Big Data Analytics
Enhance industry performance and operational intelligence with Big Data Analytics, a key component in the realm of Digital Twin technology.
Big Data Analytics represents a transformative capability in today’s data-driven world. It involves the complex process of examining large and varied data sets to uncover hidden patterns, unknown correlations, market trends, customer preferences, and other useful business information. This process enables organizations to make more informed business decisions and drives innovation. Within the broader digital landscape, Big Data Analytics has risen to prominence as businesses increasingly rely on data to gain competitive advantages.
Simultaneously, Digital Twin Technology has gained importance across modern industries. This technology creates virtual replicas of physical assets, processes, or systems, allowing businesses to simulate, predict, and optimize operations. The integration of Big Data Analytics with Digital Twin Technology is particularly powerful because it enhances the accuracy and depth of these simulations. By providing real-time data and historical insights, Big Data Analytics fuels the Digital Twin with the information it needs to function effectively, leading to improved operational efficiency and reduced risk.
Experience a New Level of Productivity in Meegle
Concept and definition
Detailed Definition of Big Data Analytics
Big Data Analytics refers to the use of advanced analytic techniques against very large, diverse data sets that include structured, semi-structured, and unstructured data, from different sources, and in different sizes. It involves several key components and processes, including data collection, processing, storage, and analysis. The goal is to extract meaningful insights that can lead to better decision-making and strategic business moves.
The primary components of Big Data Analytics include:
- Data Mining: The process of discovering patterns in large data sets.
- Machine Learning: A type of artificial intelligence that allows software applications to become more accurate at predicting outcomes without being explicitly programmed to do so.
- Predictive Analytics: A branch of advanced analytics used to make predictions about unknown future events.
- Descriptive Analytics: This type focuses on interpreting historical data to identify trends and patterns.
Despite its many advantages, Big Data Analytics is often misunderstood. A common misconception is that it is only useful for large corporations due to its scale. However, businesses of all sizes can benefit from Big Data Analytics by tailoring solutions to their specific needs and capabilities.
How Big Data Analytics Fit into the Broader Digital Twin Framework
Digital Twin Technology refers to the creation of a virtual model of a process, product, or service. This pairing of the virtual and physical worlds allows data analysis and system monitoring to head off problems before they occur, prevent downtime, develop new opportunities, and even plan for the future by using simulations.
Big Data Analytics plays a crucial role in enhancing the functionality of Digital Twins. By integrating analytic insights, Digital Twins can become more dynamic and responsive. They utilize real-time data from IoT devices and sensors, historical data, and even external data sources to optimize operations and provide predictive insights. This symbiotic relationship ensures that any changes in the physical state of a system are accurately reflected in its digital counterpart, facilitating precise monitoring and control.
Historical development
Brief History of Big Data Analytics Evolution
The evolution of Big Data Analytics is a story of technological advancement and growing data demands. It began as a response to the massive increase in data generated by digital transactions and internet activity. Early on, traditional data processing tools were overwhelmed by the volume, velocity, and variety of data, leading to the development of more sophisticated analytic methods and technologies.
Key developments in Big Data Analytics include the creation of Hadoop in 2005, an open-source framework that allows for the distributed storage and processing of large data sets. This was a significant milestone, as it provided the infrastructure to manage and analyze big data efficiently. Over the years, advancements in data processing technology, cloud computing, and machine learning have further shaped Big Data Analytics, making it more accessible and powerful.
The transition from traditional data processing to advanced analytics has been driven by the realization that data holds the key to gaining competitive advantage. Companies like Google and Facebook were among the early adopters, using Big Data Analytics to enhance their algorithms and improve user experiences. This trend has only accelerated as more industries recognize the transformative potential of data-driven insights.
Milestones in the Development and Application of Big Data Analytics
Several pivotal moments have marked the development and application of Big Data Analytics. The launch of Apache Spark in 2014 was a game-changer, offering a powerful tool for processing data in real-time. This capability has been crucial for industries that require rapid insights, such as finance and telecommunications.
Industry-specific milestones highlight the diverse applications of Big Data Analytics. In healthcare, analytics has enabled precision medicine by allowing doctors to tailor treatments based on genetic information. In manufacturing, predictive maintenance powered by analytics has significantly reduced downtime and maintenance costs.
