Analytics-Driven Retail Strategies
Gain essential insights on optimizing Analytics-driven retail strategies with our detailed guide, offering strategies for successful Online-to-Offline (O2O) engagement.
Overview of Analytics-driven Retail Strategies
Analytics-driven retail strategies are the backbone of modern retail success, particularly in the context of O2O experiences. At their core, these strategies leverage data analytics to understand consumer behavior, optimize operations, and personalize the shopping journey. In an age where customers expect a seamless experience across both digital and physical touchpoints, the ability to integrate these domains is crucial. The relevance of analytics in this space cannot be overstated. According to a report by McKinsey, retailers that extensively use customer analytics see a potential increase in their profitability by 126%.
In the O2O landscape, the role of analytics is to bridge the traditional brick-and-mortar experience with the digital world. This integration allows for a holistic understanding of customer interactions, whether it's through social media engagement, in-store purchases, or online browsing. By analyzing data from these various channels, retailers can create a unified profile of their customers, enabling them to deliver personalized experiences that drive loyalty and increase sales.
Moreover, the importance of analytics-driven strategies is underscored by the rapid changes in consumer expectations. Today’s customers demand more than just products; they seek personalized experiences and value-driven interactions. Analytics empowers retailers to meet these demands by providing insights that inform everything from inventory management to marketing campaigns. For example, by analyzing purchase history and browsing patterns, retailers can anticipate customer needs and tailor promotions accordingly.
In summary, analytics-driven retail strategies are essential for navigating the complexities of the modern retail landscape. They enable retailers to bridge the gap between online and offline experiences, meet evolving consumer expectations, and ultimately, drive business success.
Brief Historical Evolution Relating to O2O Scenarios
The evolution of retail strategies, particularly in the context of O2O, is a fascinating journey marked by technological advancements and shifting consumer behaviors. Historically, retailers operated primarily through brick-and-mortar stores, where customer interactions were limited to face-to-face engagements. This traditional model began to evolve with the advent of the internet, which introduced a new dimension to retail—online shopping.
The early 2000s saw the rise of e-commerce, where retailers started to establish online storefronts to complement their physical locations. This era marked the beginning of the O2O model, albeit in its nascent stage. The integration of online and offline experiences was minimal, often resulting in disjointed customer journeys.
As technology advanced, so did the opportunities for deeper integration. The introduction of sophisticated data analytics tools enabled retailers to collect and analyze vast amounts of customer data from various touchpoints. This era saw the emergence of multichannel retailing, where brands began to recognize the value of creating consistent experiences across all channels.
A significant milestone in this evolution was the widespread adoption of mobile technology. Smartphones became a pivotal tool for consumers, allowing them to research products, compare prices, and make purchases on the go. Retailers responded by optimizing their websites for mobile use and developing apps that further blurred the lines between online and offline shopping.
Recently, the proliferation of big data, artificial intelligence, and machine learning has propelled O2O strategies into a new realm. These technologies enable retailers to predict consumer behavior with unprecedented accuracy, allowing for personalized marketing and improved customer service. Furthermore, innovations like augmented reality (AR) and virtual reality (VR) are enhancing in-store experiences by providing interactive, immersive shopping environments.
In conclusion, the evolution of retail strategies from traditional brick-and-mortar models to sophisticated O2O experiences is a testament to the transformative power of technology. As we continue to advance, the integration of analytics-driven strategies will become even more critical, shaping the future of retail in ways we are only beginning to imagine.
Navigate O2O Project Success with Meegle, Today
Conceptual framework
Core Principles of Analytics-Driven Retail Strategies
At the heart of analytics-driven retail strategies are several core principles that guide the effective use of data in bridging online and offline experiences. Understanding these principles is essential for any retailer looking to harness the full potential of analytics to drive business success.
The first principle is data collection. Successful analytics-driven strategies begin with gathering comprehensive data from all customer interaction points. This includes online behaviors such as website visits and social media interactions, as well as offline activities like in-store purchases and customer service engagements. The goal is to compile a holistic view of the customer, which serves as the foundation for all subsequent analysis and strategy formulation.
