicles, Nissan's AI systems can optimize battery usage, reducing energy consumption and improving range. The implementation of these technologies has resulted in significant cost savings and enhanced energy efficiency, making Nissan a leader in sustainable electric vehicle manufacturing. The use of AI has also enabled Nissan to offer more reliable and efficient vehicles, meeting consumer demands for sustainability and performance.
BMW's AI-Driven Battery Health Diagnostics
BMW has embraced AI-driven battery health diagnostics to deliver advanced diagnostics and anomaly detection in its battery systems. This technology enables BMW to identify potential issues before they impact vehicle performance, ensuring reliability and enhancing customer satisfaction. The use of AI has allowed BMW to offer vehicles that are not only efficient but also highly reliable, strengthening its competitive position in the market. By leveraging AI technologies, BMW continues to innovate and develop cutting-edge battery health monitoring solutions that set new standards for the industry.
These case studies highlight the significant impact of AI in battery health monitoring, demonstrating its potential to revolutionize electric vehicle manufacturing. By providing real-time insights and predictive analytics, AI enables manufacturers to optimize battery performance, extend lifespan, and improve overall vehicle efficiency. As more companies embrace AI technologies, the industry will continue to evolve, driving innovation and promoting a more sustainable and efficient transportation ecosystem.
Challenges and solutions
Navigating Obstacles in AI-Driven Battery Health Monitoring
Despite the promising potential of AI in battery health monitoring, implementing these technologies is not without its challenges. One of the primary obstacles is the complexity of data involved in battery health monitoring. AI systems must analyze vast amounts of data from various sensors, requiring sophisticated algorithms and computational power. Additionally, integrating AI into existing battery management systems can be challenging, particularly for traditional manufacturers accustomed to more conventional approaches.
To overcome these challenges, it's essential to develop standardized protocols that facilitate the seamless integration of AI technologies into battery management systems. Standardization can simplify the process, ensuring compatibility and interoperability between different systems. Moreover, enhancing data security is crucial to protect sensitive information and maintain consumer trust. Implementing robust cybersecurity measures can safeguard data and prevent unauthorized access, ensuring the integrity of AI-driven systems.
Resistance to adopting AI technologies within traditional manufacturing setups is another challenge. This resistance often stems from a lack of understanding or fear of change. To address this issue, manufacturers can implement change management strategies that foster a culture of innovation and adaptability. Training programs can help employees understand the benefits of AI and equip them with the necessary skills to work with these technologies. Engaging stakeholders and encouraging collaboration can also facilitate the transition to AI-driven systems.
Successful case studies provide valuable insights into overcoming these challenges. For example, companies like Tesla and BMW have effectively addressed data complexity and integration issues by investing in advanced AI algorithms and robust data management systems. By prioritizing data security and fostering a culture of innovation, these companies have successfully implemented AI-driven battery health monitoring, setting a benchmark for the industry. By learning from these examples, other manufacturers can navigate the challenges of AI integration and unlock its full potential in battery health monitoring.
Click here to read our expertly curated top picks!
Regulatory and ethical considerations
Navigating Compliance and Ethical Practices in AI Deployment
The deployment of AI in battery health monitoring for electric vehicles is subject to various regulatory and ethical considerations. Compliance with regulatory frameworks governing AI applications in EV manufacturing is essential to ensuring the technology's safe and responsible use. These regulations may cover data privacy, cybersecurity, and the ethical use of AI in decision-making processes. Familiarizing yourself with the relevant regulations and guidelines is crucial to navigating the compliance landscape and avoiding potential legal pitfalls.
Ethical considerations are also paramount in AI-driven battery health monitoring. Data privacy is a significant concern, as AI systems rely on vast amounts of data to function effectively. Ensuring that data is collected, stored, and processed in compliance with data protection regulations is critical to maintaining consumer trust and safeguarding sensitive information. Additionally, the ethical use of AI in decision-making processes should be prioritized, ensuring that AI-driven systems operate transparently and fairly, without bias or discrimination.
The impact of regulations on innovation and technology adoption can be significant. While regulations are necessary to ensure the safe and ethical use of AI, they can also pose challenges to innovation and technology adoption. Striking a balance between regulatory compliance and technological advancement is essential to fostering an environment that encourages innovation while ensuring responsible AI use. Engaging with regulatory bodies and participating in industry discussions can help shape regulations that promote innovation and protect consumer interests.
Industry standards play a crucial role in promoting responsible AI use. These standards provide guidelines for the ethical and transparent deployment of AI technologies, fostering trust and accountability. By adhering to industry standards, manufacturers can ensure that their AI-driven battery health monitoring systems operate responsibly and align with best practices. Participating in industry collaborations and initiatives can further enhance the development and adoption of responsible AI technologies, driving innovation and sustainability in the electric vehicle industry.
