AI-Driven Customer Insights
Gain essential insights on optimizing AI-driven customer insights with our detailed guide, offering strategies for successful Online-to-Offline (O2O) engagement.
In today’s fast-paced digital era, understanding customer behavior has become more crucial than ever for businesses striving to maintain a competitive edge. Customer insights have evolved from being a mere support function to a strategic cornerstone of business transformation. As companies navigate the complexities of the modern marketplace, harnessing accurate and timely insights into customer preferences and behaviors can be the differentiator between success and failure. This shift is largely driven by the burgeoning role of artificial intelligence (AI), which has revolutionized how businesses gather, analyze, and apply customer data.
The advent of AI has introduced sophisticated mechanisms for deriving insights that were previously unimaginable. Particularly in the domain of Online-to-Offline (O2O) strategies—where businesses aim to seamlessly integrate their online and offline operations—AI-driven insights provide a crucial link. These insights enable businesses to create personalized customer experiences, optimize inventory, and enhance operational efficiency. As such, the business landscape is witnessing a paradigm shift where AI is not just an enabler but a necessity for strategic decision-making.
This article aims to serve as a detailed guide for professionals and aspiring project managers seeking to leverage AI-driven customer insights effectively. By exploring the various facets of AI applications, from conceptual frameworks to implementation strategies, we seek to empower readers with the knowledge needed to transform their business operations. Through practical examples, expert opinions, and a problem-solving approach, this guide will illuminate the path to successfully integrating AI-driven insights into O2O strategies and beyond.
Navigate O2O Project Success with Meegle, Today
Overview of ai-driven customer insights
Defining AI-driven Customer Insights in the Digital Age
AI-driven customer insights refer to the process of utilizing artificial intelligence technologies to collect, analyze, and interpret customer data. In the digital age, where data is abundant yet complex, AI provides the tools necessary to make sense of massive datasets, uncover patterns, and predict future behaviors. This capability is essential for businesses looking to gain a deeper understanding of their customers and to create strategies that are both responsive and proactive.
The transition from traditional data analysis to AI-enhanced insights marks a significant shift in how businesses operate. Traditional methods often relied on historical data and manual analysis, which were time-consuming and prone to human error. In contrast, AI-driven insights utilize machine learning algorithms and data mining techniques to provide real-time, accurate, and predictive insights. This transition not only improves efficiency but also enhances the quality and applicability of the insights generated.
In the context of O2O strategies, AI-driven insights play a pivotal role. They enable businesses to seamlessly integrate their online and offline operations by providing a unified view of customer interactions across all channels. This integration is crucial for creating a consistent and personalized customer experience, which is a key factor in driving customer loyalty and business success. For example, AI can analyze online browsing behaviors to predict in-store purchase patterns, allowing businesses to optimize inventory and improve customer satisfaction.
Historically, the use of AI in customer insights has evolved from simple rule-based systems to sophisticated machine learning models. Early efforts focused on automating basic customer service tasks, such as chatbots, but have since expanded to encompass complex data analysis and predictive modeling. Current trends indicate a growing adoption of AI across industries, driven by advancements in computing power, data availability, and algorithmic innovations. As businesses continue to embrace AI-driven customer insights, the potential for enhanced decision-making and competitive advantage becomes increasingly apparent.
Conceptual framework
Core Principles of AI-driven Customer Insights
The foundation of AI-driven customer insights lies in several core principles, including machine learning, data mining, and predictive analytics. Each of these concepts plays a crucial role in transforming raw data into actionable insights, equipping businesses with the tools needed to understand and anticipate customer needs.
Machine learning, a subset of AI, involves algorithms that improve automatically through experience. These algorithms analyze data to identify patterns and make decisions with minimal human intervention. In the context of customer insights, machine learning models can predict customer behaviors, segment customer groups, and offer personalized recommendations. For example, a retail company might use machine learning to analyze purchase histories and suggest products that are likely to interest individual customers.
Data mining is the process of discovering patterns in large datasets. It involves techniques from statistics, artificial intelligence, and database systems. Through data mining, businesses can uncover hidden patterns and correlations in customer data, enabling them to understand trends and customer preferences better. For instance, a restaurant chain might use data mining to determine which menu items are most popular among certain demographics, allowing them to tailor their marketing strategies accordingly.