Once data is collected, the next crucial step is data analysis. This involves applying advanced analytical techniques to extract meaningful insights from the raw data. Retailers can employ various methods, such as predictive analytics, to forecast future trends, or sentiment analysis to gauge customer satisfaction. The insights derived from this analysis form the basis for data-driven decision-making, allowing retailers to tailor their strategies to meet specific business goals.
The third principle is application, or turning insights into action. This involves using the data-derived insights to implement strategies that enhance the customer experience, improve operational efficiency, and ultimately drive sales. For example, a retailer might use predictive analytics to optimize inventory levels based on anticipated demand, thereby reducing costs and increasing customer satisfaction.
An often-overlooked principle is continuous improvement. The retail landscape is dynamic, with consumer preferences and market conditions constantly evolving. As such, analytics-driven strategies must be flexible and adaptive. Retailers should regularly review and update their strategies based on new data and insights, ensuring they remain relevant and effective in a changing environment.
In summary, the core principles of analytics-driven retail strategies—data collection, analysis, application, and continuous improvement—provide a structured approach to leveraging data for business success. By adhering to these principles, retailers can create cohesive customer experiences that bridge the gap between online and offline platforms, ultimately enhancing both customer satisfaction and business outcomes.
Integrating Online and Offline Experiences
The integration of online and offline retail experiences is a complex yet rewarding endeavor that can significantly enhance customer satisfaction and drive business growth. Successfully harmonizing these environments requires a strategic approach, leveraging data and technology to create seamless, cohesive experiences across all customer touchpoints.
One effective method for integration is the use of omnichannel retailing. Unlike multichannel approaches, which often result in fragmented experiences, omnichannel retailing focuses on providing a unified customer journey. This means ensuring that customers receive consistent information, services, and branding, whether they're shopping online, in-store, or through a mobile app. For instance, a customer should be able to start a purchase on a retailer's website, continue it on their mobile device, and complete it in-store without any disruption.
Technology plays a crucial role in facilitating this integration. Advanced tools such as customer relationship management (CRM) systems and point-of-sale (POS) solutions enable retailers to track customer interactions across all platforms. By consolidating this data into a single view, retailers can gain insights into customer preferences and behaviors, allowing for more personalized and relevant interactions.
Another key aspect of integration is inventory management. By synchronizing inventory data across online and offline channels, retailers can provide accurate stock information to customers, reducing the frustration of finding an item unavailable in-store or online. This real-time inventory visibility also supports initiatives like buy-online-pick-up-in-store (BOPIS), which combines the convenience of online shopping with the immediacy of in-store pickup.
Successful integration is also about enhancing the in-store experience with digital elements. Technologies like augmented reality (AR) can create immersive shopping experiences, while interactive kiosks and digital signage can provide customers with additional product information and personalized recommendations.
Ultimately, the goal of integrating online and offline experiences is to meet the expectations of modern consumers who desire convenience, consistency, and personalization. By adopting a strategic approach and leveraging the right technologies, retailers can create a seamless customer journey that not only satisfies but delights, driving loyalty and long-term success.
Click here to utilize our expertly curated top project management templates!
Technological drivers
Advanced Tools and Technologies
In the realm of analytics-driven retail strategies, advanced technologies are the engines propelling the industry forward. With the rapid evolution of technology, retailers have access to a plethora of tools that enhance customer insights, personalize shopping experiences, and streamline operations.
One of the most transformative technologies in retail today is artificial intelligence (AI). AI-driven solutions can analyze vast amounts of data to uncover patterns and insights that human analysts might miss. For example, AI can power recommendation engines that suggest products to customers based on their browsing and purchase history, thereby increasing the likelihood of conversion. According to Gartner, businesses that deploy AI in their sales processes can expect a 30% increase in conversion rates by 2025.
