A/B Testing
Enhance your marketing strategies and boost your growth trajectory with actionable insights and practical advice on A/B Testing.
In today's fast-paced digital landscape, A/B Testing has emerged as a cornerstone of effective digital marketing and business growth strategies. Known for its ability to transform data into actionable insights, A/B Testing empowers businesses to make informed decisions that enhance user experience and optimize marketing efforts. The primary aim of this article is to equip professionals with the knowledge and skills needed to harness the full potential of A/B Testing. By delving into its core principles, implementation strategies, and optimization techniques, readers will gain a comprehensive understanding of how to leverage A/B Testing for sustained competitive advantage.
The importance of data-driven decision-making cannot be overstated in today's market. As digital ecosystems become increasingly competitive, businesses that utilize A/B Testing effectively can refine their strategic approaches to meet consumer demands more accurately. This article will explore the intricacies of A/B Testing, uncovering its historical significance and modern-day applications. With a focus on practical insights and expert recommendations, this guide will serve as an essential resource for professionals aiming to enhance their decision-making processes and drive business growth through data-driven strategies.
Your workflow your way. Build it today!
Understanding a/b testing
Introduction to A/B Testing
A/B Testing, sometimes referred to as split testing, is a method of comparing two versions of a webpage, email, or other marketing asset to determine which performs better. By randomly assigning visitors or users to one of two groups—Group A (the control group) and Group B (the treatment group)—businesses can measure the impact of changes in real-time. The primary goal of A/B Testing is to optimize key performance indicators such as click-through rates, conversion rates, and user engagement. This method is rooted in experimental design, allowing marketers to make data-backed decisions by isolating and testing individual variables.
The significance of A/B Testing in optimizing marketing strategies and user experience is profound. For instance, by testing different versions of a call-to-action button, a company can determine which color, placement, or phrasing results in higher conversions. This iterative approach enables businesses to continuously refine their strategies based on empirical evidence, paving the way for improved customer satisfaction and revenue growth. Furthermore, A/B Testing is not confined to digital marketing alone; it can also be applied to product development, pricing strategies, and personalized customer interactions, making it an indispensable tool for modern businesses.
Historically, A/B Testing has evolved significantly in the digital age. Its roots can be traced back to the 1920s when it was first introduced in the context of agricultural research. Fast forward to the digital era, and A/B Testing has become a staple for tech giants like Google and Amazon, who leverage it to fine-tune user experiences and enhance product offerings. As the digital landscape continues to evolve, A/B Testing remains at the forefront of innovation, enabling businesses to navigate change and capitalize on emerging opportunities with confidence.
Theoretical framework
The Principles Behind A/B Testing
At its core, A/B Testing is underpinned by statistical theories that ensure the reliability and validity of its outcomes. The process begins with a clear understanding of control and treatment groups. The control group serves as the baseline, representing the current or standard version of the asset being tested. In contrast, the treatment group is exposed to the new variation, allowing businesses to observe and measure differences in performance. This setup is crucial for isolating the effects of the tested variable and attributing any observed changes directly to the intervention.
Hypothesis testing is a fundamental aspect of A/B Testing. Before launching an experiment, businesses formulate a hypothesis—a prediction of how the change will impact the desired metric. For example, a hypothesis might state that changing the color of a "Buy Now" button from blue to green will increase click-through rates. The experiment is then designed to test this hypothesis, with significance levels determining the likelihood that observed differences are not due to chance. Typically, a significance level of 0.05 is used, indicating a 95% confidence level in the results.
Despite its widespread adoption, A/B Testing is not without its misconceptions. One common misunderstanding is the notion that A/B Testing can provide definitive answers to complex questions. In reality, A/B Testing offers insights into specific variables, and its results must be interpreted within the context of broader business objectives. Additionally, it's essential to understand that the success of an A/B Test depends on proper execution, including adequate sample sizes and appropriate test durations. Missteps in these areas can lead to unreliable results and misguided decisions.
Click here to read our expertly curated top picks!
Implementation strategies
How to Apply A/B Testing
Implementing A/B Testing effectively requires a systematic approach. The process begins with defining clear objectives—what do you hope to achieve with the test? This could range from increasing conversion rates to improving user engagement. Next, select the variables to test. It's crucial to focus on one change at a time, such as altering a headline or adjusting a webpage layout, to ensure that any observed differences can be attributed to that specific variable.
Designing experiments is the next step. This involves creating two versions of the webpage or asset: the control group (A) and the treatment group (B). Use random assignment to allocate users to each group, ensuring that the sample is representative of your target audience. Tools like Google Optimize, Optimizely, and VWO facilitate this process, offering platforms to set up and manage A/B Tests efficiently.
