AI And Machine Learning In ITSM
Gain expert insights on AI and Machine Learning in ITSM, including strategic implementations and best practices to streamline your IT service management processes.
Understanding AI and Machine Learning in ITSM
AI and Machine Learning are often used interchangeably but have distinct roles in ITSM. Artificial Intelligence refers to the broader concept of machines being able to carry out tasks in a way that we would consider "smart." In contrast, Machine Learning is a subset of AI that allows systems to automatically learn and improve from experience without being explicitly programmed. Within ITSM, these technologies can automate repetitive tasks, analyze massive datasets, and provide predictive insights that preempt potential issues. For instance, in a large enterprise, AI can automate the ticketing process by categorizing and routing service requests based on historical data and patterns, drastically reducing response times. The scope of AI and ML in ITSM extends beyond mere automation. They play a crucial role in strategic planning, resource allocation, and enhancing customer experiences by offering personalized service delivery. According to Gartner, by 2025, 25% of service operations will use AI to automate tasks, a significant increase from 5% in 2021, highlighting the growing adoption and relevance of these technologies in ITSM.
Objectives of Integrating AI and Machine Learning in ITSM
Integrating AI and ML into ITSM processes aims to achieve several strategic objectives that align with organizational goals. First and foremost, these technologies are implemented to enhance service delivery. This entails improving the responsiveness and accuracy in handling incidents, service requests, and changes. For example, AI-driven chatbots can provide instant support, resolving common queries without human intervention. Another critical objective is optimizing resource management. By leveraging ML algorithms, organizations can predict demand and allocate resources more efficiently, ensuring that the right resources are available at the right time. This predictive capability also plays a pivotal role in reducing operational costs. Minimizing manual interventions and errors leads to substantial cost savings, enabling organizations to invest in other strategic initiatives. Moreover, improving user experience is a key goal. AI-based insights can help tailor interactions based on user behavior and preferences, ensuring a more personalized and satisfying experience. A Forrester study highlights that organizations adopting AI-driven ITSM strategies report a 40% increase in customer satisfaction, underscoring the tangible benefits of these technologies.
Managing IT Services to the Next Level with Meegle
Core principles
Fundamental Concepts Behind AI and Machine Learning in ITSM
At the heart of AI and ML integration in ITSM are several fundamental concepts that drive their effectiveness. Automation and Intelligence are central to these technologies, simplifying complex processes and enabling AI systems to perform tasks that traditionally required human intervention. For instance, automating the incident management process can significantly reduce response times and improve service levels. In addition to automation, data-driven decision-making is a crucial principle. Machine learning algorithms can sift through historical and real-time data to derive actionable insights, enabling organizations to make informed decisions. This capability is particularly beneficial in identifying patterns and predicting future trends, which can preempt potential service disruptions. Furthermore, AI and ML offer scalability and flexibility that are vital for ITSM. These technologies provide scalable solutions that can adapt to varying business needs, whether expanding services or enhancing existing ones. The scalability ensures that as an organization's needs grow, its ITSM capabilities can keep pace without necessitating a complete overhaul of existing systems.
Standards and Best Practices
Implementing AI and ML in ITSM requires adherence to industry standards and best practices to ensure successful deployment and operation. Compliance with industry standards such as ITIL (Information Technology Infrastructure Library) is essential to maintain consistency and alignment with established processes. ITIL provides a framework for ITSM that, when combined with AI and ML, can enhance service delivery and operational efficiency. Equally important are the best practices for deployment of these technologies. Effective deployment starts with stakeholder engagement, ensuring that all parties understand the benefits and potential challenges of AI integration. An iterative development approach is recommended, allowing for continuous refinement and improvement of AI systems based on real-world feedback and changing business needs. Additionally, organizations should focus on building a robust data infrastructure, as the quality and integrity of data significantly impact the effectiveness of AI and ML solutions. By adhering to these standards and best practices, organizations can maximize the benefits of AI and ML in ITSM while mitigating risks associated with implementation.
Click here to read our expertly curated top picks!
