Predictive Analytics
Streamline your supply chain operations with our expert insights on Predictive Analytics, providing you with the know-how to boost efficiency and effectiveness.
Background of Predictive Analytics in Supply Chains
Predictive analytics has emerged as a crucial decision-support tool in the intricate world of supply chain management. Essentially, predictive analytics involves the use of historical data, statistical algorithms, and machine learning techniques to predict future outcomes. In the context of supply chains, these predictions can pertain to demand forecasting, inventory management, risk analysis, and numerous other facets that require foresight for optimal decision-making. By leveraging historical data, businesses can uncover patterns and trends that inform strategic planning and operational adjustments.
The integration of predictive analytics within supply chains represents a fusion of data science and supply chain expertise. For instance, a retailer might use predictive analytics to anticipate holiday shopping spikes, ensuring stock levels are sufficient to meet consumer demand without overstocking. This approach not only minimizes waste but also maximizes sales opportunities. Similarly, manufacturers may predict equipment failures and schedule maintenance proactively, thus minimizing downtime and maintaining productivity.
The application of machine learning within predictive analytics further enhances its efficacy. Machine learning algorithms can continuously learn and adapt to new data inputs, refining predictions over time. This capability is particularly valuable in volatile market conditions where past trends may not fully predict future occurrences. As such, predictive analytics has become indispensable for modern supply chains, offering a robust foundation for agile and informed decision-making.
Importance in Today’s Market
In the current economic and technological climate, the importance of predictive analytics in supply chains is underscored by several key factors. Market volatility, driven by globalization and rapid technological advancements, presents a constant challenge for businesses striving to remain competitive. Customer expectations have evolved significantly, with consumers demanding faster delivery times, personalized service, and greater transparency. As such, companies are compelled to enhance their operational efficiency and responsiveness.
Predictive analytics offers a viable solution to these challenges by providing actionable insights that drive strategic decision-making. According to a report by McKinsey, businesses that effectively leverage data analytics are 23 times more likely to outperform their competitors in customer acquisition and 19 times more likely to achieve above-average profitability. This underscores the transformative potential of predictive analytics in supply chain management.
Moreover, globalization has introduced a new level of complexity to supply chains, necessitating advanced analytics to manage and optimize cross-border operations. Predictive analytics enables companies to anticipate and mitigate potential disruptions, such as geopolitical tensions or supply shortages, ensuring business continuity. By integrating predictive insights into their strategies, companies can better align their operations with market demands and enhance their competitive positioning.
Navigate supply chain management with Meegle. Sign up for free and see the difference!
Historical development
Origins of Predictive Analytics
The origins of predictive analytics can be traced back to the early days of statistical analysis and business intelligence. In the mid-20th century, industries began to recognize the potential of quantitative data analysis to improve business operations. One of the earliest applications of predictive analytics in business was the use of linear regression for inventory management and sales forecasting. Companies like Ford and General Electric pioneered the use of statistical models to optimize production schedules and reduce costs.
As businesses increasingly embraced data-driven decision-making, predictive analytics evolved to encompass a broader range of applications. In the 1980s and 1990s, advancements in computer technology and data storage capabilities facilitated the development of more sophisticated models. The advent of enterprise resource planning (ERP) systems enabled companies to collect and analyze large volumes of data, paving the way for more accurate and comprehensive predictive analytics.
Over the years, key developments in data science, such as the introduction of machine learning algorithms and neural networks, have significantly enhanced the capabilities of predictive analytics. These advancements have allowed businesses to move beyond traditional statistical models and leverage more dynamic and adaptive predictive techniques. Today, predictive analytics is an integral part of supply chain management, enabling companies to optimize their operations, reduce risks, and improve customer satisfaction.
Evolution Over Time
The evolution of predictive analytics has been driven by advancements in computational power and data availability. In recent decades, the exponential growth of digital data has created new opportunities for businesses to leverage predictive analytics to gain a competitive edge. According to a report by IDC, the global datasphere is projected to grow from 33 zettabytes in 2018 to 175 zettabytes by 2025. This data explosion has enabled companies to develop more accurate and nuanced predictive models.