Predictive analytics extends beyond data mining by forecasting future events based on past data. This approach uses statistical algorithms and machine learning techniques to predict outcomes with a high degree of accuracy. In customer insights, predictive analytics can anticipate customer churn, identify upsell opportunities, and optimize pricing strategies. A telecommunications company, for example, might use predictive analytics to identify customers who are likely to switch to a competitor and offer them targeted retention incentives.
Integrating these principles into an O2O strategy requires careful attention to data quality and algorithmic precision. High-quality data ensures that the insights generated are accurate and reliable, while precise algorithms ensure that predictions are robust and actionable. Businesses must invest in robust data collection and processing systems, as well as continuous model training and validation, to maintain the effectiveness of their AI-driven insights. By adhering to these core principles, businesses can create a seamless and integrated customer experience that bridges the gap between online and offline interactions.
Click here to utilize our expertly curated top project management templates!
Technological drivers
Advanced Tools and Technologies for AI-driven Insights
The landscape of AI-driven customer insights is heavily influenced by technological advancements, with a range of sophisticated tools and platforms driving innovation and efficiency. These technologies are crucial for businesses looking to harness AI's full potential and transform their operations.
One of the primary technologies driving AI-driven insights is AI platforms. These platforms provide the necessary infrastructure for deploying machine learning models and managing large datasets. They offer pre-built algorithms and tools for data processing, allowing businesses to implement AI solutions quickly and efficiently. Leading AI platforms, such as Google Cloud AI and IBM Watson, provide robust frameworks for developing custom AI applications tailored to specific business needs.
Customer Relationship Management (CRM) systems are another essential component in the AI-driven insights ecosystem. Modern CRM systems integrate AI capabilities to analyze customer interactions and extract valuable insights. For example, Salesforce Einstein uses AI to automate data entry, predict sales outcomes, and provide personalized customer experiences. By leveraging AI-enabled CRM systems, businesses can enhance customer relationships and improve overall satisfaction.
Analytics tools, such as Tableau and Power BI, play a critical role in visualizing and interpreting AI-driven insights. These tools allow businesses to create dynamic dashboards and reports that make complex data accessible and actionable. By visualizing insights in a user-friendly format, companies can make informed decisions more quickly and effectively.
Big data and cloud computing are also driving forces behind the scalability and efficiency of AI-driven insights. Big data technologies, like Hadoop and Apache Spark, enable the processing of vast amounts of data in real-time, providing a comprehensive view of customer interactions. Cloud computing platforms, such as Amazon Web Services (AWS) and Microsoft Azure, offer the flexibility and scalability needed to store and analyze large datasets without significant upfront investment.
Real-world applications of these technologies demonstrate their impact on business success. For instance, a global retail chain implemented an AI-driven analytics platform to optimize inventory management. By analyzing sales data and customer preferences, the company reduced stockouts by 30% and increased sales by 15%. Similarly, a financial institution used AI-enhanced CRM systems to improve customer segmentation and targeting, resulting in a 20% increase in customer retention rates.
These technological drivers are essential for businesses seeking to leverage AI-driven customer insights effectively. By investing in advanced tools and technologies, companies can enhance their capabilities, streamline operations, and ultimately deliver superior customer experiences.
Implementation strategies
Deploying AI-driven Customer Insights Effectively
Implementing AI-driven customer insights in a business setting requires a methodical approach to ensure successful integration and tangible results. By following a structured process, businesses can harness the power of AI to drive strategic decision-making and enhance customer experiences.
-
Define Objectives and Goals: The first step in deploying AI-driven insights is to clearly define the business objectives and goals. This involves identifying the specific problems or opportunities that AI will address. For example, a retail company might aim to reduce customer churn or optimize inventory management. Clearly defined objectives provide direction and focus for the implementation process.
-
Data Collection and Preparation: High-quality data is the foundation of effective AI-driven insights. Businesses must establish robust data collection processes to gather relevant customer data from various sources, including online interactions, in-store transactions, and social media. Data preparation involves cleaning, normalizing, and integrating data to ensure consistency and accuracy. This step is critical for building reliable machine learning models.
-
Select Appropriate AI Tools: Once data is prepared, businesses must select the appropriate AI tools and platforms to analyze the data. This selection depends on the specific use case and business requirements. For instance, a company focused on predictive analytics might choose platforms that offer advanced machine learning algorithms, while a business prioritizing customer segmentation might opt for tools with strong data mining capabilities.
