AI In Diagnostics And Treatment Planning
Discover the pivotal role of AI in Diagnostics and Treatment Planning in revolutionizing healthcare practices to enhance efficiency and patient outcomes.
The Rapid Growth of AI Technologies in Healthcare
In recent years, the adoption of AI technologies in healthcare has accelerated rapidly, driven by advances in data science, computational power, and the availability of large datasets. AI's ability to process and analyze vast amounts of medical data with precision offers new opportunities to improve diagnostics and treatment planning. The complexity of data in healthcare – ranging from imaging and genomics to patient history – makes it an ideal candidate for AI solutions, which can uncover patterns and insights beyond human capability.
Specificity of AI Applications in Diagnostics and Treatment Planning
AI's applications in diagnostics include image recognition for radiology, pathology, and dermatology, where AI systems can detect anomalies with remarkable accuracy. For example, AI algorithms are employed in mammography to identify early signs of breast cancer, often outperforming human radiologists in accuracy. In treatment planning, AI helps in developing personalized medicine by analyzing genetic and lifestyle data to suggest individualized treatment protocols. This specificity not only enhances the precision of care but also enables healthcare providers to predict disease trajectories and outcomes more accurately.
Addressing Healthcare Challenges and Improving Patient Outcomes
The integration of AI in healthcare is not just a technological advancement; it is a strategic solution to existing challenges. AI can help mitigate human errors, reduce diagnostic times, and ensure high-quality care across diverse patient populations. By providing predictive analytics, AI can identify at-risk patients and facilitate early interventions, potentially saving lives and reducing healthcare costs. For instance, AI-driven tools in cardiology can predict heart disease risks by analyzing patient data, allowing for timely preventive measures. The ultimate goal of these applications is to improve patient outcomes through more informed decision-making and personalized care.
Experience the next level of project management today!
Core components and functions of ai in healthcare
Understanding AI Algorithms and Models
AI in healthcare primarily relies on different types of algorithms and models, each suited to specific tasks. Machine learning models, a subset of AI, are particularly effective for diagnostics as they learn from vast datasets to recognize patterns and make predictions. For example, supervised learning algorithms are trained on labeled datasets, where the algorithm learns from examples to predict outcomes in new data. Conversely, deep learning models, which use neural networks with many layers, are excellent for image and speech recognition tasks. These models are designed to mimic the human brain's structure and function, allowing them to process complex patterns in medical images.
In diagnostics, AI models analyze medical data to identify patterns that signify particular diseases or conditions. For instance, convolutional neural networks (CNNs), a type of deep learning model, are widely used in radiology to detect anomalies in X-rays and MRIs with precision that rivals or exceeds human expertise. Moreover, AI's role in predictive analytics involves forecasting patient outcomes based on historical data, thereby enhancing decision support for healthcare providers. This capability is crucial in developing early intervention strategies and tailored treatment plans.
Addressing Healthcare Needs with AI
AI addresses critical healthcare needs by enhancing the accuracy and efficiency of diagnostics and treatment planning. One of the primary areas where AI excels is in early diagnosis, where it can detect diseases at stages that are often missed by human observers. For example, AI systems in ophthalmology can screen for diabetic retinopathy by analyzing retinal images, enabling early treatment and preventing vision loss.
In personalized treatment, AI leverages genomics and patient data to develop individualized care plans tailored to a patient's unique genetic makeup and health profile. AI algorithms can analyze a patient's genomic data alongside clinical history to predict how they might respond to different medications, thus optimizing therapeutic outcomes. Moreover, AI reduces human error by providing decision support systems that alert clinicians to potential oversights and inconsistencies in medical records, leading to more accurate and reliable diagnostics.
Technological integration in ai diagnostics and treatment
Enhancing Diagnostics with Advanced Technologies
AI's integration with advanced technologies like the Internet of Things (IoT), cloud computing, and telemedicine is revolutionizing diagnostics. IoT devices collect real-time health data which AI can analyze to provide instant insights and alerts for chronic conditions. For instance, wearable devices that monitor heart rate and blood pressure can feed data into AI systems to detect anomalies, prompting timely medical interventions.
