AI In Autonomous Vehicles
Explore the foundations, applications, and future of AI in Autonomous Vehicles, illustrating its significant impact on technology and industry. Deep dive into real-world case studies and emerging trends.
The Role of AI in Modern Transportation
Artificial intelligence in autonomous vehicles represents a cutting-edge intersection of technology and transportation. The application of AI in these vehicles involves sophisticated systems such as machine learning, computer vision, and advanced sensor technologies, which enable vehicles to perform tasks traditionally requiring human intervention. These AI systems are critical for processing vast amounts of data from the vehicle's environment, allowing for real-time decision-making and navigation. The importance of AI-driven vehicles in modern transportation is underscored by their potential to reduce traffic accidents, improve traffic flow, and decrease carbon emissions through optimized driving patterns.
Reshaping the Automotive Industry
AI is not only reshaping how vehicles operate but is also fundamentally transforming the automotive industry itself. Traditional automakers are increasingly investing in AI technologies to stay competitive, while tech companies are entering the automotive space with innovative solutions. The shift towards AI-driven vehicles is prompting automakers to rethink vehicle design, manufacturing processes, and business models. Furthermore, as AI technologies continue to advance, they are paving the way for new services and applications in transportation, such as ride-sharing platforms and delivery services, which rely heavily on autonomous vehicle capabilities.
Build powerful workflows with Meegle for free!
Historical background of ai in autonomous vehicles
Evolution of AI Technologies in the Automotive Sector
The journey of AI in autonomous vehicles is marked by numerous technological breakthroughs and innovations. The evolution of AI technologies in the automotive sector can be traced back to the mid-20th century when early experiments in robotics and automation laid the groundwork for today’s advancements. Early initiatives focused on developing basic automated systems, such as cruise control, which gradually evolved into more complex AI-driven solutions. Over the years, significant milestones have been achieved, including the development of autonomous prototypes by research institutions and technology companies.
Key Milestones and Breakthroughs
One of the most notable milestones in the development of autonomous vehicles was the DARPA Grand Challenge in 2004, which showcased the potential of autonomous technology. This competition spurred significant advancements in autonomous navigation and control systems. Subsequent breakthroughs have been driven by advancements in sensor technologies, machine learning algorithms, and data processing capabilities, leading to the development of fully autonomous vehicles capable of operating in diverse environments.
Keep Reading
Key technologies and methodologies in ai in autonomous vehicles
Machine Learning and Computer Vision
Machine learning and computer vision are at the heart of AI systems in autonomous vehicles, enabling them to perceive and understand their environment. Machine learning algorithms allow vehicles to learn from data and improve their performance over time. These algorithms are used for tasks such as object detection, lane recognition, and traffic sign identification. Computer vision, on the other hand, equips vehicles with the ability to interpret visual information from cameras and sensors, facilitating real-time decision-making.
Neural Networks and Deep Learning
Neural networks and deep learning play a crucial role in enhancing vehicle autonomy by providing advanced capabilities in pattern recognition and decision-making. Deep learning models can process large datasets and extract meaningful insights, allowing vehicles to navigate complex environments with higher accuracy. These models are particularly effective in handling unpredictable scenarios, such as pedestrian crossings and dynamic traffic situations, which are essential for achieving full autonomy.
Case studies: ai in autonomous vehicles in action
Tesla's Autopilot
Tesla’s Autopilot system is a prime example of AI in autonomous vehicles, demonstrating the potential and challenges of AI-driven technology. Since its introduction, Tesla’s Autopilot has significantly impacted consumer trust in AI, offering features such as automated lane-keeping, adaptive cruise control, and self-parking. Despite its success, the system has faced scrutiny over safety concerns, highlighting the importance of continuous improvement and robust testing in AI systems.
Waymo's Public Trials
Waymo, a subsidiary of Alphabet Inc., has been at the forefront of autonomous vehicle development, conducting extensive public trials to advance navigation precision. Waymo’s autonomous vehicles utilize a combination of sensors, machine learning algorithms, and high-definition mapping to navigate urban environments. These trials have provided valuable insights into the operational challenges and societal implications of deploying autonomous vehicles on public roads.
Uber's Self-Driving Trucks
Uber’s venture into self-driving trucks demonstrates the potential of AI in transforming logistics and supply chain operations. By leveraging AI technologies, Uber has developed autonomous trucks capable of long-haul transportation, reducing the need for human drivers and optimizing delivery routes. This initiative highlights the economic benefits of AI-driven logistics, including cost savings and increased efficiency.