-
Build and Train AI Models: With the right tools in place, businesses can build and train AI models to generate insights. This involves selecting the appropriate algorithms, setting parameters, and training models using historical data. Continuous model evaluation and refinement are essential to ensure accuracy and relevance. Businesses should also consider incorporating feedback mechanisms to improve model performance over time.
-
Integrate Insights into Business Processes: The final step is to integrate AI-driven insights into existing business processes and decision-making frameworks. This involves aligning insights with strategic objectives and ensuring that relevant stakeholders have access to actionable information. For example, marketing teams might use insights to personalize campaigns, while supply chain managers might adjust inventory levels based on predictive analytics.
By following these steps, businesses can effectively deploy AI-driven customer insights to enhance their operations and achieve strategic goals. Best practices, such as fostering cross-departmental collaboration and maintaining a focus on data quality, further contribute to successful implementation. Ultimately, a well-executed AI strategy empowers businesses to make informed decisions, improve customer experiences, and gain a competitive advantage in the marketplace.
Click here to utilize our expertly curated top project management templates!
Challenges and solutions
Common Pitfalls and How to Overcome Them
Implementing AI-driven customer insights is not without its challenges. Businesses often face a range of obstacles that can hinder successful adoption and integration of AI technologies. By identifying these common pitfalls and understanding how to overcome them, companies can enhance the effectiveness of their AI initiatives.
One of the most prevalent challenges is data privacy and security concerns. As businesses collect and analyze vast amounts of customer data, ensuring data protection becomes paramount. Organizations must comply with data privacy regulations, such as the General Data Protection Regulation (GDPR) in Europe, to avoid legal repercussions and maintain customer trust. Implementing robust data encryption, access controls, and anonymization techniques can help mitigate privacy risks and protect sensitive information.
Integration complexities also pose significant challenges for businesses adopting AI-driven insights. Integrating AI solutions with existing systems and processes can be daunting, particularly for organizations with legacy infrastructure. To address this challenge, businesses should adopt a phased approach, starting with pilot projects and gradually scaling AI initiatives. Leveraging APIs and middleware solutions can also facilitate seamless integration with existing systems, minimizing disruption and ensuring a smooth transition.
Another common pitfall is the lack of skilled personnel and expertise in AI technologies. Implementing AI-driven insights requires specialized knowledge in data science, machine learning, and analytics. To bridge this skills gap, businesses can invest in training programs, hire experienced professionals, or partner with AI solution providers. Building cross-functional teams that combine technical expertise with domain knowledge can enhance the effectiveness of AI initiatives and drive successful outcomes.
Technological barriers, such as limited computing resources and outdated infrastructure, can also impede AI adoption. Cloud computing offers a viable solution to overcome these challenges by providing scalable and cost-effective resources for data storage and processing. By leveraging cloud platforms, businesses can access advanced AI capabilities without significant capital investment, enabling them to scale their AI initiatives efficiently.
To prevent these challenges from derailing AI-driven insights initiatives, businesses should adopt a proactive approach. This includes conducting thorough risk assessments, establishing clear governance frameworks, and fostering a culture of continuous learning and adaptation. By addressing common pitfalls and implementing preventive measures, organizations can unlock the full potential of AI-driven customer insights and drive sustainable business growth.
Impact analysis
Measuring the Success of AI-driven Customer Insights
Evaluating the effectiveness of AI-driven customer insights is crucial for businesses to understand the impact of their AI initiatives and make informed decisions for future improvements. By assessing the success of these insights, companies can optimize their strategies and maximize the value derived from AI technologies.
One of the primary methods for measuring success is through the use of key performance indicators (KPIs) and metrics. These metrics provide quantifiable measures of how well AI-driven insights are meeting business objectives. Common KPI examples include customer satisfaction scores, conversion rates, and customer retention rates. By tracking these metrics over time, businesses can gauge the effectiveness of their AI initiatives and identify areas for improvement.
In addition to traditional metrics, businesses can also leverage advanced analytics to gain deeper insights into the impact of AI-driven customer insights. Techniques such as A/B testing and cohort analysis allow organizations to compare different strategies and understand which AI-driven interventions are most effective. For example, a retail company might conduct A/B testing to determine the impact of personalized product recommendations on sales and customer engagement.