Cloud computing offers scalable resources for storing and processing large medical datasets, enabling sophisticated AI algorithms to function efficiently. AI-enhanced diagnostic tools, such as smart imaging systems, utilize deep learning to provide automated readings of scans, reducing the workload on radiologists and increasing diagnostic accuracy. Such systems can highlight regions of interest in medical images, assisting healthcare professionals in making informed decisions. The combination of AI and telemedicine facilitates remote diagnostics, allowing patients in underserved areas to access expert opinions and timely care.
Innovations and Improvements in Treatment Planning
AI is not only enhancing diagnostics but also driving innovations in treatment planning. By analyzing complex datasets, AI contributes to the development of new treatment protocols and personalized medicine approaches. In oncology, for instance, AI algorithms can analyze a patient's tumor genetics and recommend targeted therapies that are more likely to be effective, minimizing trial-and-error approaches.
AI also plays a pivotal role in therapy optimization by continuously learning from patient responses and suggesting adjustments to treatment plans. In drug discovery, AI accelerates the identification of potential drug candidates by screening vast chemical libraries and predicting drug interactions, significantly reducing the time and cost associated with traditional methods. AI-driven treatment planning systems integrate patient data, clinical guidelines, and real-world evidence to create comprehensive care plans that are personalized, evidence-based, and adaptable to changing patient conditions.
Project management in ai implementation
Effective Practices for AI Project Management
Implementing AI solutions in healthcare requires meticulous project management to ensure success. The critical phases of AI project management include planning, development, testing, deployment, and evaluation. A well-structured approach begins with a thorough needs assessment, identifying specific areas where AI can offer the most value. It's essential to engage stakeholders early in the process, including clinicians, IT professionals, and patients, to gain diverse perspectives and foster buy-in.
Interdisciplinary collaboration is crucial as AI projects often require expertise from various fields, such as data science, medicine, and software engineering. Methodologies like Agile and Lean provide frameworks for managing AI projects, emphasizing iterative development, flexibility, and continuous improvement. Regular feedback loops are vital for refining AI models and ensuring they meet clinical requirements and ethical standards.
Implementing and Monitoring AI Solutions
Successful AI implementation in healthcare follows a step-by-step approach. It begins with defining clear objectives and outcomes, followed by selecting appropriate AI tools and technologies. Once the AI solution is developed, rigorous testing is conducted to validate its accuracy and reliability in real-world settings. Deployment involves integrating the AI system into existing healthcare workflows, ensuring minimal disruption and maximum efficiency.
Monitoring AI system performance is critical to identify potential issues and areas for improvement. Tools and techniques for monitoring include performance metrics, user feedback, and routine audits. Continuous improvement is a cornerstone of AI system management, involving regular updates and refinements to enhance performance and adapt to evolving healthcare needs. Establishing feedback loops ensures that insights from clinical practice inform future AI developments, maintaining alignment with patient care goals.
Real-world examples of ai in diagnostics and treatment
Ai in radiology: revolutionizing imaging
Ai in radiology: revolutionizing imaging
AI is transforming radiology by enhancing imaging accuracy and efficiency. AI tools, such as Aidoc and Zebra Medical Vision, are designed to analyze medical images and detect abnormalities with high precision. These systems use deep learning algorithms to highlight potential areas of concern, assisting radiologists in making accurate diagnoses. For instance, AI can detect early signs of lung cancer in CT scans, often with greater accuracy than human interpretation. By automating routine tasks, AI frees radiologists to focus on complex cases, improving overall diagnostic outcomes.
Precision oncology: ai-powered cancer treatment
Precision oncology: ai-powered cancer treatment
In oncology, AI is driving the development of personalized cancer treatment plans. AI algorithms analyze a patient's genetic data, tumor characteristics, and treatment history to devise targeted therapies with the best chance of success. Companies like IBM Watson for Oncology and Tempus harness AI to provide oncologists with actionable insights, helping tailor treatment options to individual patient profiles. Success stories include AI systems identifying promising treatment combinations for rare cancers, improving survival rates and quality of life for patients.