Machine learning, a subset of AI, is another powerful tool that enables retailers to predict trends and customer behaviors with remarkable accuracy. By training algorithms on historical data, retailers can forecast demand, optimize pricing strategies, and reduce the risk of stockouts. This predictive capability is crucial for maintaining a competitive edge in a fast-paced market.
Big data analytics is also revolutionizing the retail landscape. With the ability to process and analyze large datasets from various sources, retailers can gain a comprehensive understanding of customer preferences and market trends. Tools like Hadoop and Spark allow for real-time data processing, enabling retailers to make informed decisions quickly.
Moreover, the Internet of Things (IoT) is enhancing the integration of online and offline experiences. IoT devices, such as smart shelves and RFID tags, provide real-time data on inventory levels and customer interactions in-store. This information can be used to optimize store layouts, enhance customer service, and improve inventory management.
In conclusion, advanced technologies like AI, machine learning, big data analytics, and IoT are the driving forces behind analytics-driven retail strategies. By embracing these technologies, retailers can enhance their customer insights, personalize shopping experiences, and drive operational efficiency, ultimately achieving greater business success.
Real-World Applications and Case Studies
To truly understand the impact of analytics-driven retail strategies, examining real-world applications and case studies is essential. These examples demonstrate how retailers have successfully implemented these strategies to improve customer experiences and drive business growth.
One notable example is Amazon, which has become a leader in analytics-driven retail. Amazon utilizes advanced data analytics to personalize customer interactions and streamline operations. Their recommendation engine, powered by AI and machine learning, analyzes customer data to suggest products tailored to individual preferences. This personalization has been a significant driver of Amazon's success, with recommendations accounting for 35% of their total sales.
Another case study is that of Walmart, which employs big data analytics to optimize its supply chain and improve inventory management. Walmart's Retail Link system allows suppliers to access sales data, helping them better understand consumer demand and plan production accordingly. By leveraging data analytics, Walmart has achieved a 25% reduction in inventory levels while simultaneously increasing sales.
A smaller-scale example is the furniture retailer IKEA, which uses augmented reality (AR) to enhance the customer experience. IKEA's Place app allows customers to visualize how furniture will look in their homes using AR technology. This innovative approach not only improves the shopping experience but also reduces the likelihood of returns, as customers can make more informed purchasing decisions.
These case studies illustrate the tangible benefits of analytics-driven retail strategies. By leveraging data and technology, retailers can gain valuable insights, optimize operations, and create personalized customer experiences that drive loyalty and growth. The lessons learned from these examples highlight the importance of embracing analytics as a core component of modern retail strategy.
Implementation strategies
Deploying Analytics-Driven Retail Strategies Effectively
Implementing analytics-driven retail strategies requires a structured approach to ensure seamless integration into existing operations. A step-by-step guide can help retailers navigate this process, from data collection to strategy execution.
-
Data Collection: The first step is to gather comprehensive data from all customer interaction points. This includes both online and offline channels, such as website visits, social media interactions, and in-store purchases. Ensuring data quality and consistency is crucial, as accurate insights depend on reliable data.
-
Data Analysis: Once data is collected, the next step is to analyze it to extract meaningful insights. This involves using advanced analytical tools and techniques, such as predictive analytics and machine learning, to uncover patterns and trends. Retailers should engage data scientists and analysts to interpret the data and provide actionable insights.
-
Strategy Development: Based on the insights gained from data analysis, retailers can develop targeted strategies that align with their business goals. This may involve personalizing marketing campaigns, optimizing inventory management, or enhancing customer service. Retailers should prioritize strategies that offer the greatest potential impact and align with their overall business objectives.
-
Execution: The final step is to execute the strategies effectively. This involves integrating the strategies into existing operations, utilizing the appropriate technologies and tools. Retailers should also ensure that staff are trained and equipped to support the new initiatives, fostering a data-driven organizational culture.
By following this roadmap, retailers can deploy analytics-driven strategies effectively, enhancing their ability to meet customer expectations and drive business success.
