Real-Time Analytics
Enhance industry performance and operational intelligence with Real-time Analytics, a key component in the realm of Digital Twin technology.
In the current digital age, the ability to process and analyze data instantaneously is a game-changer for businesses across all sectors. Real-time analytics refers to the process of analyzing data as soon as it becomes available, enabling organizations to derive insights and make data-driven decisions without delay. This capability is increasingly vital in an environment where timely responses to market changes and operational dynamics can make or break a business.
Real-time analytics plays a pivotal role in enhancing operational efficiency. Unlike traditional analytics, which often involves batch processing of historical data, real-time analytics processes data on the fly. This allows businesses to gain immediate insights and respond to events as they happen. Industries such as finance, healthcare, manufacturing, and logistics are already leveraging real-time analytics to optimize operations, reduce costs, and improve customer experiences.
For instance, in the financial sector, real-time analytics is used to detect fraudulent transactions as they occur, reducing potential losses and enhancing security. In healthcare, it facilitates continuous patient monitoring and timely interventions. Manufacturing plants use real-time data to streamline production processes and maintain quality control. The common thread across these applications is the enhanced decision-making capability that real-time insights provide.
Experience a New Level of Productivity in Meegle
Concept and definition
What are Real-time Analytics?
Real-time analytics is a data processing technique that enables the instantaneous collection, analysis, and visualization of data. Unlike traditional batch processing methods, which analyze data after it has been collected and stored, real-time analytics processes information as it is received. This immediacy allows organizations to act swiftly on insights, making it crucial for applications where timing is of the essence.
Key characteristics of real-time analytics include:
- Low Latency: The ability to process data with minimal delay, allowing for immediate insights.
- Continuous Processing: Ongoing data processing that supports live updates and ongoing analysis.
- Dynamic Data Integration: Capability to integrate and analyze data from various sources in real-time.
Traditional analytics, on the other hand, involves the retrospective examination of data, often leading to delayed insights and slower response times. While both have their use cases, real-time analytics is indispensable in scenarios that demand rapid responsiveness.
The core components of real-time analytics systems typically include data sources (such as sensors or transaction logs), a real-time processing engine, and a user interface for visualization. Together, these components facilitate the seamless capture, processing, and display of data in real-time.
Real-time Analytics in the Digital Twin Framework
Digital twin technology creates a virtual representation of a physical asset or system, allowing for real-time monitoring and analysis. This concept is increasingly gaining traction in industries such as manufacturing, aerospace, and urban planning. When combined with real-time analytics, digital twins can offer a powerful tool for operational efficiency and innovation.
Real-time analytics significantly enhances the functionality of digital twins by providing up-to-the-minute data that reflects the current state of the physical entity. This continuous data influx allows digital twins to simulate various scenarios, predict outcomes, and optimize processes. For example, in manufacturing, a digital twin of a production line can use real-time data to identify bottlenecks and suggest adjustments to improve efficiency.
The symbiotic relationship between real-time analytics and digital twins is particularly evident in predictive maintenance. By continuously analyzing data from a digital twin, organizations can anticipate equipment failures before they occur, reducing downtime and maintenance costs. This proactive approach not only extends the lifespan of assets but also improves overall operational efficiency.
In industrial applications, this combination allows for the modeling and testing of different operational strategies without disrupting actual processes. By leveraging real-time analytics within the digital twin framework, businesses can explore new opportunities, optimize resource allocation, and drive innovation.
Click here to read our expertly curated top picks!
Historical development
Evolution of Real-time Analytics
The journey of real-time analytics is marked by significant technological advancements that have transformed how businesses process and utilize data. Historically, data analytics was confined to batch processing, where information was collected, stored, and analyzed after a certain period. This method, while useful for historical analysis, often led to delayed decision-making and missed opportunities.
The evolution of real-time analytics began in the late 20th century with the advent of faster computing systems and the rise of the internet. These technological advancements paved the way for real-time data processing, enabling businesses to analyze and act on data as it was generated. The emergence of big data technologies, such as Apache Kafka and Spark, further accelerated this evolution by providing platforms capable of handling large volumes of data in real-time.