Click here to read our expertly curated top picks!
Tips for do's and don'ts in ai-driven battery health monitoring
Do's | Don'ts |
---|---|
Invest in high-quality data collection systems. | Ignore the importance of data security and privacy. |
Continuously update AI algorithms for accuracy. | Overlook the need for regular system evaluations. |
Engage with stakeholders for collaboration. | Neglect training for workforce on AI systems. |
Monitor industry trends and advancements. | Resist adopting new technologies due to inertia. |
Click here to read our expertly curated top picks!
Conclusion
Key Takeaways
The integration of AI in battery health monitoring for electric vehicles holds transformative potential, offering significant economic, environmental, and performance benefits. By providing real-time insights and predictive analytics, AI enables manufacturers to optimize battery performance, extend lifespan, and reduce maintenance costs. These advancements contribute to the overall efficiency and sustainability of electric vehicles, promoting a cleaner and more environmentally friendly transportation ecosystem. As the industry continues to evolve, embracing AI technologies will be crucial for stakeholders seeking to drive innovation and sustainability in EV manufacturing. Ongoing innovations and future prospects for AI in this sector promise a brighter and more sustainable future for electric vehicle production.
All You Need for Project Management is in Meegle!
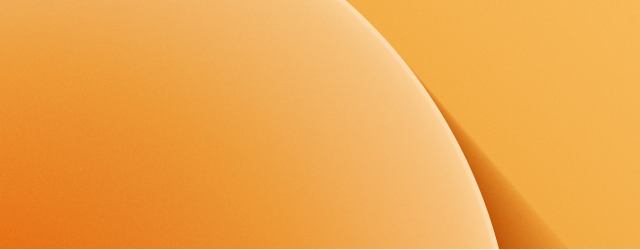
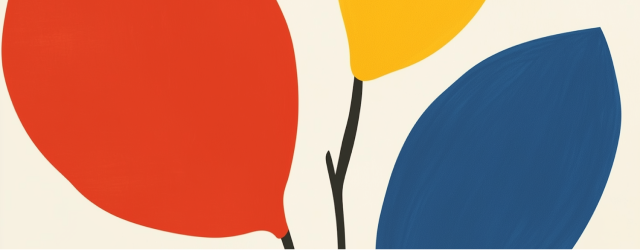
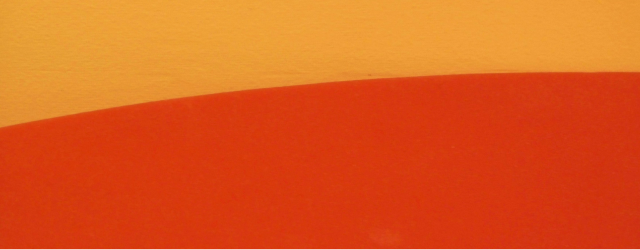
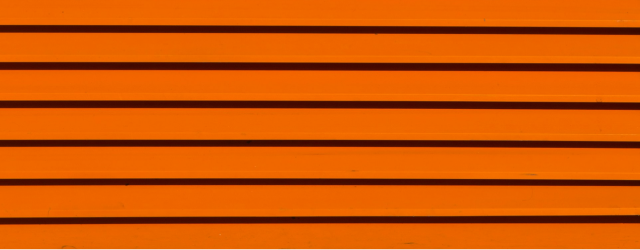
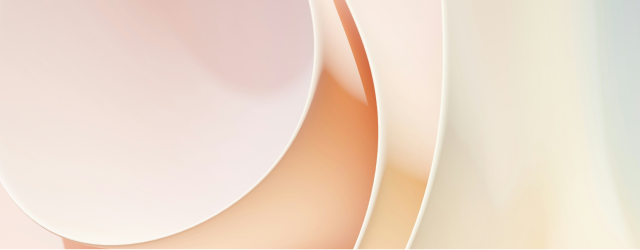
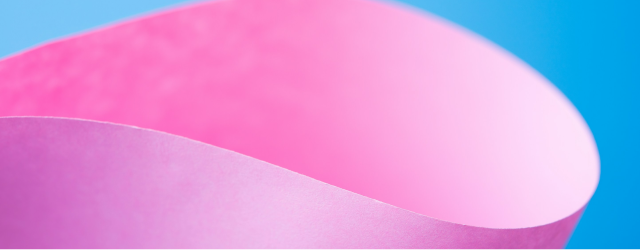
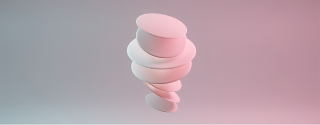