Implementation strategies
Planning and Preparations
Successful AI and ML integration into ITSM begins with meticulous planning and preparation. The first step is an assessment of current ITSM capabilities. Organizations must evaluate their existing processes and identify areas where AI can deliver significant value. This assessment involves analyzing current workflows, identifying bottlenecks, and understanding user needs and expectations. Once the current state is understood, the next step is to define clear objectives for the AI and ML initiatives. Establishing measurable goals and key performance indicators (KPIs) is crucial for tracking progress and evaluating the success of implementation. Objectives might include reducing incident response times, improving customer satisfaction scores, or achieving cost savings through automation. Building a skilled team is another critical aspect of preparation. Organizations should assemble a team with expertise in both ITSM and AI technologies, as well as an understanding of business processes and goals. This cross-functional team will be responsible for guiding the implementation, ensuring alignment with business objectives, and fostering a culture of innovation and continuous improvement.
Execution of AI and Machine Learning in ITSM
Step 1: Conduct a Needs Analysis
The execution phase begins with a thorough needs analysis to identify specific areas where AI can add value. This involves engaging stakeholders across the organization to gather insights into pain points and opportunities for improvement. The needs analysis will inform the selection of AI solutions that align with business objectives and priorities.
Step 2: Develop a Roadmap for AI Integration
With the needs identified, the next step is to develop a comprehensive roadmap for AI integration. This roadmap outlines the timelines, resource requirements, and key milestones for implementation. It should also include a risk management plan to address potential challenges and ensure a smooth transition.
Step 3: Pilot AI Solutions
Before full-scale implementation, it is advisable to pilot AI solutions in a controlled environment. Piloting allows organizations to test the feasibility and effectiveness of AI solutions without disrupting existing operations. It also provides an opportunity to gather feedback and refine the solutions based on real-world usage.
Step 4: Gradually Scale Successful Pilots
Upon successful piloting, organizations can gradually scale AI solutions across the enterprise. This involves expanding the scope of AI applications and integrating them into existing ITSM processes. Continuous monitoring and evaluation are essential to ensure that the solutions deliver the expected benefits.
Step 5: Implement Feedback Loops for Continuous Improvement
Finally, implementing feedback loops is crucial for continuous improvement and adaptation. Feedback from users, stakeholders, and performance metrics should be used to refine AI models and processes, ensuring they remain aligned with business objectives and evolving needs.
Practical applications
Scenario-based examples
Scenario-based examples
Example 1: Automated Incident Management
In the realm of ITSM, automating incident management is one of the most impactful applications of AI and ML. By leveraging machine learning algorithms, organizations can automate ticket categorization and routing, significantly expediting incident resolution. For instance, a large financial institution implemented AI-driven automation for incident management and experienced a 40% reduction in average resolution time. The system could accurately categorize incidents based on historical data and route them to the appropriate support teams, minimizing delays and improving service levels.
Example 2: Predictive Maintenance
Predictive maintenance is another compelling use case for AI and ML in ITSM. By analyzing historical data and real-time monitoring, machine learning algorithms can predict potential IT infrastructure failures before they occur. A manufacturing company implemented predictive maintenance strategies using AI and achieved a 30% reduction in unplanned downtime. The system could identify patterns and anomalies in equipment performance, allowing preemptive maintenance actions that minimized disruptions to operations.
Example 3: Enhanced Customer Support
AI-driven chatbots and virtual assistants have become invaluable tools for enhancing customer support in ITSM. These intelligent systems can provide instant support, resolving common queries without human intervention. A telecommunications provider deployed AI-based customer support solutions and saw a 20% increase in user satisfaction scores. The chatbots were able to handle a significant volume of customer inquiries, providing quick and accurate responses that improved the overall customer experience.
Case studies
Case studies
Case Study 1: Company A
Company A, a leading tech firm, leveraged AI-driven automation to transform its incident management process. By implementing AI algorithms to categorize and route service requests, the company achieved a 50% reduction in incident response time. The automation freed up valuable resources, allowing IT teams to focus on more strategic initiatives and improving service delivery.
Case Study 2: Company B
Company B, an enterprise in the manufacturing sector, adopted predictive maintenance strategies powered by AI to reduce operational costs. By analyzing equipment performance data, the AI system could predict potential failures and recommend maintenance actions. As a result, the company achieved a 30% reduction in IT operations costs, demonstrating the financial benefits of AI integration.