A pivotal moment in the evolution of real-time analytics was the development of in-memory computing. This technology allows data to be stored and processed in a computer's RAM, significantly reducing latency and increasing processing speed. In-memory computing has been instrumental in enabling real-time analytics solutions that are both fast and scalable.
The historical context of real-time analytics within the broader field of data analytics is one of continuous innovation and adaptation. As businesses increasingly recognize the value of real-time insights, the demand for advanced analytics solutions continues to grow, driving further advancements in the field.
Milestones in Real-time Analytics
Several key milestones have shaped the development and application of real-time analytics. One of the earliest was the introduction of real-time operating systems (RTOS) in the 1960s, which enabled computers to process tasks in real-time. This laid the groundwork for more sophisticated real-time data processing technologies.
The 1990s saw the rise of enterprise resource planning (ERP) systems, which integrated various business processes and provided real-time access to data across an organization. This development was crucial in demonstrating the value of real-time data for operational efficiency and decision-making.
In the early 2000s, the advent of cloud computing revolutionized data processing by offering scalable, on-demand computational resources. This allowed businesses to implement real-time analytics without the need for extensive on-premise infrastructure. The introduction of big data technologies, such as Hadoop and Spark, further expanded the capabilities of real-time analytics by enabling the processing of large datasets at high speeds.
These milestones have had a profound impact on various industries, enabling them to harness the power of real-time analytics for enhanced decision-making and operational efficiency. Today's real-time analytics solutions are the result of decades of technological advancements and the continuous pursuit of more efficient data processing methods.
Core applications
Industry applications of real-time analytics
Industry applications of real-time analytics
Real-time analytics has found applications across a wide range of industries, each leveraging its capabilities to address specific challenges and opportunities. In healthcare, real-time analytics is used to monitor patient vitals, predict health outcomes, and improve care delivery. For instance, hospitals employ real-time analytics to manage patient flow, optimize resource allocation, and ensure timely interventions.
In the manufacturing sector, real-time analytics is used to optimize production processes, enhance quality control, and reduce waste. By analyzing data from sensors and machines in real-time, manufacturers can identify inefficiencies, predict equipment failures, and implement corrective actions before issues escalate.
The finance industry relies heavily on real-time analytics for risk management, fraud detection, and algorithmic trading. Financial institutions use real-time data to monitor market trends, detect suspicious transactions, and make informed investment decisions. This capability is crucial in an industry where split-second decisions can have significant financial implications.
Other industries, such as logistics and retail, use real-time analytics to optimize supply chain operations, manage inventory, and enhance customer experiences. By analyzing real-time data on consumer behavior, retailers can personalize marketing efforts, improve customer engagement, and drive sales.
Real-world usage and impact
Real-world usage and impact
Numerous companies and organizations have successfully harnessed the power of real-time analytics to achieve measurable business outcomes. For example, a leading automotive manufacturer implemented real-time analytics to monitor the performance of its production lines. This initiative led to a 20% increase in production efficiency and a 15% reduction in operational costs. By continuously analyzing data from sensors, the company was able to identify and address bottlenecks in real-time, resulting in smoother operations and higher output.
Another notable example is a healthcare provider that adopted real-time analytics to enhance patient care. By integrating real-time data from electronic health records, wearable devices, and monitoring equipment, the provider was able to predict patient deterioration and initiate timely interventions. This approach not only improved patient outcomes but also reduced hospital readmissions by 30%, leading to significant cost savings.
These case studies highlight the transformative impact of real-time analytics on business efficiency, profitability, and service delivery. By enabling organizations to make informed decisions based on current data, real-time analytics empowers them to operate more effectively and achieve their strategic goals.
Benefits and advantages
Operational Benefits
Real-time analytics offers a plethora of operational benefits that can significantly enhance business performance. One of the primary advantages is cost savings. By providing real-time insights into operations, businesses can identify inefficiencies, reduce waste, and optimize resource allocation. For instance, in the manufacturing sector, real-time analytics can identify energy-intensive processes and suggest optimizations, leading to lower energy costs.