Ai in cardiology: predictive health monitoring
Ai in cardiology: predictive health monitoring
AI's application in cardiology focuses on predictive health monitoring and early detection of heart conditions. AI-powered tools like HeartFlow and Cleerly use advanced imaging and data analytics to assess cardiovascular risk and plan interventions. These systems can predict heart attacks by analyzing coronary CT angiography images, allowing for proactive treatments. AI also aids in managing chronic heart conditions by continuously monitoring patient data from wearable devices, enabling timely adjustments to treatment plans and reducing hospitalizations.
Challenges and solutions in ai adoption
Navigating Ethical and Regulatory Challenges
The adoption of AI in healthcare is fraught with ethical and regulatory challenges that must be navigated carefully. One of the primary ethical concerns is data privacy, as AI systems require access to vast amounts of patient data to function effectively. Ensuring the confidentiality and security of this data is paramount to maintaining patient trust. Moreover, algorithmic bias poses a risk, where AI systems trained on biased datasets may produce skewed results, leading to disparities in healthcare delivery.
Regulatory challenges include compliance with healthcare standards and obtaining approvals from bodies like the FDA. These challenges necessitate robust validation and transparency in AI system development and deployment. Engaging ethicists, regulators, and diverse stakeholders in the AI deployment process ensures that the systems align with ethical guidelines and legal requirements. Implementing governance frameworks that oversee data usage, algorithm performance, and patient outcomes helps in mitigating ethical risks.
Overcoming Technical and Operational Barriers
Technical and operational barriers often impede AI adoption in healthcare. Common technical challenges include data quality issues, as incomplete or inaccurate data can undermine AI system performance. Integration with existing healthcare infrastructure is another hurdle, where legacy systems may not support modern AI technologies. Overcoming these barriers requires investments in data management practices and the development of interoperable systems.
Training and upskilling staff are crucial for fostering an environment conducive to AI adoption. Healthcare professionals must be equipped with the skills to interpret AI-generated insights and incorporate them into clinical practice effectively. Infrastructure development, including robust IT systems and reliable internet connectivity, supports the seamless integration of AI solutions. By addressing these challenges through strategic planning and resource allocation, healthcare providers can unlock the full potential of AI.
Future prospects and developments in ai
Emerging Trends in AI Technology
The future of AI in healthcare is marked by emerging trends that promise to further enhance diagnostics and treatment planning. Explainable AI is gaining traction as it addresses the "black box" problem of traditional AI systems, providing transparency and interpretability of AI decisions. This trend is particularly important in healthcare, where understanding the rationale behind AI recommendations is crucial for clinical decision-making.
Another significant trend is edge computing, which involves processing data closer to the source rather than relying on centralized cloud servers. This approach reduces latency and enhances data security, making it ideal for real-time health monitoring and diagnostics. As AI systems become more sophisticated, their ability to integrate with augmented reality (AR) and virtual reality (VR) technologies could revolutionize surgical planning and patient education by providing immersive, interactive experiences.
Predictions for AI's Role in Future Healthcare
Looking ahead, AI is poised to play an even more integral role in healthcare delivery. The continued development of AI technologies will likely lead to further advancements in diagnostic accuracy and treatment personalization. AI could enable more proactive healthcare, where predictive analytics and real-time monitoring lead to early interventions and improved patient outcomes. As AI systems become more autonomous, they may take on routine tasks, allowing healthcare professionals to focus on complex decision-making and patient care.
Moreover, AI's role in global health initiatives could expand as it helps address disparities in healthcare access and quality. By facilitating telemedicine and remote diagnostics, AI can extend healthcare services to underserved populations worldwide. Future advancements in AI are expected to be driven by collaborations between technology developers, healthcare providers, and policymakers, ensuring that innovations align with patient needs and ethical standards.
Do's and don'ts in ai implementation
Do's | Don'ts |
---|---|
Engage stakeholders early in the process | Overlook the importance of data privacy |
Ensure continuous monitoring and evaluation | Rely solely on AI without human oversight |
Invest in training and upskilling staff | Ignore ethical considerations and biases |
Foster collaboration between tech and medical teams | Assume one-size-fits-all solutions are effective |
Experience the next level of project management today!