Seamless Integration into Existing Systems
Integrating analytics-driven strategies into existing retail systems can present challenges, particularly when dealing with legacy infrastructure and data silos. However, with the right approach, these challenges can be overcome, ensuring a smooth transition and successful implementation.
One of the primary challenges is the integration of disparate data sources. Retailers often have data stored in various systems, such as CRM, POS, and e-commerce platforms. To achieve a unified view of customer interactions, these data sources must be integrated. This can be achieved through the use of data integration tools and platforms that facilitate data consolidation and enable real-time data sharing.
Overcoming legacy system limitations is another common challenge. Many retailers rely on outdated systems that may not support advanced analytics or integration with newer technologies. In such cases, retailers should consider upgrading their infrastructure or adopting cloud-based solutions that offer greater flexibility and scalability.
Addressing data silos is also crucial for successful integration. Data silos occur when information is isolated within specific departments or systems, limiting its accessibility and usability. Retailers can break down these silos by adopting a centralized data management approach, ensuring that data is accessible to all relevant stakeholders.
Finally, fostering a data-driven culture within the organization is essential for seamless integration. This involves encouraging collaboration between departments, investing in employee training, and promoting a mindset that values data-driven decision-making.
By addressing these challenges and leveraging the right tools and strategies, retailers can integrate analytics-driven strategies into their existing systems smoothly, enhancing their ability to deliver personalized and cohesive customer experiences.
Click here to utilize our expertly curated top project management templates!
Challenges and solutions
Common Pitfalls and How to Overcome Them
Implementing analytics-driven retail strategies is not without its challenges. Retailers often encounter several common pitfalls that can hinder their efforts and impact the success of their initiatives. Understanding these challenges and how to overcome them is critical for ensuring successful implementation.
One of the most prevalent challenges is data privacy and security concerns. With increasing regulatory requirements, such as the General Data Protection Regulation (GDPR), retailers must ensure that they handle customer data responsibly and comply with all relevant regulations. Failure to do so can result in significant fines and damage to brand reputation. Retailers must implement robust data protection measures, such as encryption and access controls, and ensure that all employees are trained in data privacy best practices.
Technological barriers also pose a significant challenge. Many retailers rely on outdated systems that may not support advanced analytics or integration with newer technologies. Upgrading these systems can be costly and time-consuming, but it is essential for staying competitive. Retailers should explore cloud-based solutions and invest in scalable infrastructure that can accommodate future growth and technological advancements.
Organizational resistance to change is another common pitfall. Employees may be hesitant to adopt new technologies or approaches, fearing disruption to their established workflows. Retailers can address this resistance by fostering a data-driven culture, providing training and support, and demonstrating the value of analytics-driven strategies in enhancing business outcomes.
Finally, data quality and consistency issues can undermine the effectiveness of analytics-driven strategies. Inaccurate or incomplete data can lead to flawed insights and misguided decision-making. Retailers must prioritize data quality, implementing robust data management processes and regularly auditing their data to ensure its accuracy and reliability.
By addressing these common challenges and implementing preventive measures, retailers can overcome potential obstacles and ensure the successful deployment of analytics-driven strategies.
Practical Solutions and Preventive Measures
To effectively implement analytics-driven retail strategies, retailers must adopt practical solutions and preventive measures that address common challenges and mitigate potential risks. By doing so, they can ensure a smooth implementation process and achieve their desired outcomes.
One practical solution for ensuring data privacy and security is to conduct regular audits of data handling practices and systems. Retailers should establish clear data governance policies and procedures, ensuring that customer data is collected, stored, and processed in compliance with all relevant regulations. Additionally, investing in advanced security technologies, such as encryption and multi-factor authentication, can help protect sensitive data from unauthorized access.
To overcome technological barriers, retailers should explore modern, cloud-based solutions that offer greater flexibility and scalability. These solutions can integrate seamlessly with existing systems and support advanced analytics capabilities. Retailers should also collaborate with technology partners and vendors to ensure that their infrastructure is optimized for analytics-driven strategies.