Case Study 3: Company C
Company C, a service provider, enhanced its customer support capabilities by deploying AI-based chatbots. The AI solutions were able to handle a significant volume of customer inquiries, providing instant support and resolving common queries. This led to a 20% increase in user satisfaction scores, highlighting the positive impact of AI on customer experience.
Click here to read our expertly curated top picks!
Tools and resources
Recommended Tools for AI and Machine Learning in ITSM
Selecting the right tools and platforms is crucial for successful AI and ML integration in ITSM. Several AI platforms offer robust capabilities for implementing AI solutions in ITSM environments:
- IBM Watson: Known for its natural language processing and machine learning capabilities, IBM Watson is widely used for developing AI-driven ITSM solutions.
- Microsoft Azure AI: With its comprehensive suite of AI services, Azure AI provides tools for building scalable and flexible AI solutions.
- Google AI: Google AI offers a range of machine learning and AI tools that facilitate the integration of AI into ITSM processes.
In addition to AI platforms, machine learning frameworks like TensorFlow and PyTorch are essential for developing custom machine learning models tailored to specific ITSM needs.
Integration Tips with ITSM Platforms
Integrating AI tools with existing ITSM platforms requires careful planning and execution to ensure seamless operation. Here are some strategies for successful integration:
- Seamless Integration: Choose AI tools that offer compatibility with popular ITSM platforms such as ServiceNow, Jira, or BMC Remedy. This ensures a seamless exchange of data and processes between AI systems and ITSM platforms.
- Data Handling and Management: Implement best practices for managing data flows between AI systems and ITSM platforms. This includes ensuring data accuracy, security, and privacy to maintain the integrity of AI-driven insights.
Monitoring and evaluation
Metrics to Monitor AI and Machine Learning in ITSM
Monitoring and evaluation are critical for assessing the performance and effectiveness of AI and ML solutions in ITSM. Key performance metrics to track include:
- Incident resolution time: Measure the average time taken to resolve incidents and compare it with historical data to evaluate improvements.
- User satisfaction scores: Gather feedback from users to assess the impact of AI-driven solutions on customer experience.
- Operational cost savings: Calculate cost savings achieved through automation and predictive maintenance to determine the financial benefits of AI integration.
Ensuring data quality and integrity is also crucial. Accurate and up-to-date data is essential for AI systems to deliver reliable insights and predictions. Regular audits and validation processes should be implemented to maintain data quality.
Continuous Improvement Approaches
Continuous improvement is essential for maximizing the benefits of AI and ML in ITSM. Implementing feedback loops allows organizations to gather insights from users and stakeholders, which can be used to refine AI models and processes. Feedback should be collected regularly and used to make data-driven adjustments that enhance the performance and effectiveness of AI solutions.
Adopting iterative development methodologies, such as agile, enables organizations to iteratively enhance AI capabilities based on changing ITSM needs. This approach fosters innovation and ensures that AI solutions remain aligned with business objectives and evolving requirements.
Related:
Feature Management TemplateClick here to read our expertly curated top picks!
Do's and don'ts
Do's | Don'ts |
---|---|
Ensure stakeholder buy-in and engagement. | Rush the implementation without adequate planning. |
Start with small, manageable AI projects. | Overlook the importance of data quality. |
Continuously monitor and adjust AI models. | Ignore user feedback and insights. |
Prioritize compliance with industry standards. | Deploy AI solutions without thorough testing. |
Related:
Feature Management TemplateClick here to read our expertly curated top picks!
Conclusion
Summarizing Key Points
AI and Machine Learning are transforming IT Service Management by enhancing efficiency, reducing operational costs, and improving user experiences. From automating routine tasks to predicting future service trends, these technologies offer substantial benefits that align with organizational goals. By understanding fundamental concepts, adhering to industry standards, and implementing best practices, organizations can successfully integrate AI and ML into their ITSM processes.