Real-world case studies further illustrate the impact of AI-driven customer insights. For instance, a telecommunications company implemented AI-powered predictive analytics to identify at-risk customers and offer targeted retention incentives. As a result, the company achieved a 25% reduction in customer churn and improved customer satisfaction scores by 15%. Similarly, an e-commerce platform used AI-driven insights to optimize pricing strategies, leading to a 10% increase in revenue and a 20% improvement in customer lifetime value.
To ensure ongoing success, businesses should establish continuous feedback loops and incorporate insights from customer feedback and market trends. Regularly reviewing and refining AI models based on real-world performance data can enhance accuracy and relevance, ensuring that AI-driven insights remain aligned with business objectives.
By adopting a comprehensive approach to impact analysis, businesses can measure the success of AI-driven customer insights effectively. This not only enables organizations to demonstrate the value of their AI initiatives but also empowers them to make data-driven decisions that drive growth and innovation.
Click here to utilize our expertly curated top project management templates!
Future outlook
Trends and Future Developments in AI-driven Customer Insights
The future of AI-driven customer insights holds immense potential for businesses seeking to enhance their strategic capabilities and customer experiences. As AI technologies continue to evolve, several emerging trends and developments are poised to shape the landscape of customer insights in the coming years.
One of the most significant trends is the increasing integration of AI with other advanced technologies, such as the Internet of Things (IoT) and augmented reality (AR). This convergence will enable businesses to gather more comprehensive and real-time data from a wide array of sources, providing richer insights into customer behaviors and preferences. For example, IoT devices can collect data on customer interactions with physical products, while AR can enhance customer engagement by providing immersive shopping experiences.
Another key development is the growing emphasis on ethical AI and responsible data use. As AI-driven insights become more prevalent, businesses must prioritize transparency, fairness, and accountability in their AI practices. This involves addressing biases in AI models, ensuring data privacy, and fostering trust with customers. Organizations that adopt ethical AI practices will not only mitigate risks but also enhance their brand reputation and customer loyalty.
The rise of explainable AI is also set to transform how businesses interpret and utilize AI-driven insights. Explainable AI provides clear and understandable explanations for AI model decisions, enabling businesses to gain deeper insights into the factors driving customer behaviors. This transparency empowers organizations to make more informed decisions and fosters trust in AI technologies.
For project managers and business strategists, staying abreast of these trends is crucial for navigating the evolving landscape of AI-driven customer insights. Embracing continuous learning, fostering innovation, and building agile teams will be essential for leveraging emerging opportunities and addressing potential challenges.
In conclusion, the future of AI-driven customer insights is characterized by technological advancements, ethical considerations, and enhanced interpretability. By embracing these developments, businesses can unlock new avenues for growth, innovation, and customer engagement, solidifying their position as leaders in the digital age.
Examples of ai-driven customer insights
Example 1: retail industry transformation
Example 1: retail industry transformation
The retail industry has been at the forefront of leveraging AI-driven customer insights to revolutionize customer experience and drive business growth. AI technologies enable retailers to deliver personalized shopping experiences, optimize inventory management, and enhance customer engagement, ultimately transforming how they operate.
One notable application of AI-driven insights in retail is personalized marketing. By analyzing customer data, such as purchase history, browsing behavior, and demographic information, retailers can create tailored marketing campaigns that resonate with individual customers. For example, a clothing retailer might use AI algorithms to recommend outfits based on a customer's past purchases and preferences, increasing the likelihood of conversion and customer satisfaction.
Inventory management is another area where AI-driven insights have had a significant impact. Retailers can use predictive analytics to forecast demand accurately, ensuring that products are available when and where customers want them. By optimizing inventory levels, businesses can reduce costs associated with overstocking and stockouts, improving operational efficiency and profitability. For instance, a grocery store chain might use AI-driven insights to predict which products are likely to see increased demand during certain seasons or events, allowing them to adjust inventory levels accordingly.
Real-world examples demonstrate the transformative power of AI-driven customer insights in retail. A global e-commerce giant implemented AI-powered recommendation systems to personalize product suggestions for customers. As a result, the company reported a 20% increase in revenue from personalized recommendations, highlighting the effectiveness of AI-driven insights in driving sales and customer loyalty.
Overall, AI-driven customer insights have revolutionized the retail industry by enabling businesses to create personalized, efficient, and engaging shopping experiences. As AI technologies continue to evolve, retailers can expect to unlock even more opportunities for innovation and growth, cementing their position in the competitive market landscape.