The rise of cloud computing and big data analytics has further accelerated the evolution of predictive analytics, providing businesses with the tools and resources to process and analyze massive amounts of data in real time. These technologies have democratized access to predictive analytics, allowing companies of all sizes to harness the power of data-driven insights. For example, retailers can use predictive analytics to forecast demand with greater accuracy, optimize inventory levels, and enhance customer experiences.
Shifts in global supply chain dynamics have also influenced the evolution of predictive analytics. The increasing complexity of supply chains, driven by globalization and the rise of e-commerce, has created new challenges and opportunities for businesses. Predictive analytics has become a critical tool for companies to navigate these complexities, enabling them to anticipate and respond to changing market conditions, manage risks, and improve operational efficiency.
Related:
Youth And EntrepreneurshipClick here to read our expertly curated top picks!
Core concepts
Defining Predictive Analytics
Predictive analytics is a subset of advanced analytics that uses statistical techniques, data mining, and machine learning to analyze current and historical data in order to make predictions about future events. The core components of predictive analytics include data collection, data preprocessing, model selection, and model evaluation. These components work together to uncover patterns and trends that inform strategic decision-making.
Data mining is a crucial aspect of predictive analytics, involving the extraction of valuable information from large datasets. This process includes identifying relationships and patterns within the data that can be used to build predictive models. Statistical modeling, another key component, involves creating mathematical models that represent the underlying structure of the data. These models are used to make predictions about future outcomes based on historical data.
Machine learning plays a pivotal role in predictive analytics by enabling models to learn from data and improve their predictive accuracy over time. Machine learning algorithms can automatically identify patterns and relationships within the data, allowing for more accurate and reliable predictions. For example, in supply chain management, machine learning algorithms can be used to forecast demand, optimize inventory levels, and identify potential supply chain disruptions.
Notable Features
Predictive analytics offers a range of key features and capabilities that make it a valuable tool for supply chain management. One of the most notable features is real-time data processing, which allows businesses to analyze and act on data as it is generated. This capability enables companies to respond quickly to changing market conditions, optimize their operations, and enhance customer satisfaction.
Another important feature of predictive analytics is scenario analysis, which involves evaluating different scenarios to assess their potential impact on business operations. This capability enables companies to anticipate potential risks and develop contingency plans to mitigate their impact. For example, a company might use scenario analysis to assess the potential impact of a supply chain disruption and develop strategies to ensure business continuity.
Demand forecasting is another critical feature of predictive analytics, allowing companies to anticipate customer demand and optimize inventory levels. By accurately forecasting demand, businesses can reduce inventory costs, improve customer satisfaction, and enhance their overall operational efficiency. Predictive analytics also enables companies to identify trends and patterns in customer behavior, allowing them to tailor their products and services to meet customer needs.
Strategic implementation
Planning and Execution
Effectively integrating predictive analytics into supply chain operations requires careful planning and execution. The first step in this process is to align predictive analytics initiatives with business objectives and supply chain strategies. This involves defining clear goals and objectives for the use of predictive analytics, such as improving demand forecasting, optimizing inventory levels, or enhancing customer satisfaction.
Once the objectives have been defined, it is important to develop a comprehensive implementation plan that outlines the steps and resources required to achieve these goals. This plan should include a timeline, budget, and resource allocation, as well as a clear delineation of roles and responsibilities. It is also important to establish key performance indicators (KPIs) to measure the success of the predictive analytics initiatives and ensure they are aligned with business objectives.
Collaboration and communication are critical to the successful implementation of predictive analytics in supply chain operations. It is essential to foster cross-functional collaboration between different departments, such as sales, marketing, and operations, to ensure that predictive analytics initiatives are integrated into the overall business strategy. Regular communication and feedback loops can help identify potential issues and ensure that predictive analytics initiatives are continuously refined and optimized.
Best Practices
To optimize the use of predictive analytics in supply chain management, it is important to follow established best practices. One of the most critical best practices is ensuring data quality and accuracy. High-quality data is essential for developing accurate and reliable predictive models. This involves implementing robust data governance processes to ensure data is collected, processed, and stored accurately.
Another best practice is fostering cross-functional collaboration and communication. Predictive analytics initiatives should involve input and collaboration from various departments, such as sales, marketing, and operations, to ensure they are aligned with overall business objectives. Regular communication and feedback loops can help identify potential issues and ensure that predictive analytics initiatives are continuously refined and optimized.