Interpreting results is arguably the most critical stage of A/B Testing. Once the experiment is complete, analyze the data to determine which version performed better. It's essential to consider statistical significance to ensure that the results are not due to random chance. Use metrics such as conversion rate differences, p-values, and confidence intervals to guide your analysis. Remember, the goal is not just to identify the winning version but to understand why it performed better, enabling you to apply these insights to future campaigns.
Sample size and timeline considerations are pivotal for reliable results. A sample size that's too small or a test that runs for an insufficient duration can lead to inconclusive or misleading outcomes. Utilize online calculators or statistical software to estimate the appropriate sample size based on your desired confidence level and effect size. In general, the larger the sample size, the more reliable the results.
Common Applications of A/B Testing
A/B Testing finds applications across various domains, each with unique opportunities for optimization. In the realm of eCommerce, A/B Testing can be instrumental in enhancing conversion rates by experimenting with product page layouts, checkout processes, and promotional offers. For instance, an online retailer might test two versions of a product page—one with detailed descriptions and another with customer reviews prominently displayed—to determine which version drives more sales.
In the Software as a Service (SaaS) sector, A/B Testing is invaluable for refining product features and enhancing user experiences. By testing different onboarding processes, pricing models, or feature placements, SaaS companies can identify the most effective ways to attract and retain customers. A successful case study involves Dropbox, which used A/B Testing to optimize its signup flow, resulting in a significant increase in user registrations.
Digital marketing campaigns also benefit from A/B Testing, particularly in email marketing, social media advertising, and pay-per-click (PPC) campaigns. By testing different subject lines, ad creatives, or landing page designs, marketers can identify the elements that resonate most with their audience, driving higher engagement and conversion rates.
Beyond these applications, A/B Testing plays a crucial role in optimizing website layouts and user interfaces. By experimenting with different navigation structures, color schemes, and content placements, businesses can enhance user satisfaction and reduce bounce rates. In essence, A/B Testing empowers organizations to make data-driven decisions across various touchpoints, ensuring that every aspect of their digital presence is optimized for success.
Skills and proficiency
Developing Skills Around A/B Testing
To conduct effective A/B Testing, professionals must cultivate a specific set of skills and competencies. Statistical literacy is paramount, as A/B Testing relies heavily on data analysis and interpretation. Understanding concepts such as probability, significance levels, and hypothesis testing allows individuals to design robust experiments and draw meaningful conclusions from the results. Additionally, proficiency in statistical software and tools, such as R or Python, can enhance one's ability to analyze complex datasets efficiently.
Data analysis skills are equally important in A/B Testing. Professionals must be adept at extracting insights from raw data, identifying patterns, and making data-driven recommendations. This requires familiarity with data visualization techniques, which facilitate the communication of findings to stakeholders in a clear and compelling manner. Furthermore, critical thinking is essential for evaluating the implications of A/B Test results and making informed strategic decisions.
To stay updated with industry trends and best practices, professionals should invest in continuous learning. Various online platforms offer courses and certifications in A/B Testing, covering topics such as experimental design, statistical analysis, and optimization strategies. Websites like Coursera, Udacity, and edX provide comprehensive resources for individuals seeking to enhance their skills and knowledge. Additionally, attending industry conferences and joining professional networks can facilitate knowledge sharing and keep professionals abreast of the latest developments in A/B Testing.
Ultimately, the ability to conduct effective A/B Testing is a valuable asset in today's data-driven business environment. By developing the necessary skills and embracing continuous learning, professionals can unlock new opportunities for growth and innovation, positioning themselves as leaders in the realm of data-driven decision-making.
Click here to read our expertly curated top picks!
Optimization tips
Tips for Maximizing A/B Testing Efficacy
Designing and executing successful A/B Tests requires attention to detail and a strategic approach to avoid common pitfalls. One practical tip is to start with a clear hypothesis. A well-defined hypothesis sets the foundation for the experiment and guides the selection of variables to test. It should be specific, measurable, and aligned with broader business objectives. For example, instead of vaguely aiming to "increase sales," a more targeted hypothesis could be "changing the homepage banner text will increase click-through rates by 15%."
Another key consideration is the duration of the test. Running an A/B Test for an appropriate length of time is critical to obtaining reliable results. Tests that are too short may not capture enough data to reach statistical significance, while excessively long tests can lead to fluctuating conditions and compromised results. Use statistical tools to estimate the optimal test duration based on your desired confidence level and expected effect size.
Avoiding pitfalls such as confirmation bias is essential for maintaining the integrity of the experiment. Confirmation bias occurs when experimenters subconsciously favor results that align with their expectations. To mitigate this, approach A/B Testing with an open mind and let the data guide decision-making. Additionally, be wary of false positives—results that appear significant but are due to random chance. Implementing a robust statistical framework and using tools like p-values can help distinguish genuine insights from noise.