Increased productivity is another key benefit of real-time analytics. By enabling organizations to monitor and manage operations in real-time, businesses can streamline processes, minimize downtime, and maximize output. This is particularly important in industries such as manufacturing and logistics, where operational efficiency directly impacts profitability.
Risk reduction is also a significant benefit of real-time analytics. By providing real-time visibility into operations, organizations can detect and address potential issues before they escalate. For example, in the finance industry, real-time analytics can detect fraudulent transactions as they occur, reducing the risk of financial losses and enhancing security.
Real-time analytics also enhances customer experience and service delivery. By analyzing real-time data on customer interactions, businesses can personalize services, improve response times, and deliver a more engaging customer experience. This capability is crucial in industries such as retail and hospitality, where customer satisfaction is a key competitive differentiator.
Comparative Analysis
The impact of real-time analytics on operational performance can be quantitatively measured by comparing performance metrics before and after implementation. For instance, a manufacturing company that implemented real-time analytics reported a 15% reduction in production costs and a 20% increase in output. These improvements were attributed to the ability to monitor and optimize production processes in real-time.
A financial institution that adopted real-time analytics for fraud detection reported a 30% reduction in fraudulent activities and a 25% increase in customer trust. By analyzing transactions in real-time, the institution was able to identify and block suspicious activities before they resulted in financial losses.
These examples illustrate how real-time analytics can transform business operations by providing actionable insights that drive efficiency, reduce costs, and enhance customer satisfaction. By leveraging real-time data, organizations can make informed decisions that align with their strategic objectives and deliver tangible business value.
Integration strategies
Effective Integration with Existing Systems
Integrating real-time analytics into existing technology infrastructures requires careful planning and execution. To ensure a successful integration, organizations should follow a structured approach that aligns with their business objectives and technical requirements.
One of the first steps in the integration process is conducting a thorough needs assessment. This involves evaluating the current technology infrastructure, identifying data sources, and defining the specific goals and objectives of the real-time analytics solution. This assessment helps organizations understand the scope of the integration and identify any potential challenges.
Next, organizations should invest in scalable infrastructure that can support real-time data processing. This may involve upgrading hardware, implementing cloud-based solutions, or leveraging big data technologies that offer high-speed processing capabilities. The chosen infrastructure should be flexible and scalable to accommodate growing data volumes and evolving business needs.
Aligning real-time analytics with business objectives is crucial for maximizing its value. This requires collaboration between IT teams and business units to ensure that the analytics solution addresses key business challenges and opportunities. By involving stakeholders throughout the integration process, organizations can ensure that the solution meets their needs and delivers the desired outcomes.
Challenges and Considerations
The integration of real-time analytics is not without its challenges. Common hurdles include data integration issues, technical complexities, and data security concerns. To overcome these challenges, organizations should adopt best practices and leverage proven solutions.
One common challenge is integrating data from diverse sources into a unified analytics platform. This requires robust data integration capabilities that can handle different data formats, sources, and protocols. Organizations should invest in data integration tools that offer seamless connectivity and support real-time data ingestion.
Technical complexities can also pose challenges during the integration process. To address these, organizations should ensure they have the necessary technical expertise and resources to implement and manage the real-time analytics solution. This may involve training existing staff, hiring specialists, or partnering with technology vendors.
Data security and integrity are critical considerations when implementing real-time analytics. Organizations should implement robust security measures to protect sensitive data and ensure compliance with regulations. This includes encryption, access controls, and regular security audits to safeguard data and maintain trust.
By addressing these challenges and considerations, organizations can successfully integrate real-time analytics into their operations and unlock its full potential.
Future trends and predictions
Emerging Trends in Real-time Analytics
The field of real-time analytics is continually evolving, driven by technological advancements and changing business needs. Several emerging trends are expected to shape the future of real-time analytics and its applications across industries.
One of the most significant trends is the integration of artificial intelligence (AI) and machine learning (ML) with real-time analytics. By incorporating AI and ML algorithms, organizations can enhance their ability to derive insights from real-time data and automate decision-making processes. This integration is expected to drive innovations in areas such as predictive analytics, anomaly detection, and personalized customer experiences.
