Data Analysis Teams
Discover how effective workflow management can transform efficiency and productivity for Data Analysis Teams, driving stronger business outcomes.
What is Workflow Management?
Workflow management refers to the systematic organization and direction of tasks and processes within a team or organization to achieve specific goals. It includes several components such as process mapping, task automation, and the continuous assessment of processes. For data analysis teams, workflow management is essential in orchestrating the sequence of data collection, processing, analysis, and reporting activities. Unlike simple task management which focuses on individual tasks, workflow management offers a strategic approach that integrates all tasks into a cohesive, streamlined process. This integration is vital for optimal efficiency and effectiveness in handling complex data-driven activities.
Workflow management's strategic importance lies in its ability to optimize and streamline data-centric processes. It ensures that every component of a data analysis task, from data cleaning to final reporting, is executed in harmony, minimizing redundancies and errors. By leveraging tools and techniques such as automation and process mapping, teams can visualize their workflows, uncover inefficiencies, and implement improvements. Effective workflow management can transform the chaotic, often siloed nature of data analysis into a well-oiled machine, ensuring that insights are delivered accurately and on time.
Importance for Data Analysis Teams
For data analysis teams, the importance of workflow management cannot be overstated. It is the backbone of achieving better accuracy, efficiency, and team coordination. In a field that demands precision, even minor errors can lead to significant misinterpretations and faulty decision-making. Workflow management helps mitigate these risks by ensuring a structured approach to data handling. It facilitates clear communication and collaboration among team members, aligning their efforts towards common objectives.
Moreover, efficient workflow management directly impacts project timelines and the speed at which data-driven decisions are made. With clearly defined processes and automated tasks, teams can reduce the time spent on mundane, repetitive tasks, freeing up resources for more critical, analytical work. This efficiency not only improves the quality of insights but also enhances the team's ability to meet tight deadlines and adapt quickly to new projects or changes in strategy. In essence, effective workflow management is a catalyst for driving the impact and success of data analysis endeavors within any organization.
Turn Project Chaos Into a Visual and Structured Plan
Key components of effective workflow management
Process Mapping
Process mapping is a pivotal component of effective workflow management. It involves creating a visual representation of the sequence of activities in a workflow, enabling teams to see the big picture of their operations. For data analysis teams, process mapping is instrumental in identifying bottlenecks, redundancies, and inefficiencies that can hinder productivity and accuracy. By breaking down complex processes into manageable steps, teams can better understand each phase of data handling, from initial data collection to the final reporting.
Visualizing workflows through process mapping provides clarity and insight into where improvements can be made. It allows teams to pinpoint areas where resources are being wasted or where tasks are being duplicated unnecessarily. By addressing these inefficiencies, teams can streamline their processes, allocate resources more effectively, and ultimately deliver insights more quickly and accurately. Tools like flowcharts, swimlane diagrams, and process flow diagrams are commonly used in process mapping to represent workflows. These tools help teams standardize their procedures and communicate them effectively to new team members, ensuring consistency and quality in data analysis activities.
Task Automation
Task automation is a powerful strategy for enhancing the efficiency of data analysis workflows. By automating repetitive, time-consuming tasks, teams can focus their efforts on more complex, value-added activities. Task automation involves using software tools and platforms to execute tasks automatically, without manual intervention. This can include data entry, data cleaning, report generation, and even certain aspects of data analysis itself.
The benefits of task automation for data analysis workflows are numerous. It reduces the risk of human error, improves consistency and accuracy, and frees up valuable time for team members to engage in more strategic tasks. Additionally, automation can increase the speed at which tasks are completed, allowing teams to deliver insights more quickly and respond to business needs in a timely manner. However, it's important to strike a balance between automation and human oversight. While automation can handle routine tasks efficiently, human judgment and expertise are still crucial for interpreting data and making informed decisions. Quality control measures should be in place to ensure that automated processes are functioning correctly and producing reliable results.
Click here to explore our expertly curated top project templates!