Example 2: banking and financial services
Example 2: banking and financial services
The banking and financial services industry has embraced AI-driven customer insights to enhance customer segmentation, risk management, and overall customer satisfaction. By leveraging AI technologies, financial institutions can gain deeper insights into customer behaviors, preferences, and needs, enabling them to offer personalized services and improve operational efficiency.
One of the key applications of AI-driven insights in banking is customer segmentation. By analyzing customer data, such as transaction history, credit scores, and demographic information, banks can identify distinct customer segments with varying needs and behaviors. This segmentation allows financial institutions to tailor their products and services to specific customer groups, enhancing customer satisfaction and loyalty. For example, a bank might use AI-driven insights to identify high-net-worth individuals and offer them personalized wealth management services.
Risk management is another critical area where AI-driven insights have made a significant impact. By analyzing historical data and identifying patterns, AI algorithms can predict potential risks and fraudulent activities with high accuracy. This predictive capability enables banks to take proactive measures to mitigate risks and protect customer assets. For instance, a financial institution might use AI-driven insights to detect unusual transaction patterns, flagging potential fraud for further investigation.
Real-world examples highlight the benefits of AI-driven insights in the banking industry. A leading bank implemented AI-powered chatbots to enhance customer service and support. These chatbots provide instant responses to customer queries, reducing wait times and improving customer satisfaction. As a result, the bank reported a 30% increase in customer satisfaction scores and a 15% reduction in operational costs.
Overall, AI-driven customer insights have transformed the banking and financial services industry by enabling institutions to deliver personalized services, improve risk management, and enhance customer experiences. As AI technologies continue to advance, banks can expect to unlock new opportunities for innovation and growth, strengthening their position in the competitive financial landscape.
Example 3: healthcare and patient engagement
Example 3: healthcare and patient engagement
The healthcare industry has witnessed significant advancements in patient care and engagement through AI-driven customer insights. By leveraging AI technologies, healthcare providers can deliver personalized treatment plans, improve operational efficiency, and enhance patient outcomes.
One of the primary applications of AI-driven insights in healthcare is personalized treatment planning. By analyzing patient data, such as medical history, genetic information, and lifestyle factors, AI algorithms can recommend tailored treatment plans that optimize patient outcomes. For example, an AI-driven system might analyze a cancer patient's genetic profile to recommend personalized chemotherapy regimens, increasing the likelihood of treatment success.
Predictive analytics is another area where AI-driven insights have had a profound impact. By analyzing historical data and identifying patterns, healthcare providers can predict patient outcomes and optimize healthcare operations. For instance, AI-driven insights can predict patient readmission rates, allowing hospitals to implement interventions that reduce readmissions and improve patient care.
Real-world examples demonstrate the benefits of AI-driven insights in healthcare. A leading hospital implemented an AI-powered system to analyze patient data and recommend personalized treatment plans for chronic disease management. As a result, the hospital reported a 20% improvement in patient outcomes and a 15% reduction in healthcare costs, highlighting the effectiveness of AI-driven insights in enhancing patient care.
Overall, AI-driven customer insights have transformed the healthcare industry by enabling providers to deliver personalized, efficient, and effective care. As AI technologies continue to evolve, healthcare providers can expect to unlock new opportunities for innovation and improve patient outcomes, solidifying their position as leaders in the healthcare landscape.
Click here to utilize our expertly curated top project management templates!
Tips for do's and don'ts
Do's | Don'ts |
---|---|
Focus on data quality and relevance | Ignore data privacy regulations |
Ensure cross-departmental collaboration | Rely solely on automated insights without human oversight |
Keep scalability and flexibility in mind | Overlook the importance of user training |
Click here to utilize our expertly curated top project management templates!
Conclusion
Recap and Final Thoughts
In conclusion, AI-driven customer insights have emerged as a powerful tool for businesses seeking to enhance their O2O strategies and deliver superior customer experiences. By harnessing the capabilities of AI technologies, companies can gain deeper insights into customer behaviors, optimize operations, and make data-driven decisions that drive growth and innovation.
This comprehensive guide has explored the various facets of AI-driven customer insights, from conceptual frameworks and technological drivers to implementation strategies and impact analysis. By understanding the core principles of AI, businesses can effectively deploy insights to achieve strategic objectives and enhance customer engagement.