Another trend is the growing adoption of edge computing in real-time analytics. Edge computing allows data processing to occur closer to the data source, reducing latency and enabling faster insights. This trend is particularly relevant in industries such as manufacturing and logistics, where real-time data from IoT devices and sensors is crucial for operational efficiency.
The rise of 5G technology is also expected to impact real-time analytics by enabling faster data transmission and supporting the proliferation of connected devices. This will facilitate more sophisticated real-time analytics applications, such as autonomous vehicles and smart city solutions.
As these trends continue to evolve, organizations must stay abreast of technological advancements and adapt their strategies to leverage the full potential of real-time analytics.
Future Industry Impacts
The future impacts of real-time analytics are expected to be transformative, reshaping how industries operate and strategize. In the healthcare sector, real-time analytics is anticipated to enhance personalized medicine and remote patient monitoring, improving patient outcomes and reducing healthcare costs.
In the manufacturing industry, real-time analytics is expected to drive the adoption of smart factories and Industry 4.0 initiatives. By enabling real-time monitoring and optimization of production processes, manufacturers can achieve higher efficiency, reduce waste, and improve product quality.
The finance industry is likely to see further advancements in algorithmic trading and fraud detection, driven by real-time analytics. By leveraging real-time data, financial institutions can enhance risk management, improve regulatory compliance, and deliver personalized financial services.
To capitalize on these future developments, businesses should invest in real-time analytics solutions that align with their strategic goals and provide a competitive advantage. By embracing technological innovations and staying adaptable, organizations can position themselves for success in an increasingly data-driven world.
Use cases and case studies
Example 1: Healthcare
A healthcare organization implemented real-time analytics to revolutionize patient care and resource management. By integrating real-time data from electronic health records, wearable devices, and monitoring equipment, the organization was able to continuously monitor patient vitals and predict health outcomes. This proactive approach enabled timely interventions, reducing hospital readmissions by 30% and improving patient outcomes.
The implementation of real-time analytics also enhanced operational efficiency by optimizing resource allocation and reducing wait times. By analyzing real-time data on patient flow and resource utilization, the organization was able to streamline processes, improve staff productivity, and enhance the overall patient experience. The lessons learned from this implementation include the importance of robust data integration capabilities and the need for collaboration between IT and clinical teams.
Example 2: Manufacturing
A leading manufacturing firm adopted real-time analytics to optimize its production processes and enhance quality control. By analyzing data from sensors and machines in real-time, the firm was able to identify inefficiencies, predict equipment failures, and implement corrective actions before issues escalated. This resulted in a 20% increase in production efficiency and a 15% reduction in operational costs.
Real-time analytics also played a crucial role in improving supply chain management. By providing real-time visibility into inventory levels and supplier performance, the firm was able to optimize procurement, reduce lead times, and minimize stockouts. The key takeaways from this case study include the importance of investing in scalable infrastructure and the value of real-time insights for operational optimization.
Example 3: Finance
A financial institution employed real-time analytics to enhance security and fraud detection. By analyzing transactions in real-time, the institution was able to detect and block suspicious activities before they resulted in financial losses. This proactive approach reduced fraudulent activities by 30% and increased customer trust by 25%.
Real-time analytics also improved compliance with regulatory requirements by providing real-time visibility into financial activities and enabling timely reporting. The insights gained from this implementation include the importance of robust data security measures and the need for continuous monitoring and refinement of analytics processes.
Click here to read our expertly curated top picks!
Do's and don'ts
Do's | Don'ts |
---|---|
Conduct thorough needs assessment | Rush the implementation process |
Invest in scalable infrastructure | Ignore data security and compliance |
Engage stakeholders throughout | Overlook training and support |
Continuously monitor and refine | Set unrealistic expectations |
Conclusion
The transformative power of real-time analytics lies in its ability to provide timely insights that drive efficiency, innovation, and strategic decision-making. By embracing real-time analytics and integrating it with digital twin technology, businesses can unlock new levels of operational efficiency and competitiveness. The future potential of real-time analytics is vast, and organizations that embrace this technology will be well-positioned to capitalize on emerging opportunities and navigate the challenges of an increasingly data-driven world.