Addressing organizational resistance requires a focus on change management and employee engagement. Retailers should involve employees in the planning and implementation process, providing training and support to help them adapt to new technologies and approaches. Demonstrating the value of analytics-driven strategies through pilot programs and success stories can also help build buy-in and enthusiasm.
Finally, ensuring data quality and consistency is crucial for the success of analytics-driven strategies. Retailers should implement robust data management processes, including data validation, cleansing, and enrichment. By regularly auditing their data and addressing any discrepancies, retailers can ensure that their insights are accurate and reliable.
By implementing these practical solutions and preventive measures, retailers can overcome common challenges and achieve successful deployment of analytics-driven strategies, ultimately enhancing their ability to deliver personalized and cohesive customer experiences.
Impact analysis
Measuring the Success of Analytics-Driven Retail Strategies
Evaluating the effectiveness of analytics-driven retail strategies is crucial for understanding their impact and guiding future initiatives. By employing robust measurement methodologies, retailers can assess the success of their strategies, identify areas for improvement, and drive continuous growth.
One approach to measuring success is through qualitative assessments. These involve gathering feedback from customers and employees to understand their perceptions and experiences. Surveys, interviews, and focus groups can provide valuable insights into how analytics-driven strategies are perceived and how they impact customer satisfaction and employee engagement. Retailers can use this feedback to refine their strategies and enhance their effectiveness.
Quantitative metrics are equally important for assessing success. Retailers should identify key performance indicators (KPIs) that align with their business objectives and track progress over time. Examples of relevant KPIs include sales growth, customer retention rates, and conversion rates. By analyzing these metrics, retailers can determine whether their strategies are delivering the desired outcomes and make data-driven adjustments as needed.
In addition to traditional metrics, retailers should also consider advanced analytics techniques to measure success. For example, cohort analysis can help identify patterns and trends in customer behavior, providing deeper insights into the impact of analytics-driven strategies. A/B testing can also be used to assess the effectiveness of specific initiatives, allowing retailers to compare different approaches and optimize their strategies accordingly.
Ultimately, measuring the success of analytics-driven retail strategies requires a comprehensive approach that combines qualitative and quantitative assessments with advanced analytics techniques. By doing so, retailers can gain a holistic understanding of their strategies' impact and drive continuous improvement, ultimately enhancing their ability to deliver personalized and cohesive customer experiences.
Metrics and KPIs to Track Progress
To effectively measure the success of analytics-driven retail strategies, retailers must identify and track key performance indicators (KPIs) that align with their business objectives. These metrics provide valuable insights into the impact of their strategies and guide data-driven decision-making for continuous improvement.
One of the most critical KPIs is sales growth. Retailers should track overall sales performance and segment it by channel, product category, and customer segment. By analyzing sales data, retailers can assess the effectiveness of their strategies in driving revenue and identify potential areas for optimization.
Customer retention rates are another essential KPI. Retaining existing customers is often more cost-effective than acquiring new ones, making customer retention a vital measure of success. Retailers should track metrics such as repeat purchase rates, loyalty program participation, and churn rates to gauge customer loyalty and satisfaction.
Conversion rates provide valuable insights into the effectiveness of marketing and sales initiatives. Retailers should track conversion rates across different channels and touchpoints, such as website visits, email campaigns, and in-store promotions. By analyzing conversion data, retailers can identify successful tactics and optimize their strategies for better performance.
Customer satisfaction scores, such as Net Promoter Score (NPS) and Customer Satisfaction Score (CSAT), offer qualitative insights into customer perceptions and experiences. These scores can help retailers understand how customers perceive their brand and identify areas for improvement.
Finally, advanced analytics techniques, such as customer lifetime value (CLV) and customer segmentation analysis, provide deeper insights into customer behavior and preferences. By understanding the lifetime value of different customer segments, retailers can prioritize high-value customers and tailor their strategies accordingly.
By tracking these metrics and KPIs, retailers can gain a comprehensive understanding of their strategies' impact, make data-driven adjustments, and drive continuous improvement in their analytics-driven retail initiatives.