Continuous improvement is another important best practice for optimizing the use of predictive analytics in supply chain management. This involves regularly reviewing and refining predictive models to ensure they remain accurate and relevant in changing market conditions. It also involves staying informed about the latest advancements in predictive analytics technologies and methodologies to ensure that predictive analytics initiatives remain cutting-edge.
Click here to read our expertly curated top picks!
Technological integration
Tools and Technologies
The latest technological advancements have significantly enhanced the functionality of predictive analytics in supply chain management. A variety of software, platforms, and tools are available to facilitate the implementation of predictive analytics in supply chain contexts. These technologies enable companies to process and analyze large volumes of data in real time, allowing for more accurate and reliable predictions.
One key technology is machine learning, which enables predictive models to learn from data and improve their accuracy over time. Machine learning algorithms can automatically identify patterns and relationships within the data, allowing for more accurate and reliable predictions. For example, in supply chain management, machine learning algorithms can be used to forecast demand, optimize inventory levels, and identify potential supply chain disruptions.
Cloud computing is another important technology that enhances the functionality of predictive analytics. Cloud-based platforms provide companies with the resources and tools to process and analyze large volumes of data in real time. This capability enables companies to respond quickly to changing market conditions and optimize their operations. Cloud-based platforms also offer scalability and flexibility, allowing companies to adjust their predictive analytics capabilities as needed.
Impact of Digital Transformation
Digital transformation has had a profound impact on the use and development of predictive analytics in supply chains. The rise of the Internet of Things (IoT) has enabled companies to collect and analyze vast amounts of data from connected devices, providing valuable insights into supply chain operations. IoT devices can monitor and track various aspects of the supply chain, such as inventory levels, equipment performance, and transportation logistics, enabling companies to identify potential issues and optimize their operations.
Big data analytics has also played a significant role in enhancing predictive capabilities. The ability to process and analyze large volumes of data in real time has enabled companies to develop more accurate and reliable predictive models. This capability allows companies to anticipate and respond to changing market conditions, manage risks, and improve operational efficiency.
Cloud computing has also played a crucial role in the digital transformation of predictive analytics. Cloud-based platforms provide companies with the resources and tools to process and analyze large volumes of data in real time, allowing for more accurate and reliable predictions. Cloud-based platforms also offer scalability and flexibility, allowing companies to adjust their predictive analytics capabilities as needed.
Case studies and real-world applications
Successful implementations
Successful implementations
Retail Supply Chain Optimization
In the retail sector, predictive analytics has been instrumental in enhancing inventory management and demand forecasting. A notable example is the case of a major retail chain that leveraged predictive analytics to optimize its supply chain operations. By analyzing historical sales data and customer behavior, the company was able to predict demand spikes during peak shopping seasons, such as Black Friday and the holiday period. This enabled the retailer to adjust its inventory levels accordingly, reducing overstock and stockouts. As a result, the company improved its sales performance and customer satisfaction, ultimately boosting its market share.
Manufacturing Efficiency Improvement
Predictive analytics has also proven to be a valuable tool for optimizing production schedules and reducing downtime in the manufacturing industry. A leading automotive manufacturer implemented predictive analytics to monitor equipment performance and predict maintenance needs. By analyzing sensor data from production machinery, the company was able to identify patterns indicative of potential equipment failures. This proactive approach allowed the manufacturer to schedule maintenance before failures occurred, minimizing downtime and maintaining production efficiency. The result was a significant reduction in operational costs and an increase in overall production output.
Logistics and Distribution Enhancement
In the logistics industry, predictive analytics has been used to improve delivery performance and reduce operational costs. A logistics company implemented predictive analytics to optimize its delivery routes and schedules. By analyzing historical delivery data and traffic patterns, the company was able to predict potential delays and adjust its routes accordingly. This led to improved delivery times and reduced fuel consumption, ultimately lowering operational costs. The company also used predictive analytics to anticipate customer demand, allowing it to allocate resources efficiently and enhance service levels.