Experience a New Level of Productivity in Meegle
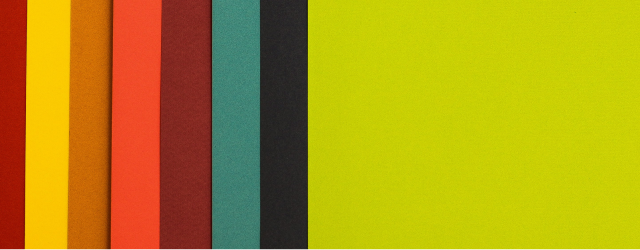
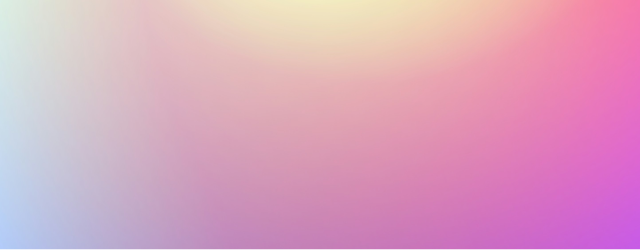
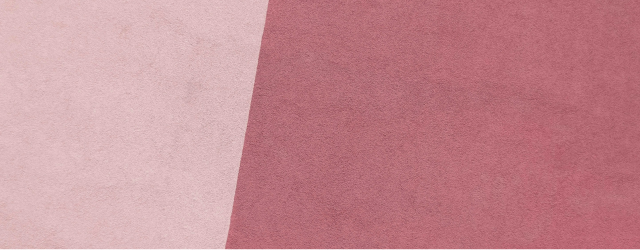
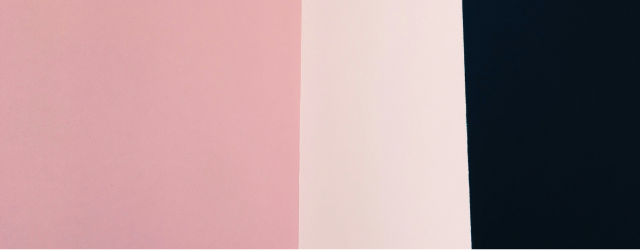
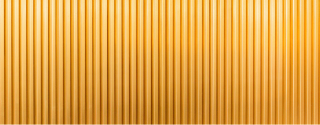
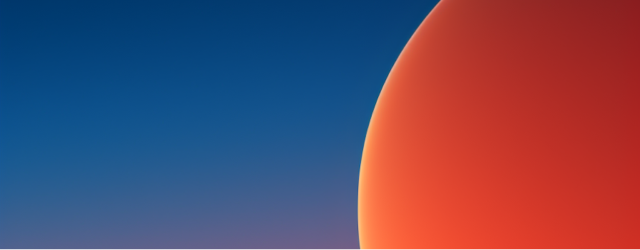
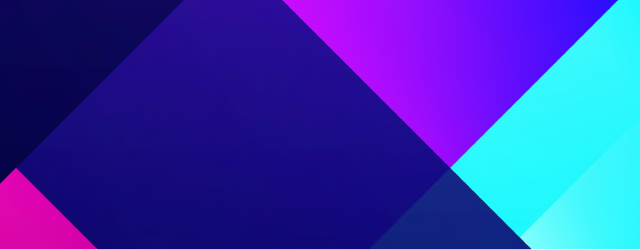
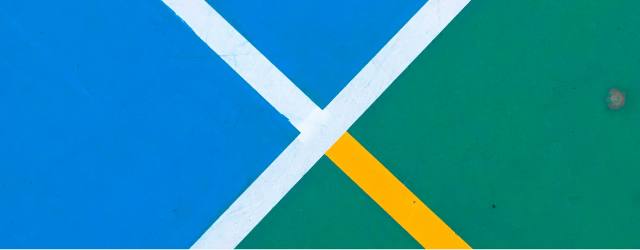