The evolution of tools and platforms supporting Big Data Analytics continues to drive innovation. Platforms like Google Cloud and Amazon Web Services have democratized access to analytics, allowing businesses of all sizes to leverage these technologies. The development of user-friendly analytics tools has further accelerated adoption, enabling professionals without a technical background to gain valuable insights from their data.
Core applications
Core applications and functionalities of big data analytics in various industries
Core applications and functionalities of big data analytics in various industries
Big Data Analytics is transforming industries across the board by offering targeted applications and functionalities that address specific needs. In healthcare, for example, analytics is used to predict patient outcomes, personalize treatments, and manage healthcare resources efficiently. By analyzing patient data, healthcare providers can identify risk factors, improve diagnostics, and enhance patient care.
In manufacturing, Big Data Analytics optimizes the production process, improves supply chain management, and enhances product quality. By analyzing data from production lines, manufacturers can identify bottlenecks, predict machine failures, and reduce waste, resulting in increased efficiency and cost savings.
The finance industry uses Big Data Analytics to detect fraud, assess credit risk, and personalize banking services. By analyzing transaction data, financial institutions can identify suspicious activities, make informed lending decisions, and offer personalized financial advice to their customers.
Examples of real-world usage impacting business outcomes
Examples of real-world usage impacting business outcomes
Numerous case studies illustrate the successful implementation of Big Data Analytics and its impact on business outcomes. For instance, Netflix uses analytics to understand viewer preferences and create content that resonates with its audience. By analyzing viewing data, Netflix can predict which shows will be successful and tailor its content offerings accordingly, leading to increased subscriber satisfaction and retention.
In the retail sector, companies like Amazon use Big Data Analytics to optimize pricing strategies, manage inventory, and personalize marketing efforts. By analyzing purchase patterns and customer behavior, Amazon can offer personalized recommendations, optimize pricing, and ensure that popular products are always in stock.
These real-world examples demonstrate how Big Data Analytics can drive business success by providing actionable insights that enhance decision-making, improve customer experiences, and optimize operations.
Benefits and advantages
Detailed Examination of the Benefits Big Data Analytics Bring to Operational Practices
The benefits of Big Data Analytics are manifold, significantly enhancing operational practices across industries. One of the primary advantages is the ability to gain efficiencies through data-driven insights. By analyzing large data sets, businesses can identify patterns and trends that were previously invisible, allowing them to optimize processes and reduce waste.
Another key benefit is the enhancement of decision-making processes. With access to accurate and timely data, decision-makers can make informed choices that align with strategic objectives. For example, in supply chain management, analytics can provide insights into demand patterns, enabling businesses to optimize inventory levels and reduce carrying costs.
Cost reductions and productivity improvements are also significant advantages of Big Data Analytics. By identifying inefficiencies and opportunities for improvement, businesses can streamline operations and reduce costs. In manufacturing, for instance, predictive maintenance powered by analytics can significantly reduce downtime and maintenance expenses, leading to increased productivity and profitability.
Comparative Analysis of Pre and Post Big Data Analytics Implementation
The impact of Big Data Analytics on operational performance is often stark when comparing pre- and post-implementation scenarios. Before the adoption of analytics, businesses may rely on intuition or limited data to make decisions, resulting in suboptimal outcomes.
Post-implementation, businesses can harness the power of data to drive strategic initiatives and optimize operations. Metrics such as reduced downtime, increased revenue, and improved customer satisfaction often showcase the tangible benefits of analytics deployment. Industry-specific examples illustrate these transformative effects, highlighting the potential for Big Data Analytics to revolutionize business practices.
Integration strategies
How to Effectively Integrate Big Data Analytics with Existing Systems
Integrating Big Data Analytics with existing systems requires careful planning and execution. A step-by-step approach can help ensure a seamless integration and maximize the benefits of analytics.
Step 1: Assess Current Infrastructure
Evaluate existing systems and identify any gaps or limitations in data management and analysis capabilities. This assessment will inform the planning and resource allocation for the integration process.
Step 2: Define Objectives and Scope
Clearly define the objectives of the analytics initiative and the scope of integration. Consider the specific business goals and the areas where analytics can provide the most value.
Step 3: Select Appropriate Tools and Platforms
Choose the right analytics tools and platforms that align with the organization's needs and capabilities. Consider factors such as scalability, ease of use, and compatibility with existing systems.