Iterating and refining experiments is a strategy that can lead to continuous improvement. A/B Testing is not a one-time endeavor but rather an ongoing process of optimization. After each test, analyze the results and identify areas for further exploration. This iterative approach allows businesses to build on previous successes and adapt to changing market conditions, driving sustained growth and innovation.
Best practices
Proven Strategies Using A/B Testing
Implementing industry best practices in A/B Testing can significantly enhance the effectiveness of experiments and drive successful outcomes. A key best practice is to maintain test integrity by ensuring that experiments are conducted under controlled conditions. This includes using random assignment to allocate users to different groups and minimizing external factors that could influence results. Additionally, it's crucial to document the testing process meticulously, including hypotheses, methodologies, and results, to facilitate transparency and replicability.
Ethical considerations are paramount in A/B Testing, particularly when experiments involve user interactions. Businesses must prioritize user privacy and consent, ensuring that tests comply with data protection regulations such as GDPR or CCPA. Clear communication with users about the nature of the experiment and its potential impact is essential for maintaining trust and credibility.
Integrating A/B Testing into the broader business strategy is another best practice that can drive sustained growth. Rather than treating A/B Testing as a standalone activity, businesses should align experiments with overarching strategic goals. This means prioritizing tests that have the potential to deliver significant business impact and allocating resources accordingly. By embedding A/B Testing into the strategic framework, organizations can foster a culture of data-driven decision-making and continuous improvement.
Successful case studies abound in the realm of A/B Testing, showcasing its potential to drive meaningful change. For instance, an online travel agency used A/B Testing to optimize its booking funnel, resulting in a 25% increase in conversions. By testing different page designs and call-to-action placements, the agency was able to identify the most effective combination for maximizing bookings. Such examples underscore the transformative power of A/B Testing and its ability to unlock new avenues for growth and innovation.
Click here to read our expertly curated top picks!
Measuring impact
Tracking and Analyzing Performance
Measuring the impact of A/B Testing involves tracking key performance indicators (KPIs) and using data to refine future experiments. KPIs such as conversion rates, click-through rates, and bounce rates are commonly used to evaluate the success of A/B Tests. These metrics provide quantifiable insights into user behavior and the effectiveness of different variations. By setting clear benchmarks and comparing results against them, businesses can assess the impact of changes and make informed decisions about next steps.
Tools and software play a critical role in data collection, analysis, and visualization. Platforms like Google Analytics, Mixpanel, and Tableau offer comprehensive solutions for tracking user interactions and visualizing test results. These tools enable businesses to monitor real-time performance, identify trends, and extract actionable insights from complex datasets. By leveraging advanced analytics capabilities, companies can gain a deeper understanding of user behavior and optimize their strategies accordingly.
Using analytics to refine hypotheses is a proactive approach to optimizing future A/B Testing efforts. After analyzing test results, businesses should revisit their hypotheses and consider new variables for testing. This iterative process allows organizations to build on existing knowledge and continually enhance their understanding of customer preferences. By adopting a data-driven mindset, businesses can stay ahead of market trends and maintain a competitive edge in an ever-evolving digital landscape.
In summary, measuring the impact of A/B Testing is a dynamic process that involves tracking performance, utilizing advanced tools, and continuously refining hypotheses. By embracing a data-driven approach and prioritizing analytics, businesses can unlock new opportunities for growth and innovation, ensuring that their strategies remain aligned with customer needs and market demands.
Examples of a/b testing success stories
Example 1: ecommerce website conversion optimization
Example 1: ecommerce website conversion optimization
An eCommerce company faced challenges with its checkout process, resulting in high cart abandonment rates. To address this issue, the company implemented an A/B Testing strategy to identify the most effective changes. By testing different versions of the checkout page, including simplified forms and enhanced security features, the company discovered that a streamlined checkout process with fewer fields led to a 20% reduction in cart abandonment and a 15% increase in completed purchases.
Example 2: email marketing campaign enhancement
Example 2: email marketing campaign enhancement
A leading SaaS company aimed to improve its email marketing campaigns' open and click-through rates. Through A/B Testing, the company experimented with various subject lines, email designs, and call-to-action buttons. The results revealed that personalized subject lines combined with visually appealing email designs significantly increased open rates by 25% and click-through rates by 10%. This insight enabled the company to tailor its email marketing strategy for better engagement and conversions.