Planning and setting up workflows for data analysis teams
Initial Assessment
Conducting an initial assessment is the first step in planning and setting up effective workflows for data analysis teams. This involves evaluating the current state of workflow processes to identify strengths, weaknesses, and bottlenecks. Understanding the existing workflow landscape is crucial for determining areas that require improvement and for designing workflows that are truly tailored to the team's needs. The assessment process should include gathering input from team members to gain insights into their experiences and challenges with current workflows.
Several tools and techniques can be used to conduct workflow assessments. Surveys and interviews with team members can provide valuable qualitative data on workflow efficiency and effectiveness. Data-driven assessments, such as time-tracking and performance metrics, can offer quantitative insights into how resources are being utilized. Identifying common pain points, such as delays in data processing or communication breakdowns, can guide teams in prioritizing areas for improvement. By thoroughly understanding their existing workflows, data analysis teams can lay the groundwork for designing custom workflows that enhance productivity and accuracy.
Designing Custom Workflows
Designing custom workflows involves creating processes that are specifically tailored to the unique needs and characteristics of a data analysis team. This requires careful consideration of factors such as team structure, complexity of tasks, data volume, and project timelines. Custom workflows should align with the team's strategic goals and support their ability to deliver insights efficiently and accurately.
When designing custom workflows, it's important to break down complex tasks into manageable steps and assign responsibilities to team members based on their expertise and strengths. Collaboration and communication are key, as workflows often involve multiple team members working on different aspects of a project simultaneously. Additionally, incorporating flexibility into workflows can allow teams to adapt to changing requirements and priorities. Examples of successful custom workflows in data analysis include implementing automated data cleaning processes, creating standardized reporting templates, and establishing clear feedback loops for continuous improvement. By designing workflows that cater to their specific needs, data analysis teams can optimize their processes and drive better outcomes.
Implementation strategies
Integration with Existing Systems
Integrating new workflows with existing systems is a critical step in the implementation process. Data analysis teams often rely on a variety of tools and platforms for data collection, processing, and visualization. Ensuring compatibility between new workflows and these existing systems is essential for a seamless transition and optimal performance. Challenges may arise when integrating new workflows, such as data format discrepancies or software compatibility issues. However, these challenges can be addressed with careful planning and collaboration with IT and technical support teams.
Strategies for successful integration include conducting a thorough analysis of existing systems to identify potential compatibility issues and working closely with technical teams to develop solutions. Additionally, testing new workflows in a controlled environment before full implementation can help identify and resolve any integration challenges. Examples of successful integrations include incorporating automated data pipelines into existing data storage systems or implementing new data visualization tools that complement existing reporting processes. By ensuring that new workflows are compatible with existing systems, data analysis teams can maximize the benefits of workflow improvements and enhance their overall efficiency.
Training and Adoption
Training and adoption are key factors in the successful implementation of new workflows. Data analysis teams must be equipped with the knowledge and skills needed to effectively use new workflows and tools. Comprehensive training programs should be developed to familiarize team members with the new processes and technologies. These programs should include hands-on training sessions, documentation, and resources for ongoing learning and support.
Encouraging adoption and overcoming resistance to new workflows requires a strategic approach. It's important to communicate the benefits of new workflows to the team and involve them in the implementation process. Providing opportunities for feedback and incorporating team input into workflow design can increase buy-in and ownership. Leadership plays a crucial role in fostering a culture of continuous improvement and encouraging team members to embrace new ways of working. By prioritizing training and adoption, data analysis teams can successfully implement new workflows and achieve greater efficiency and effectiveness.
Click here to explore our expertly curated top project templates!
Monitoring and optimization
Performance Metrics
Monitoring performance metrics is essential for evaluating the effectiveness of workflows and identifying areas for improvement. Performance metrics provide insights into how well workflows are functioning and whether they are delivering the desired outcomes. Both quantitative and qualitative metrics should be tracked to gain a comprehensive understanding of workflow performance. Quantitative metrics may include time taken to complete tasks, error rates, and resource utilization. Qualitative metrics may include team satisfaction and feedback on workflow processes.
For data analysis teams, specific metrics that are particularly relevant include the accuracy and timeliness of data analysis results, the efficiency of data processing and cleaning tasks, and the quality of data visualizations and reports. Regularly reviewing these metrics allows teams to identify trends and patterns, pinpoint areas for improvement, and make data-driven decisions to optimize workflows. By continuously monitoring performance metrics, data analysis teams can ensure that their workflows remain efficient and effective over time.