Lessons learned
Lessons learned
The case studies presented above offer valuable insights into the successful implementation of predictive analytics in supply chain management. One key takeaway is the importance of aligning predictive analytics initiatives with business objectives. Companies that clearly define their goals and objectives for predictive analytics are more likely to achieve successful outcomes.
Another important lesson is the value of cross-functional collaboration and communication. Successful implementation of predictive analytics requires input and collaboration from various departments, such as sales, marketing, and operations. This ensures that predictive analytics initiatives are integrated into the overall business strategy and aligned with company objectives.
Data quality and governance are also critical factors for success. High-quality data is essential for developing accurate and reliable predictive models. Companies must implement robust data governance processes to ensure data is collected, processed, and stored accurately.
Finally, continuous improvement is key to optimizing the use of predictive analytics. Companies must regularly review and refine predictive models to ensure they remain accurate and relevant in changing market conditions. Staying informed about the latest advancements in predictive analytics technologies and methodologies is also essential to ensure initiatives remain cutting-edge.
Click here to read our expertly curated top picks!
Future trends
Emerging Developments
The future of predictive analytics in supply chains is shaped by several emerging trends and developments. One key trend is the increasing integration of artificial intelligence (AI) into predictive analytics. AI-powered algorithms have the potential to enhance the accuracy and reliability of predictive models by automating the process of pattern recognition and data analysis. This capability allows businesses to anticipate and respond to changing market conditions more effectively.
Another emerging development is the use of blockchain technology to enhance data security and transparency in supply chains. Blockchain offers a decentralized and immutable ledger that can be used to track and verify transactions, providing a secure and transparent record of supply chain activities. This technology has the potential to enhance data integrity and trust, enabling more accurate and reliable predictive analytics.
Advanced machine learning algorithms are also playing a significant role in the evolution of predictive analytics. These algorithms can process and analyze vast amounts of data in real time, enabling more accurate and nuanced predictions. As machine learning continues to evolve, businesses will be able to leverage more sophisticated predictive models to optimize their supply chain operations and enhance their competitive positioning.
Predictions and Projections
As predictive analytics continues to evolve, several expert predictions and projections highlight its potential future impact on supply chains. One key prediction is that predictive analytics will become increasingly integrated into every aspect of supply chain management, from demand forecasting and inventory management to risk analysis and customer service. This integration will enable companies to optimize their operations and enhance their overall efficiency and competitiveness.
Another projection is that predictive analytics will play a critical role in enhancing sustainability in supply chains. By providing insights into resource utilization and waste reduction, predictive analytics can help companies minimize their environmental impact and achieve their sustainability goals. This capability will become increasingly important as companies face growing pressure to adopt sustainable practices and reduce their carbon footprint.
The future of predictive analytics in supply chains is also expected to be shaped by advances in technology, such as the rise of the Internet of Things (IoT) and big data analytics. These technologies will enable businesses to collect and analyze vast amounts of data in real time, providing valuable insights into supply chain operations. As a result, companies will be able to anticipate and respond to changing market conditions more effectively, enhancing their competitive positioning.
Related:
Process Flow DiagramClick here to read our expertly curated top picks!
Do's and don'ts of predictive analytics implementation
Do's | Don'ts |
---|---|
Ensure data quality and accuracy | Overlook the importance of data governance |
Foster cross-departmental collaboration | Isolate predictive analytics from core teams |
Continuously monitor and refine models | Ignore feedback and resist adapting |
Start with clear, measurable objectives | Begin without a strategic plan |
Click here to read our expertly curated top picks!
Conclusion
Summing Up
In summation, predictive analytics has emerged as a transformative force in modern supply chains, offering businesses a powerful tool for optimizing operations and enhancing competitiveness. By leveraging data, statistical algorithms, and machine learning techniques, companies can anticipate future trends and make informed decisions that drive efficiency, reduce costs, and improve customer satisfaction. Predictive analytics has become indispensable in today's complex and fast-paced business environment, enabling companies to navigate the challenges of globalization, market volatility, and evolving customer expectations.
The successful implementation of predictive analytics requires careful planning and execution, as well as a commitment to continuous improvement. Companies must align their predictive analytics initiatives with business objectives, foster cross-functional collaboration, and ensure data quality and accuracy. By following established best practices and staying informed about the latest advancements in technology, businesses can harness the full potential of predictive analytics and achieve long-term success.