Click here to utilize our expertly curated top project management templates!
Future outlook
Trends and Future Developments
The future of analytics-driven retail strategies is shaped by emerging trends and technological advancements that promise to transform the retail landscape. By staying informed about these developments, retailers can identify opportunities for innovation and maintain a competitive edge.
One of the most significant trends is the increasing use of artificial intelligence (AI) and machine learning in retail. As these technologies become more sophisticated, retailers can leverage them to automate processes, enhance customer insights, and deliver personalized experiences at scale. For example, AI-driven chatbots and virtual assistants can provide instant customer support, while machine learning algorithms can optimize pricing strategies and inventory management.
Another emerging trend is the rise of augmented reality (AR) and virtual reality (VR) in retail. These technologies offer immersive shopping experiences that bridge the gap between online and offline interactions. Retailers can use AR and VR to create virtual showrooms, allowing customers to visualize products in their environment before making a purchase. As these technologies become more accessible, they will play a crucial role in enhancing customer engagement and satisfaction.
The growing importance of data privacy and security is also shaping the future of analytics-driven retail strategies. With increasing regulatory requirements and consumer awareness, retailers must prioritize data protection and transparency. Implementing robust data governance frameworks and adopting privacy-enhancing technologies will be essential for maintaining customer trust and compliance.
Finally, the adoption of edge computing is set to revolutionize data processing in retail. By enabling real-time data analysis at the edge of the network, retailers can achieve faster insights and more responsive decision-making. This capability is particularly valuable for optimizing in-store operations and enhancing customer experiences.
In conclusion, the future of analytics-driven retail strategies is defined by advancements in AI, AR/VR, data privacy, and edge computing. By embracing these trends, retailers can unlock new opportunities, drive innovation, and deliver personalized and cohesive customer experiences that meet the evolving expectations of modern consumers.
Emerging Opportunities for Businesses
As the retail landscape continues to evolve, businesses that leverage analytics-driven strategies will find new opportunities to gain a competitive edge and drive growth. By capitalizing on these opportunities, retailers can enhance their customer experiences, optimize operations, and achieve long-term success.
One emerging opportunity is the ability to deliver hyper-personalized experiences. With advances in data analytics and AI, retailers can gain deeper insights into customer preferences and behaviors, allowing for highly targeted marketing and product recommendations. By tailoring experiences to individual customers, retailers can increase engagement, drive loyalty, and boost sales.
Another opportunity lies in optimizing the supply chain through predictive analytics. By analyzing historical data and market trends, retailers can forecast demand more accurately, optimize inventory levels, and reduce costs associated with overstocking or stockouts. This capability can lead to improved operational efficiency and enhanced customer satisfaction.
The rise of omnichannel retailing presents an opportunity for retailers to create seamless and integrated customer journeys. By harmonizing online and offline experiences, retailers can provide consistent branding and services across all channels, meeting the expectations of modern consumers. This approach enhances customer satisfaction and drives loyalty by providing a convenient, cohesive shopping experience.
Emerging technologies like augmented reality (AR) and virtual reality (VR) offer opportunities to create immersive and engaging shopping experiences. Retailers can use these technologies to showcase products in innovative ways, allowing customers to visualize items in their environment and make more informed purchasing decisions. As AR and VR become more accessible, they will play a crucial role in enhancing customer engagement.
Finally, the growing emphasis on sustainability presents an opportunity for retailers to differentiate themselves by adopting eco-friendly practices. By leveraging data analytics to optimize resource use and reduce waste, retailers can demonstrate their commitment to sustainability and appeal to environmentally-conscious consumers.
In summary, the future of analytics-driven retail strategies offers numerous opportunities for businesses to innovate, differentiate, and succeed. By embracing these opportunities, retailers can enhance their customer experiences, optimize operations, and achieve sustainable growth in an increasingly competitive market.