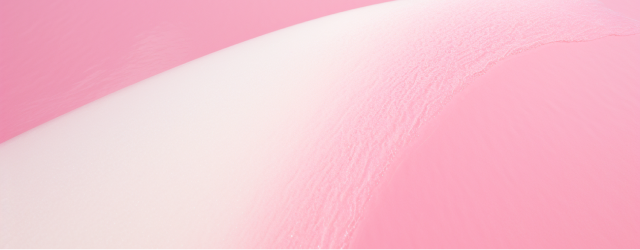
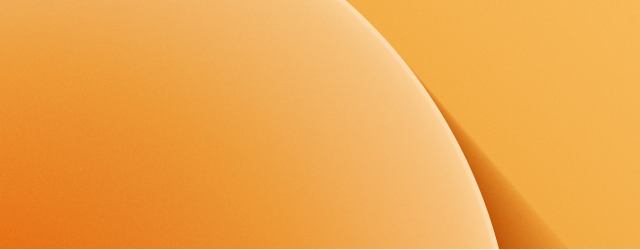
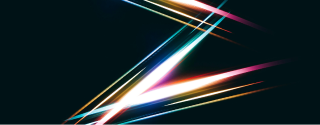
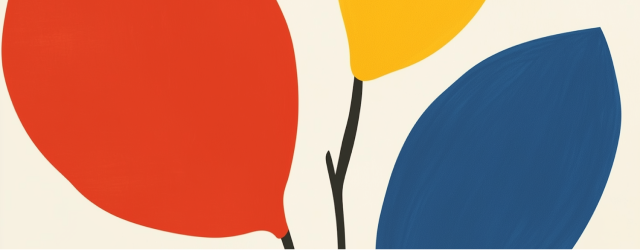
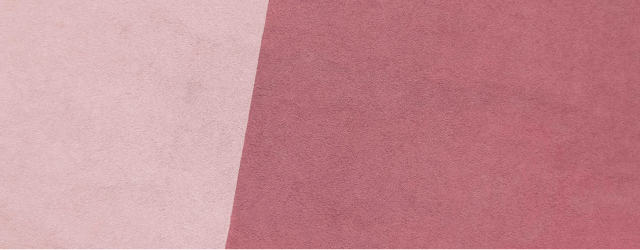
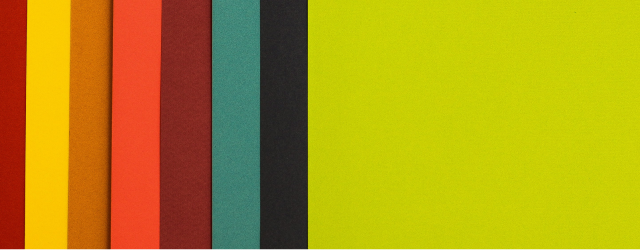
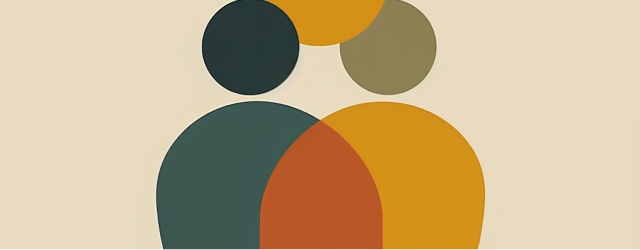
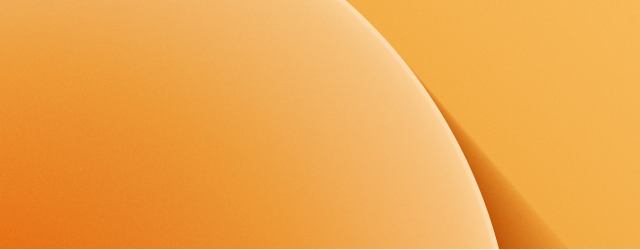