Data Accuracy
Enhance industry performance and operational intelligence with Data Accuracy, a key component in the realm of Digital Twin technology.
Introduction to Data Accuracy
Data accuracy refers to the extent to which data correctly reflects the real-world constructs it is intended to model. In essence, it is about ensuring that the data is precise, consistent, and free from errors, which is essential for making informed and reliable decisions. Accurate data is the foundation of any successful data-driven strategy, as it directly impacts the quality of insights derived from data analysis. In the context of digital twin technology, data accuracy becomes even more critical. Digital twins are virtual models that mirror real-world processes, systems, or objects, and they rely entirely on the accuracy of the data inputted to function effectively. The accuracy of this data ensures that the digital twin can provide a true-to-life representation, which is essential for simulating scenarios, predicting outcomes, and optimizing processes. The ripple effect of data accuracy extends beyond digital twins, impacting overall industry efficiency and innovation. By leveraging accurate data, organizations can streamline operations, reduce errors, and foster a culture of innovation that is data-driven and forward-thinking.
Experience a New Level of Productivity in Meegle
Concept and definition
Detailed Definition of Data Accuracy
In the realm of digital technology, data accuracy is defined as the degree to which data correctly represents the real-world phenomenon it is intended to describe. This encompasses several dimensions, including precision, correctness, and consistency. Precision refers to the level of detail and exactness of the data, ensuring that it is measured and recorded to the most appropriate level. Correctness implies that the data is free from errors and accurately reflects the reality it is meant to depict. Consistency is about maintaining uniformity and reliability across data sets over time. These dimensions collectively ensure that data can be trusted and used effectively for analysis and decision-making purposes. Inaccuracies in data can lead to flawed insights, misguided strategies, and ultimately, significant financial losses. Therefore, maintaining high levels of data accuracy is paramount in the digital age, where decisions are increasingly driven by data analytics and insights.
How Data Accuracy Fits into the Broader Digital Twin Framework
Digital twins are sophisticated virtual models that replicate physical systems, processes, or objects to allow for dynamic simulation and analysis. The success of a digital twin is inherently tied to the accuracy of the data it is fed. Accurate data allows digital twins to provide a true representation of their physical counterparts, enabling organizations to monitor performance, identify issues, and predict future outcomes with greater precision. The interplay between data accuracy and digital twin technology is pivotal in optimizing operations and enhancing decision-making processes. For instance, in manufacturing, a digital twin can simulate production line scenarios, identify potential bottlenecks, and suggest improvements, all of which rely on the accuracy of input data. Similarly, in healthcare, digital twins of patients can help in devising personalized treatment plans by accurately modeling patient data. The accuracy of data ensures that digital twins can deliver actionable insights and drive efficiencies across various industries.
Historical development
Brief History of Data Accuracy in the Field
The journey of data accuracy in digital systems has evolved significantly over the years. Initially, data accuracy was a secondary consideration, often overshadowed by the sheer volume of data being generated. However, as the limitations of inaccurate data became apparent, the emphasis on data accuracy intensified. In the early days of computing, data accuracy was primarily concerned with reducing typographical errors and ensuring data consistency across systems. As technology advanced, the focus shifted towards more sophisticated data validation and verification techniques. With the advent of big data and analytics, the importance of data accuracy skyrocketed, as organizations realized that the value of insights derived from data was only as good as the accuracy of the data itself. This led to the development of robust data management practices and the implementation of advanced technologies such as machine learning and AI to enhance data accuracy. Today, data accuracy is a critical component of digital strategies, driving innovation and efficiency across industries.
Milestones in the Development and Application of Data Accuracy
Several key milestones have shaped the journey of data accuracy, each contributing to the current practices in data management. One notable milestone was the introduction of database management systems (DBMS) in the 1960s, which provided structured methods for storing and retrieving data, thus enhancing data accuracy. The development of data cleansing and data quality tools in the 1990s further improved the precision and correctness of data. The emergence of data governance frameworks in the early 2000s marked another significant milestone, emphasizing the importance of maintaining data quality and accuracy across organizational processes. More recently, the integration of AI and machine learning algorithms has revolutionized data accuracy by automating data validation and error detection processes. These advancements have not only improved data accuracy but have also paved the way for innovative applications such as digital twin technology. By ensuring that data is accurate, organizations can leverage these technologies to drive efficiencies and gain a competitive edge in their respective industries.