Step 4: Develop a Data Strategy
Create a comprehensive data strategy that outlines how data will be collected, stored, processed, and analyzed. Ensure that data quality and security measures are in place to protect sensitive information.
Step 5: Implement and Test
Execute the integration plan and conduct thorough testing to ensure that the analytics solution is functioning as intended. Address any technical challenges and refine the solution as needed.
Step 6: Train and Support
Provide training and support to ensure that team members are equipped to leverage the analytics tools effectively. Encourage a data-driven culture where insights are used to drive decision-making.
Challenges and Considerations in Integration
Integrating Big Data Analytics with existing systems can present several challenges. Common obstacles include data silos, technical complexity, and resistance to change within the organization. To overcome these challenges, businesses must adopt strategic approaches that address both technical and cultural aspects.
Data privacy and security are critical considerations in the integration process. Organizations must ensure that their data strategy aligns with regulatory requirements and that robust security measures are in place to protect sensitive information. Collaboration between IT and business units can facilitate a smoother integration and ensure that analytics initiatives align with organizational goals.
Future trends and predictions
Future Developments and Trends Expected with Big Data Analytics
As technology continues to evolve, several trends are expected to shape the future of Big Data Analytics. One significant trend is the increasing incorporation of artificial intelligence (AI) and machine learning into analytics processes. These technologies enhance the ability to derive insights from large data sets and improve the accuracy of predictions.
Emerging technologies such as edge computing and blockchain are also poised to influence Big Data Analytics. Edge computing allows data processing to occur closer to the source, reducing latency and improving real-time decision-making capabilities. Blockchain offers enhanced data security and transparency, making it an attractive option for industries that handle sensitive information.
Predictions for the evolution of analytics capabilities suggest that analytics will become more democratized, with user-friendly tools enabling non-technical professionals to gain insights from data. The continued development of natural language processing (NLP) will further enhance the accessibility of analytics, allowing users to interact with data using conversational interfaces.
Potential Impacts and Future Applications in Industry
The future of Big Data Analytics holds exciting possibilities for new industry applications. In manufacturing, analytics could facilitate the development of smart factories, where machines communicate and optimize processes autonomously. In healthcare, predictive analytics could enable more personalized and proactive care, improving patient outcomes and reducing costs.
The societal and economic impacts of advanced analytics are also significant. By enabling more efficient resource management and decision-making, analytics can contribute to sustainability efforts and drive economic growth. Continued innovation in analytics technologies will likely lead to new capabilities and applications, further transforming industries and society as a whole.
Use cases and case studies
Specific Case Studies Showcasing the Successful Application of Big Data Analytics
Healthcare: Predictive Analytics for Patient Outcomes
A leading healthcare provider implemented predictive analytics to improve patient outcomes and reduce hospital readmissions. By analyzing patient data, the provider was able to identify high-risk patients and develop targeted interventions. This approach led to a significant reduction in readmissions and improved patient satisfaction.
Manufacturing: Enhancing Operational Efficiency
A global manufacturing company used Big Data Analytics to optimize its production processes. By analyzing data from IoT sensors on the production line, the company identified inefficiencies and implemented changes that increased throughput and reduced waste. This resulted in cost savings and improved product quality.
Retail: Personalizing Customer Experience
A major retailer leveraged analytics to personalize the customer experience and drive sales. By analyzing purchase history and customer behavior, the retailer offered personalized recommendations and targeted promotions. This approach increased customer engagement and loyalty, leading to higher sales and profitability.
Lessons Learned and Insights Gained from these Implementations
Key takeaways from successful Big Data Analytics implementations include the importance of a clear strategy, strong leadership, and cross-functional collaboration. Organizations that align their analytics initiatives with strategic goals and foster a data-driven culture are more likely to achieve positive outcomes.
Overcoming common hurdles, such as data silos and resistance to change, requires a proactive approach that involves stakeholders from across the organization. By focusing on data quality, security, and user training, businesses can maximize the benefits of analytics and drive innovation.
Click here to read our expertly curated top picks!
Step-by-step guide to implementing big data analytics
Step 1: Preparation
Begin by assessing the organization's current data capabilities and identifying key objectives for the analytics initiative. Engage stakeholders and secure buy-in from leadership to ensure alignment with strategic goals.
Step 2: Execution
Develop a detailed plan for implementing Big Data Analytics, including selecting appropriate tools and platforms, developing a data strategy, and addressing technical and organizational challenges.