Continual Improvement Processes
Continual improvement processes are essential for maintaining and enhancing the effectiveness of workflows. Data analysis teams should adopt agile methodologies and feedback loops to regularly evaluate and refine their workflows. Agile methodologies, such as iterative development and regular feedback sessions, allow teams to adapt to changing requirements and priorities. Feedback loops, such as regular team meetings and performance reviews, provide opportunities for team members to share insights and suggest improvements.
Successful continual improvement processes involve setting clear goals and objectives for workflow optimization and encouraging collaboration and innovation among team members. Examples of continual improvement processes in data analysis teams include implementing regular retrospectives to reflect on workflow successes and challenges, conducting pilot tests of new tools or processes before full implementation, and creating a culture of learning and experimentation. By embracing continual improvement, data analysis teams can enhance their workflows and drive better outcomes over time.
Common challenges and solutions
Addressing Resistance to Change
Resistance to change is a common challenge faced by data analysis teams when implementing new workflows. Team members may be hesitant to adopt new processes due to fear of the unknown, concerns about increased workload, or skepticism about the benefits of change. To address resistance, it's important to understand the underlying reasons and address them through effective communication and engagement strategies.
Strategies for addressing resistance to change include involving team members in the design and implementation of new workflows, providing clear explanations of the benefits and rationale for change, and offering support and resources for learning and adaptation. Leadership plays a crucial role in fostering a growth mindset and encouraging team members to embrace new ways of working. By creating a supportive and inclusive environment, teams can overcome resistance and successfully implement new workflows.
Solving Technical Hurdles
Technical hurdles are another common challenge in implementing new workflows for data analysis teams. These challenges may include software compatibility issues, data format discrepancies, and integration challenges. To overcome these hurdles, it's important to conduct thorough assessments of existing systems and collaborate with technical support teams to develop solutions.
Solutions for overcoming technical challenges include conducting pilot tests of new workflows in a controlled environment, developing standardized data formats and protocols, and providing training and resources for technical troubleshooting. Additionally, leveraging external resources, such as vendor support and online communities, can provide valuable insights and solutions for technical challenges. By proactively addressing technical hurdles, data analysis teams can successfully implement new workflows and achieve greater efficiency and effectiveness.
Click here to explore our expertly curated top project templates!
Examples of successful workflow management in data analysis
Example 1: streamlining data collection
Example 1: streamlining data collection
In one case study, a data analysis team at a leading e-commerce company significantly improved their data collection processes through effective workflow management. The team faced challenges with inconsistent data formats and manual data entry errors, which hindered their ability to deliver accurate and timely insights. To address these issues, the team implemented a new data collection workflow that included automated data pipelines and standardized data formats. By leveraging tools such as ETL (Extract, Transform, Load) platforms, the team was able to streamline data collection, reduce errors, and improve the accuracy and timeliness of their insights. As a result, the team was able to deliver more reliable and actionable insights to support business decision-making.
Example 2: enhancing data visualization
Example 2: enhancing data visualization
Another example of successful workflow management involves a data analysis team at a financial services company that improved their data visualization processes. The team struggled with creating clear and compelling visualizations that effectively communicated insights to stakeholders. To address this challenge, the team implemented a new workflow that included standardized data visualization templates and automated data visualization tools. By leveraging tools such as Tableau and Power BI, the team was able to create more consistent and impactful visualizations, improving stakeholder engagement and decision-making. The new workflow also allowed the team to save time and resources, enabling them to focus on more complex data analysis tasks.
Example 3: automating data reporting
Example 3: automating data reporting
In another case study, a data analysis team at a healthcare organization transformed their data reporting workflows through task automation. The team faced challenges with manual data entry and reporting processes, which were time-consuming and prone to errors. To address these issues, the team implemented a new workflow that included automated data reporting tools and processes. By leveraging tools such as automated reporting software and data integration platforms, the team was able to reduce manual data entry errors, improve the accuracy and timeliness of their reports, and free up valuable resources for more strategic tasks. As a result, the team was able to deliver more accurate and actionable insights to support healthcare decision-making and patient care.