Future Trends
As AI and Machine Learning continue to evolve, several emerging technologies will further revolutionize ITSM. Advancements in natural language processing will enhance AI-driven customer support, enabling more sophisticated and human-like interactions. Additionally, autonomous IT operations will become more prevalent, allowing AI systems to manage and optimize IT environments with minimal human intervention. The ITSM ecosystem will continue to evolve, driven by these technologies, leading to new service models and innovations that redefine IT service delivery. By staying informed and adapting to these changes, organizations can remain competitive and leverage AI and ML to drive business success.
Managing IT Services to the Next Level with Meegle
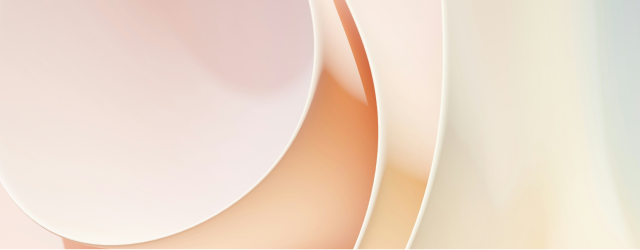
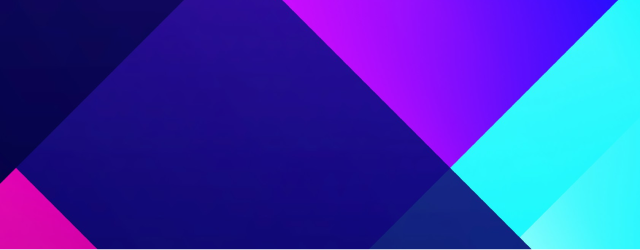
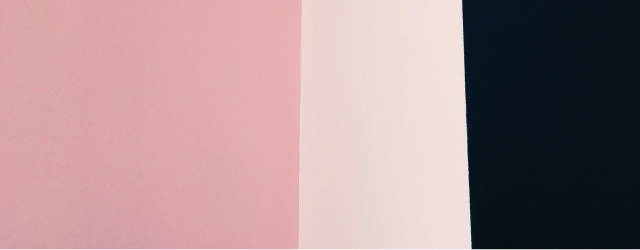
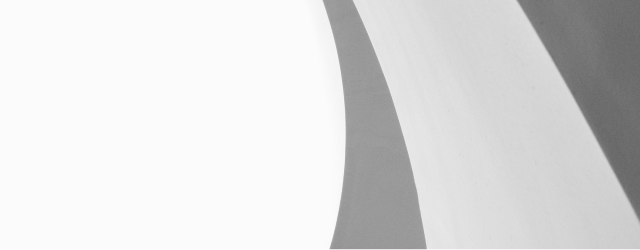
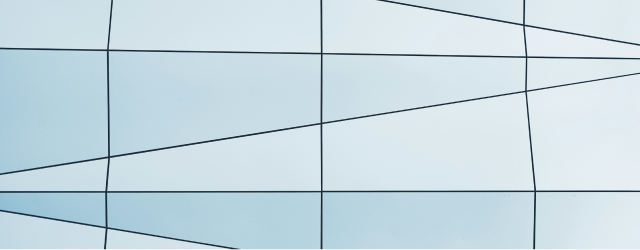
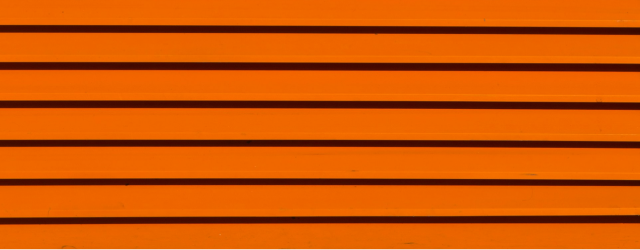
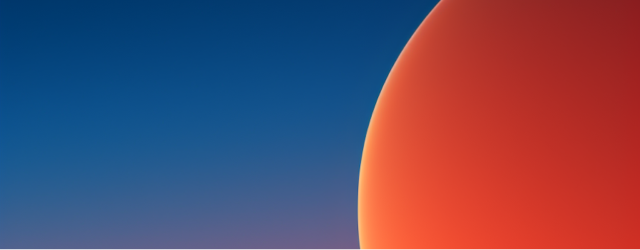
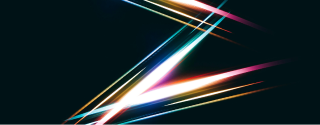