Core applications
Core applications and functionalities of data accuracy in various industries
Core applications and functionalities of data accuracy in various industries
Data accuracy plays a pivotal role in driving performance and innovation across various industries. In manufacturing, accurate data is essential for optimizing production processes, ensuring quality control, and reducing waste. By leveraging accurate data, manufacturers can implement predictive maintenance strategies, minimize downtime, and enhance overall operational efficiency. In the healthcare sector, data accuracy is critical for patient diagnosis, treatment planning, and outcome prediction. Accurate patient data enables healthcare providers to deliver personalized care and improve patient outcomes. In the logistics industry, data accuracy ensures efficient supply chain management, inventory control, and timely delivery of goods. By maintaining accurate data on inventory levels, shipment schedules, and demand forecasts, logistics companies can streamline operations and reduce costs. Across these industries, accurate data serves as the foundation for strategic decision-making, enabling organizations to innovate and remain competitive in an increasingly digital world.
Examples of real-world usage impacting business outcomes
Examples of real-world usage impacting business outcomes
Improved data accuracy can lead to significant business benefits, as demonstrated by several real-world examples. One noteworthy case is that of a global automotive manufacturer that leveraged data accuracy to enhance its production processes. By implementing advanced data validation techniques, the company was able to reduce defects in its manufacturing line by 30%, resulting in substantial cost savings and improved product quality. In the healthcare sector, a leading hospital network utilized data accuracy to optimize patient care. By ensuring the accuracy of patient data across its systems, the network reduced medication errors by 25% and achieved a 15% improvement in patient satisfaction scores. In logistics, a major e-commerce company improved its delivery efficiency by 20% by implementing data accuracy measures to optimize its supply chain operations. These examples highlight the transformative impact of data accuracy on business outcomes, demonstrating how organizations can achieve significant efficiencies and competitive advantages by prioritizing accurate data.
Benefits and advantages
Detailed Examination of the Benefits Data Accuracy Brings to Operational Practices
The benefits of data accuracy in operational practices are extensive and multifaceted. One of the primary advantages is the reduction of errors in processes, which directly translates to cost savings and improved efficiency. Accurate data ensures that decisions are based on reliable information, minimizing the risk of costly mistakes and resource wastage. Additionally, data accuracy enhances the quality of insights derived from data analysis, enabling organizations to make more informed decisions and drive strategic initiatives. By reducing data discrepancies and inconsistencies, organizations can streamline operations and improve overall productivity. Data accuracy also plays a crucial role in maintaining compliance with regulatory requirements, ensuring that data handling practices meet industry standards and avoid potential legal issues. Furthermore, accurate data fosters a culture of trust within organizations, as stakeholders can rely on the integrity of the data for decision-making purposes. Overall, the benefits of data accuracy extend beyond operational efficiencies, driving innovation and competitive advantage across industries.
Comparative Analysis of Pre and Post Data Accuracy Implementation
A comparative analysis of scenarios before and after the implementation of data accuracy measures reveals significant improvements in processes and outcomes. For instance, a major telecommunications company experienced a 40% reduction in customer complaints related to billing errors after implementing data accuracy initiatives. By enhancing the accuracy of customer data, the company was able to improve billing precision and enhance customer satisfaction. Similarly, a global retail chain reduced inventory discrepancies by 50% after prioritizing data accuracy in its supply chain operations. This led to better inventory control, reduced stockouts, and improved sales performance. In the healthcare sector, a hospital system achieved a 30% reduction in patient readmissions by ensuring the accuracy of patient data across its electronic health records. These examples illustrate the quantifiable benefits of data accuracy, highlighting how organizations can achieve significant improvements in performance and outcomes by prioritizing accurate data.
Integration strategies
How to Effectively Integrate Data Accuracy with Existing Systems
Integrating data accuracy with existing systems requires a strategic approach and the right set of tools and technologies. One effective strategy is to implement data validation and cleansing processes to ensure that data is accurate and consistent before it is integrated into existing systems. This can involve the use of automated tools that detect and correct data errors, reducing the reliance on manual checks. Additionally, organizations can establish data governance frameworks to maintain data quality and accuracy across all systems and processes. This involves setting clear policies and standards for data handling and ensuring that all stakeholders are aligned with these practices. Another key strategy is to leverage advanced technologies such as AI and machine learning to automate data accuracy processes. These technologies can analyze large volumes of data in real-time, identifying discrepancies and ensuring that data remains accurate and up-to-date. By adopting these strategies, organizations can effectively integrate data accuracy with existing systems, enhancing overall efficiency and performance.