Examples of analytics-driven retail strategies
Example 1: personalization through predictive analytics
Example 1: personalization through predictive analytics
Predictive analytics is a powerful tool that enables retailers to deliver personalized customer experiences by anticipating individual needs and preferences. A compelling example of this is a well-known fashion retailer that leveraged predictive analytics to enhance their personalization efforts. By analyzing customer data, including purchase history, browsing behavior, and demographic information, the retailer developed sophisticated algorithms to predict future buying patterns. This allowed them to tailor their marketing campaigns, product recommendations, and promotions to individual customers, significantly increasing engagement and sales.
The benefits of enhanced personalization are manifold. Customers receive relevant and timely offers, leading to higher conversion rates and increased loyalty. The retailer also saw a reduction in marketing costs, as they were able to target their efforts more effectively. This approach not only improved customer satisfaction but also drove substantial business growth, showcasing the transformative power of predictive analytics in personalization.
Example 2: inventory management optimization
Example 2: inventory management optimization
Effective inventory management is critical for retailers, and analytics-driven strategies can optimize this process by providing data-driven insights. A notable example is a global electronics retailer that implemented an analytics-driven approach to inventory management. By leveraging data from sales, demand forecasts, and market trends, they developed predictive models to optimize stock levels across their network of stores. This allowed them to reduce excess inventory, minimize stockouts, and improve overall operational efficiency.
The results were impressive. The retailer experienced a significant reduction in carrying costs and an increase in inventory turnover. Customer satisfaction also improved, as they were able to fulfill orders more quickly and accurately. This case study highlights how analytics can streamline inventory management, reduce costs, and enhance customer satisfaction, ultimately driving business success.
Example 3: enhancing customer engagement with ai
Example 3: enhancing customer engagement with ai
Artificial intelligence (AI) has revolutionized customer engagement in retail, offering innovative solutions that enhance the shopping experience. A leading example is a major online retailer that utilized AI-powered chatbots to improve customer service and engagement. These chatbots, integrated into the retailer's website and mobile app, provided instant support for customer inquiries, product recommendations, and order tracking.
The impact of AI-powered chatbots was significant. Customers received quick and accurate responses to their queries, leading to higher satisfaction and loyalty. The retailer also benefited from reduced customer service costs, as the chatbots handled a significant portion of routine interactions. This example demonstrates how AI can enhance customer engagement, improve satisfaction, and drive operational efficiency in the retail sector.
Click here to utilize our expertly curated top project management templates!
Tips for do's and don'ts
Do's | Don'ts |
---|---|
Prioritize data quality and accuracy. | Neglect data privacy and security measures. |
Invest in advanced analytics tools. | Rely solely on outdated technologies. |
Foster a data-driven organizational culture. | Ignore employee training and development. |
Continuously monitor and adjust strategies. | Set static goals without room for flexibility. |
Leverage customer feedback for improvements. | Disregard customer input and market trends. |
Click here to utilize our expertly curated top project management templates!
Conclusion
Recap and Final Thoughts
In conclusion, analytics-driven retail strategies are essential for navigating the complexities of the modern retail landscape. By leveraging data and technology, retailers can bridge the gap between online and offline experiences, meet evolving consumer expectations, and ultimately drive business success.
Throughout this guide, we have explored the core principles, technological drivers, implementation strategies, and challenges associated with analytics-driven retail strategies. We have also examined real-world examples and case studies, highlighting the tangible benefits of these strategies in enhancing customer experiences and optimizing operations.
By prioritizing data quality, investing in advanced technologies, and fostering a data-driven organizational culture, retailers can effectively implement analytics-driven strategies and achieve their desired outcomes. Measuring success through qualitative and quantitative assessments, as well as advanced analytics techniques, is crucial for driving continuous improvement and staying ahead of the competition.
Long-term Benefits in the O2O Landscape
The long-term benefits of adopting analytics-driven strategies in the O2O landscape are manifold. Retailers can achieve sustainable competitive advantage by delivering personalized and cohesive customer experiences that drive loyalty and growth. Enhanced operational efficiency, driven by data-driven decision-making, leads to cost savings and improved profitability.