Example 3: mobile app user engagement improvement
Example 3: mobile app user engagement improvement
A mobile app developer sought to enhance user engagement and retention for its app. By conducting A/B Tests on different onboarding processes, the developer tested variations in tutorial lengths, interactive elements, and reward systems. The findings indicated that a shorter, more interactive onboarding experience with immediate rewards led to a 30% increase in user engagement and a 20% improvement in retention rates. Armed with this knowledge, the developer optimized the app's onboarding process to enhance user satisfaction and loyalty.
Click here to read our expertly curated top picks!
Do's and don'ts of a/b testing
Do's | Don'ts |
---|---|
Define clear objectives | Overcomplicate test variables |
Use a large enough sample size | Change multiple variables simultaneously |
Test iteratively | Ignore statistical significance |
Analyze data thoroughly | Rush to conclusions |
Click here to read our expertly curated top picks!
Conclusion
In conclusion, A/B Testing is a powerful tool for driving data-informed business decisions and optimizing strategies across various domains. By embracing A/B Testing, professionals can experiment with different variables, refine their approaches, and enhance their understanding of customer preferences. As the digital landscape continues to evolve, A/B Testing will remain an essential component of strategic toolkits, enabling businesses to navigate change and capitalize on emerging opportunities with confidence.
Your workflow your way. Build it today!
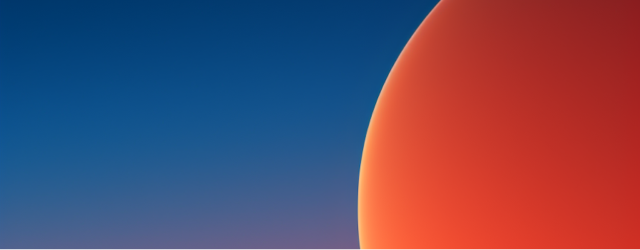
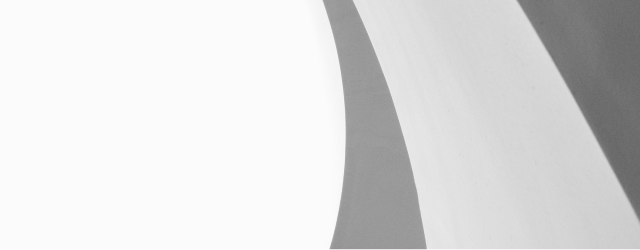
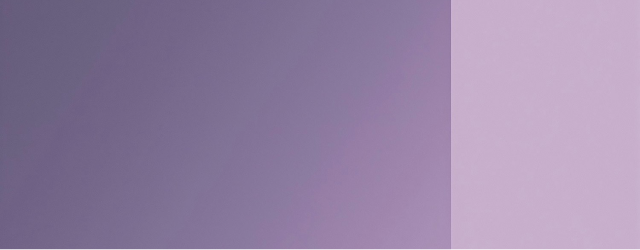
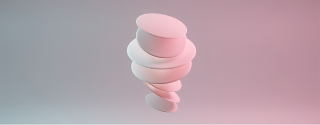
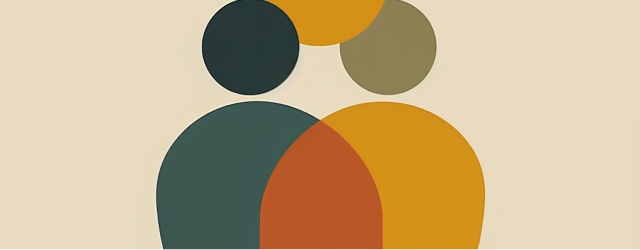
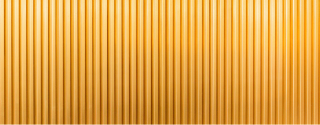
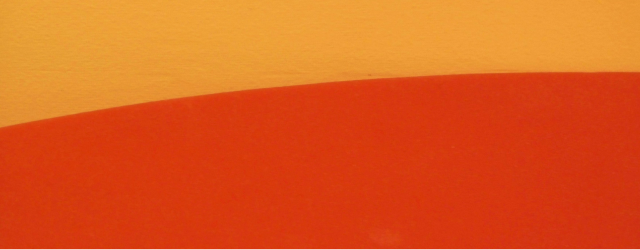
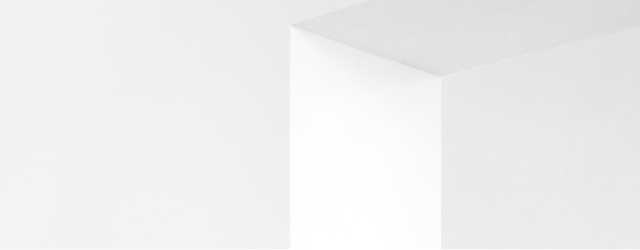