Challenges and Considerations in Integration
While integrating data accuracy with existing systems offers significant benefits, it also presents several challenges that organizations must navigate. One common challenge is the complexity of legacy systems, which may not be compatible with modern data accuracy tools and technologies. Integrating these systems can require significant time and resources, and organizations must carefully plan and execute the integration process to avoid disruptions. Another challenge is the need for staff training and engagement, as employees must be well-versed in data accuracy practices to ensure successful integration. Additionally, organizations must consider data privacy and security concerns, ensuring that data accuracy measures do not compromise the integrity of sensitive information. To overcome these challenges, organizations can adopt best practices such as conducting thorough assessments of existing systems, involving all stakeholders in the integration process, and leveraging expert guidance and support. By addressing these challenges proactively, organizations can ensure a smooth and successful integration of data accuracy with existing systems.
Future trends and predictions
Future Developments and Trends Expected with Data Accuracy
As technology continues to evolve, several future developments and trends are expected to shape the landscape of data accuracy. One key trend is the increasing use of artificial intelligence and machine learning to enhance data accuracy processes. These technologies can analyze vast amounts of data in real-time, identifying errors and inconsistencies with greater precision and efficiency. Another trend is the growing emphasis on data governance and quality frameworks, as organizations recognize the importance of maintaining data accuracy across all processes and systems. Additionally, the rise of the Internet of Things (IoT) is expected to drive demand for data accuracy, as the proliferation of connected devices generates massive amounts of data that must be accurately managed and analyzed. The integration of blockchain technology is also anticipated to enhance data accuracy by providing a decentralized and tamper-proof method of data validation and verification. Overall, these trends are expected to drive significant advancements in data accuracy, enabling organizations to leverage accurate data for innovation and competitive advantage.
Potential Impacts and Future Applications in Industry
The potential impacts and future applications of data accuracy in industry are vast and transformative. In the manufacturing sector, data accuracy is expected to enhance predictive maintenance capabilities, reducing downtime and improving overall equipment efficiency. In healthcare, accurate data will enable more precise patient monitoring and personalized treatment plans, improving patient outcomes and reducing costs. The logistics industry is also set to benefit from data accuracy, with enhanced supply chain management and inventory control driving efficiencies and reducing waste. Additionally, the integration of data accuracy with emerging technologies such as augmented reality and virtual reality is expected to create new opportunities for innovation and engagement. These applications highlight the transformative potential of data accuracy, enabling organizations to optimize operations, drive innovation, and achieve sustainable growth in an increasingly competitive landscape.
Use cases and case studies
Specific Case Studies Showcasing the Successful Application of Data Accuracy
Several case studies illustrate the successful application of data accuracy and its impact on business outcomes. In the automotive industry, a leading manufacturer implemented data accuracy measures to improve its production line efficiency. By enhancing the accuracy of its production data, the company reduced defects by 30%, resulting in significant cost savings and improved product quality. In healthcare, a hospital network utilized data accuracy to optimize patient care. By ensuring the accuracy of patient data, the network reduced medication errors by 25% and achieved a 15% improvement in patient satisfaction scores. In the logistics sector, a major e-commerce company improved its delivery efficiency by 20% by implementing data accuracy measures to optimize its supply chain operations. These case studies highlight the transformative impact of data accuracy on business outcomes, demonstrating how organizations can achieve significant efficiencies and competitive advantages by prioritizing accurate data.
Lessons Learned and Insights Gained from These Implementations
The successful implementation of data accuracy measures offers valuable lessons and insights for organizations looking to enhance their data management practices. One key lesson is the importance of adopting a strategic approach to data accuracy, involving the right tools, technologies, and frameworks to ensure success. Organizations must also prioritize staff training and engagement, as employees play a crucial role in maintaining data accuracy across all processes and systems. Another important insight is the need for continuous monitoring and improvement of data accuracy practices, as data quality and accuracy must be maintained over time. By applying these lessons and insights, organizations can effectively enhance their data accuracy practices, driving efficiencies and achieving sustainable growth in an increasingly competitive landscape.
Do's and don'ts in ensuring data accuracy
Do's | Don'ts |
---|---|
Regularly audit data for accuracy | Ignore discrepancies or errors |
Use automated tools for data validation | Rely solely on manual checks |
Train staff in best data practices | Overlook the importance of data quality training |
Implement consistent data policies | Allow inconsistent data entry |
Stay updated with latest technologies | Resist updates to legacy systems |
Conclusion
Summary of the Critical Roles and Benefits of Data Accuracy
In conclusion, data accuracy plays a critical role in advancing digital twin technology and enhancing industry efficiency. Accurate data serves as the foundation for reliable decision-making, enabling organizations to optimize operations, reduce errors, and drive innovation. By prioritizing data accuracy, industries can achieve significant efficiencies and competitive advantages, paving the way for sustainable growth in an increasingly digital landscape. The benefits of data accuracy extend beyond operational improvements, fostering a culture of trust and accountability within organizations.