The potential long-term benefits of AI-driven insights are immense, offering businesses the opportunity to transform their operations and gain a competitive advantage in the digital landscape. However, successful implementation requires a proactive approach to addressing common challenges, such as data privacy, integration complexities, and technological barriers.
As AI technologies continue to evolve, businesses must embrace continuous learning and adaptation to stay ahead of emerging trends and developments. By fostering innovation and building agile teams, organizations can unlock new avenues for growth and solidify their position as leaders in their respective industries.
Ultimately, AI-driven customer insights offer a pathway to enhanced customer experiences, improved operational efficiency, and sustainable business growth. By leveraging the power of AI, businesses can create personalized, efficient, and engaging interactions that resonate with customers and drive long-term success.
Navigate O2O Project Success with Meegle, Today
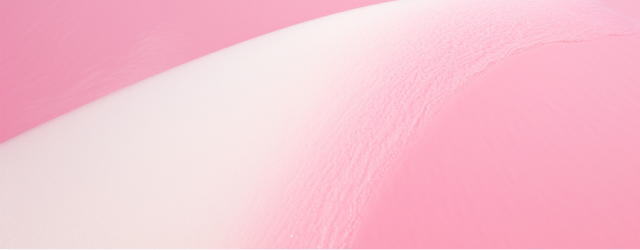
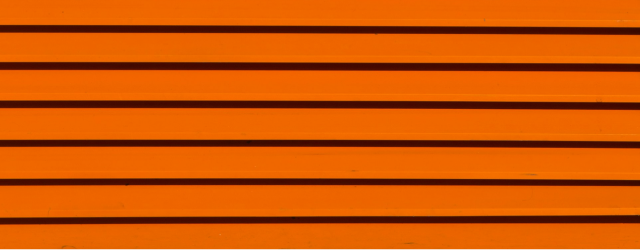
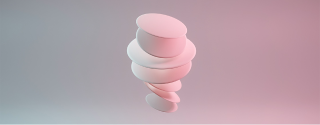
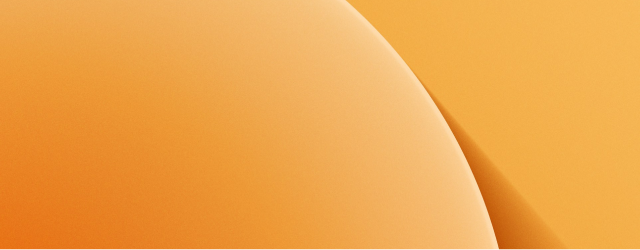
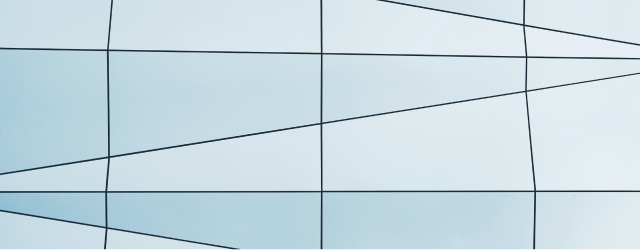
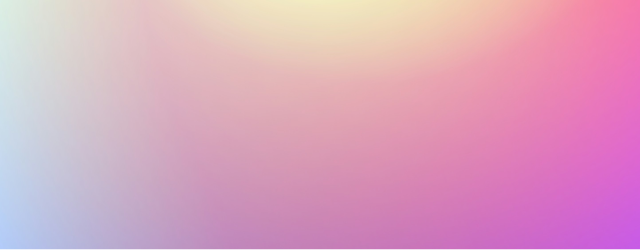
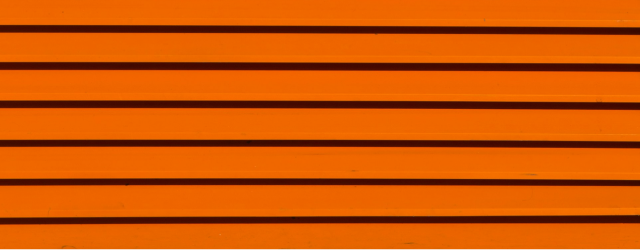
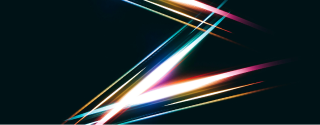