Click here to explore our expertly curated top project templates!
Do's and don'ts for workflow management in data analysis teams
Do's | Don'ts |
---|---|
Encourage collaboration and feedback | Ignore team input and specific needs |
Utilize automation where possible | Automate processes without proper oversight |
Regularly review and update workflows | Allow workflows to become outdated |
Click here to explore our expertly curated top project templates!
Conclusion
Recap of Benefits
Effective workflow management offers numerous benefits for data analysis teams, including improved efficiency, accuracy, and team coordination. By streamlining processes and eliminating inefficiencies, teams can deliver insights more quickly and accurately, supporting data-driven decision-making and business success. Workflow management also enhances team collaboration and communication, aligning efforts towards common objectives and driving better outcomes.
Encouraging Ongoing Development
Ongoing development and refinement of workflows are essential for maintaining and enhancing their effectiveness over time. Data analysis teams should embrace a culture of continuous improvement, regularly evaluating and optimizing their processes to adapt to changing requirements and priorities. By staying adaptable and open to new technologies and methodologies, teams can ensure that their workflows remain efficient and effective, driving long-term success and innovation in the data analysis landscape.
Turn Project Chaos Into a Visual and Structured Plan
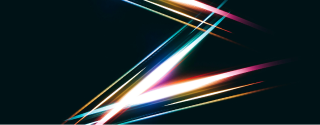
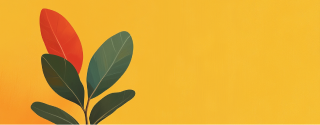
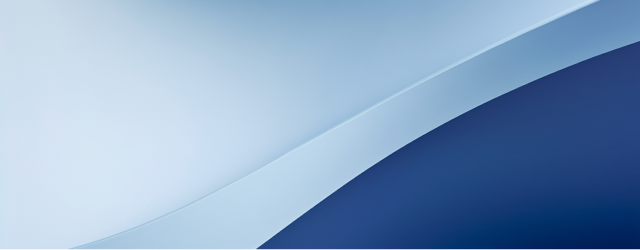
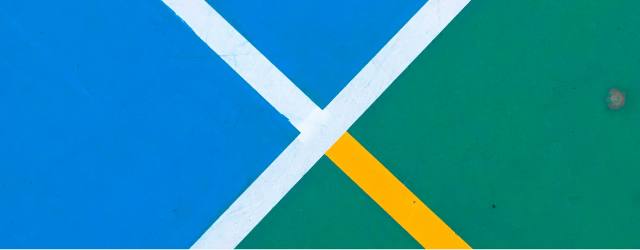
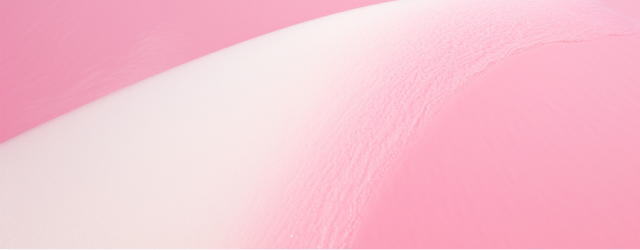
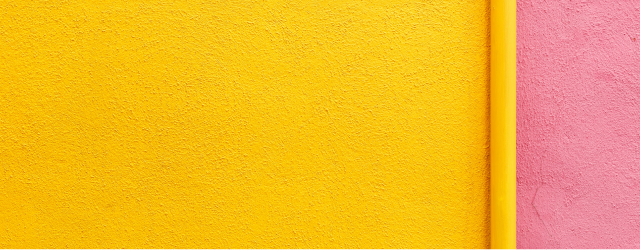
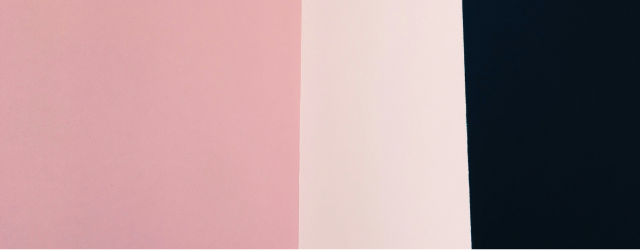