Encouragement to Adopt or Further Explore Data Accuracy in Industry Practices
As industries continue to evolve, it is imperative for organizations to prioritize data accuracy and explore its potential applications. By adopting data accuracy measures and leveraging advanced technologies, organizations can unlock new levels of efficiency and innovation. We encourage industry leaders to take proactive steps towards enhancing data accuracy, ensuring that their organizations remain competitive and resilient in the face of technological advancements.
Experience a New Level of Productivity in Meegle
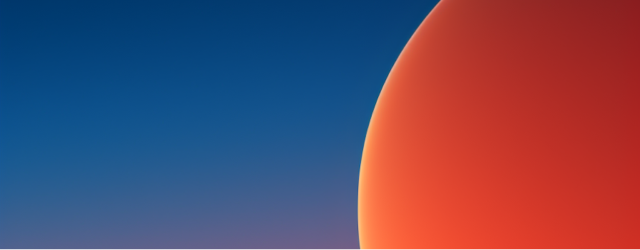
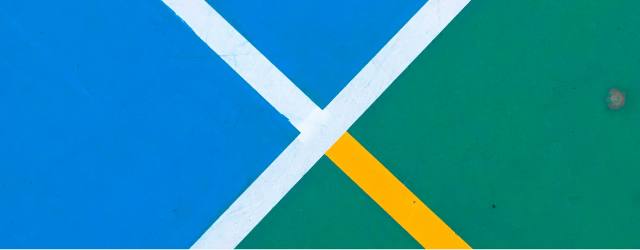
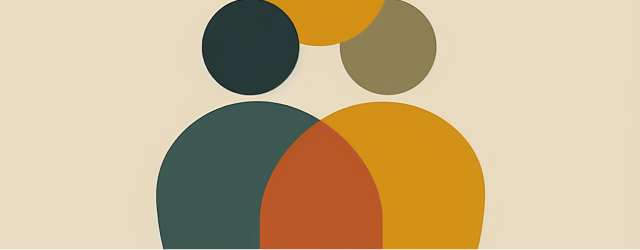
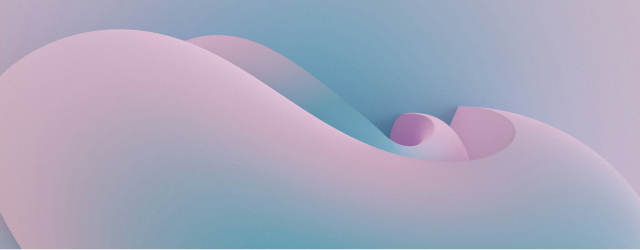
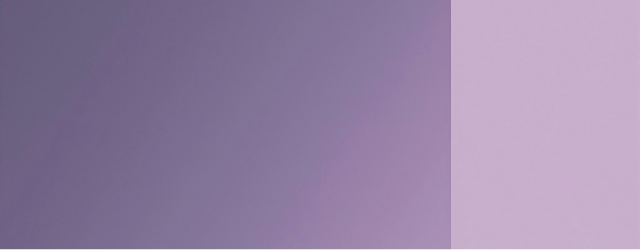
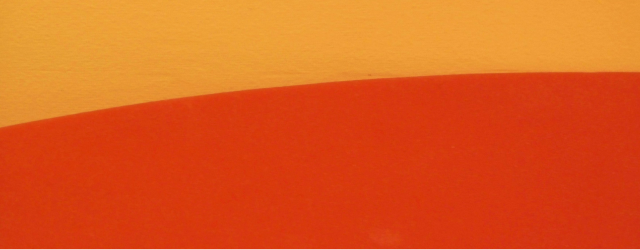
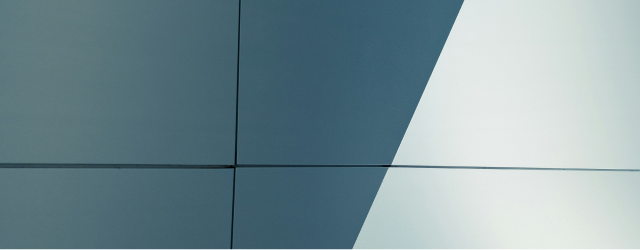
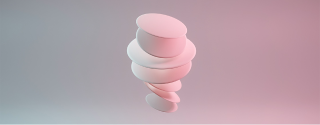