Final Thoughts
As the landscape of supply chain management continues to evolve, the importance of predictive analytics will only grow. Businesses that embrace data-driven decision-making and leverage predictive insights will be well-positioned to thrive in an increasingly competitive market. It is essential for supply chain professionals and aspiring project managers to stay informed about the latest developments in predictive analytics and continuously adapt their strategies to remain at the forefront of the industry.
Continued learning and adaptation are key to unlocking the full potential of predictive analytics in supply chain management. By staying informed about technological advancements and industry trends, professionals can develop innovative solutions to complex supply chain challenges and drive sustainable growth. The future of supply chains is data-driven, and predictive analytics will play a pivotal role in shaping the industry's evolution and success.
Navigate supply chain management with Meegle. Sign up for free and see the difference!
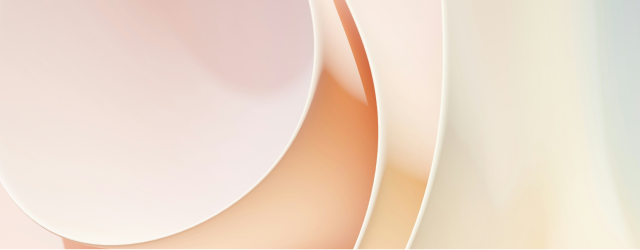
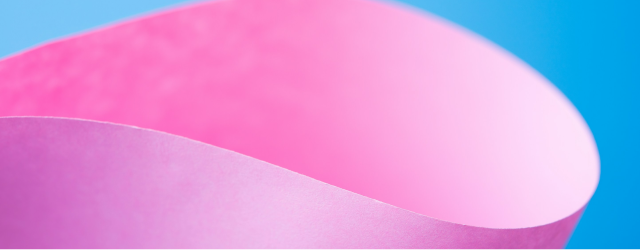
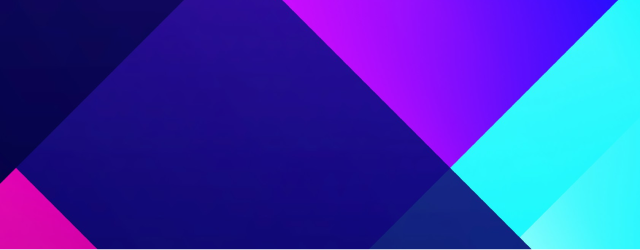
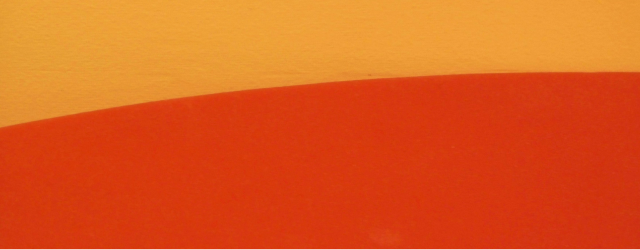
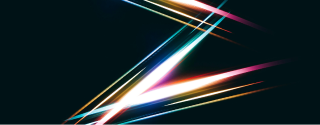
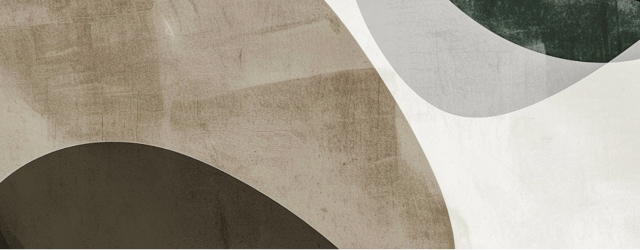
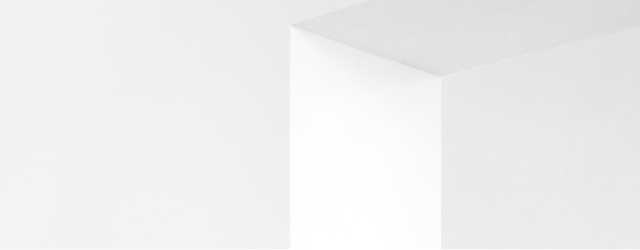