By staying informed about emerging trends and technological advancements, retailers can identify opportunities for innovation and differentiation. Embracing these developments will enable retailers to adapt to changing consumer expectations and maintain a competitive edge in a rapidly evolving market.
In summary, analytics-driven retail strategies offer enduring advantages in the O2O landscape, positioning retailers for long-term success and growth in an increasingly competitive market.
Navigate O2O Project Success with Meegle, Today
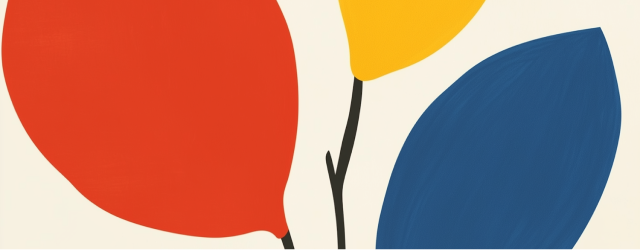
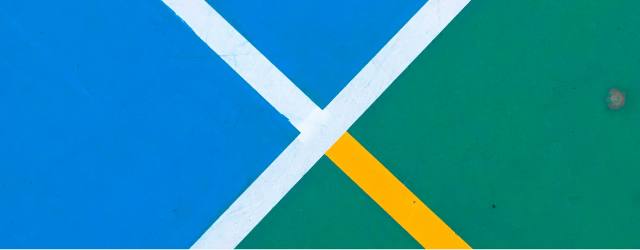
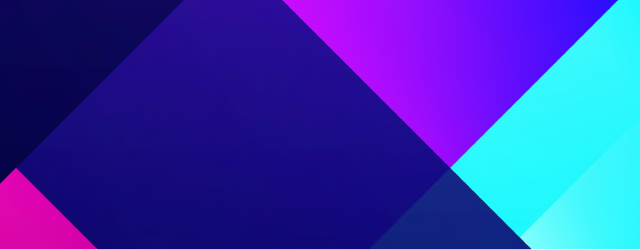
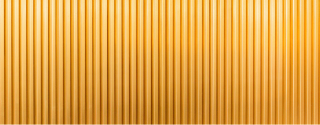
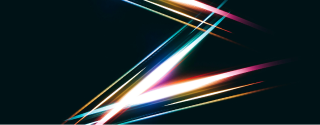
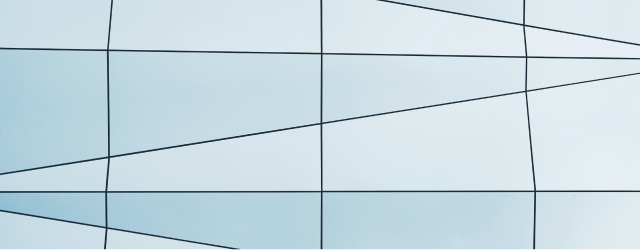
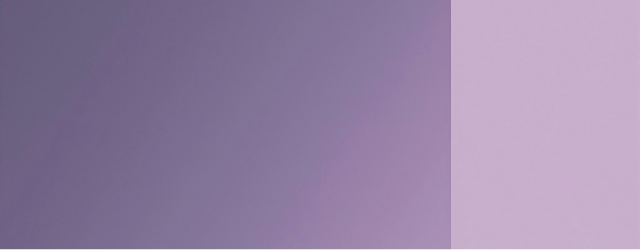
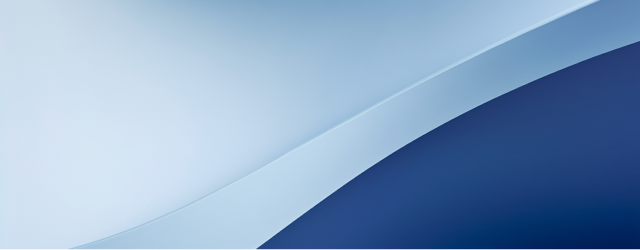