Step 3: Post-Implementation
Monitor the performance of the analytics solution and make adjustments as needed. Provide ongoing training and support to ensure that team members can effectively leverage the insights gained from analytics.
Do's and don'ts of big data analytics
Do's | Don'ts |
---|---|
Do invest in quality data infrastructure. | Don't overlook the importance of data privacy. |
Do ensure continuous training for your team. | Don't rely solely on historical data without context. |
Do integrate analytics into decision-making processes. | Don't neglect to update systems and methodologies. |
Conclusion
In conclusion, Big Data Analytics is a powerful tool that offers significant benefits to industries seeking to enhance efficiency and competitiveness. By embracing analytics, organizations can make informed decisions, optimize operations, and drive innovation. The integration of Big Data Analytics with Digital Twin Technology further amplifies these benefits, providing a dynamic and responsive approach to industry challenges. As the digital landscape continues to evolve, businesses are encouraged to explore and invest in Big Data Analytics to remain competitive and achieve long-term success.
Experience a New Level of Productivity in Meegle
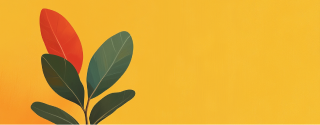
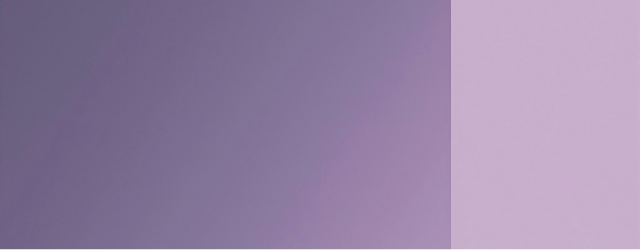
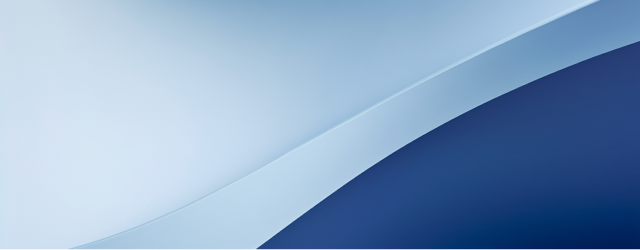
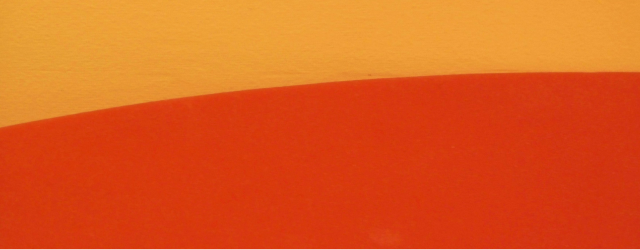
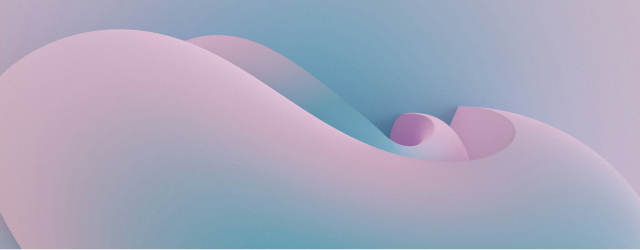
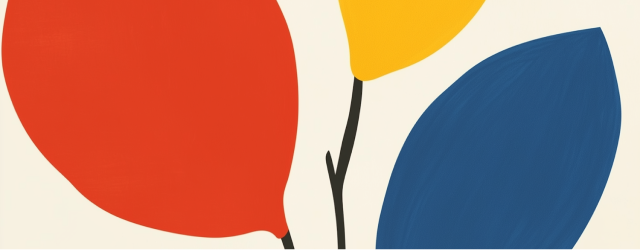
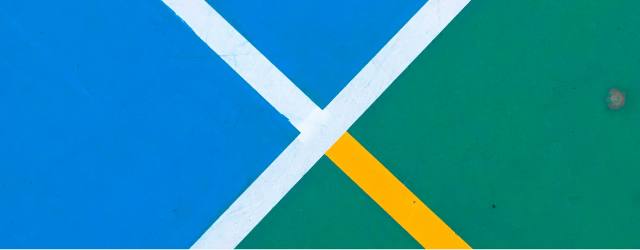