Keep Reading
Challenges and limitations of ai in autonomous vehicles
Technological and Practical Challenges
Despite the promising advancements in AI for autonomous vehicles, several technological and practical challenges remain. One of the primary challenges is ensuring the safety and reliability of AI systems in diverse and unpredictable driving conditions. This requires extensive testing and validation to address potential system failures and minimize risks. Additionally, the integration of AI with existing transportation infrastructure poses logistical challenges, necessitating updates to road systems and traffic management protocols.
Ethical and Data Privacy Concerns
The deployment of autonomous vehicles also raises ethical and data privacy concerns. Ethical dilemmas arise when AI systems must make critical decisions in unavoidable accident scenarios, such as choosing between the safety of passengers and pedestrians. Moreover, the collection and processing of vast amounts of data by autonomous vehicles raise privacy concerns, necessitating robust data protection measures and transparent data usage policies.
Future of ai in autonomous vehicles
Emerging Trends
The future landscape of AI in autonomous vehicles is shaped by emerging trends such as 5G connectivity and quantum computing. 5G networks offer faster data transmission speeds and lower latency, enhancing the real-time capabilities of autonomous systems. Quantum computing holds the potential to revolutionize data processing, enabling AI algorithms to solve complex problems more efficiently.
Predictions and Global Mobility
As AI technologies continue to evolve, they are expected to have a significant impact on global mobility. Autonomous vehicles have the potential to revolutionize urban transportation, reducing congestion and improving accessibility. In the coming years, we can expect to see increased adoption of AI-driven vehicles in various sectors, including public transportation, logistics, and personal mobility.
Economic and social impact of ai in autonomous vehicles
Economic Benefits and Business Models
The integration of AI in autonomous vehicles offers numerous economic benefits, including cost savings, increased efficiency, and new business models. Autonomous vehicles have the potential to reduce operational costs for transportation companies by minimizing the need for human drivers and optimizing fuel consumption. Additionally, AI-driven vehicles enable new business models, such as shared mobility services and autonomous delivery platforms, creating opportunities for innovation and growth.
Job Displacement and Workforce Reskilling
While AI in autonomous vehicles presents economic opportunities, it also poses challenges related to job displacement. The automation of driving tasks may lead to the displacement of traditional driving jobs, necessitating workforce reskilling and upskilling initiatives. It is essential for stakeholders to collaborate on strategies that support workers in transitioning to new roles within the evolving transportation ecosystem.
Regulatory and ethical considerations of ai in autonomous vehicles
Current Regulations and Policies
The regulatory landscape for autonomous vehicles is continuously evolving, with governments and regulatory bodies working to establish comprehensive guidelines and standards. Current regulations focus on ensuring the safety and reliability of autonomous systems, addressing issues such as testing protocols, liability, and data privacy. However, the pace of regulatory development varies across regions, highlighting the need for international cooperation in harmonizing standards.
Ethical Dilemmas and International Cooperation
The deployment of autonomous vehicles also presents ethical dilemmas, such as decision-making in critical situations and the allocation of responsibility in accidents. These dilemmas require careful consideration and the development of ethical frameworks to guide AI system design and operation. International cooperation is essential in creating comprehensive guidelines that address these ethical challenges and promote responsible innovation in the autonomous vehicle industry.
Keep Reading
Tips for do's and don'ts
Do's | Don'ts |
---|---|
Prioritize safety in AI design | Ignore regulatory requirements |
Stay updated with technological trends | Neglect data security measures |
Engage in ethical AI practices | Overlook public perception issues |
Ensure extensive testing | Rush deployment without adequate trials |
Keep Reading
Conclusion
In conclusion, the integration of artificial intelligence in autonomous vehicles represents a transformative leap in transportation technology. As this field continues to evolve, it holds the potential to reshape mobility, offering significant benefits in safety, efficiency, and accessibility. However, realizing the full potential of AI in autonomous vehicles requires addressing the technological, ethical, and regulatory challenges that accompany its development. By prioritizing safety, engaging in responsible innovation, and fostering international cooperation, stakeholders can navigate the future of transportation with AI, ensuring a sustainable and inclusive mobility ecosystem for all.
Build powerful workflows with Meegle for free!
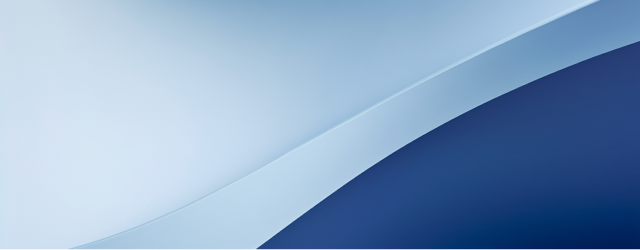
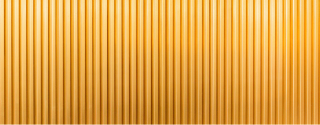
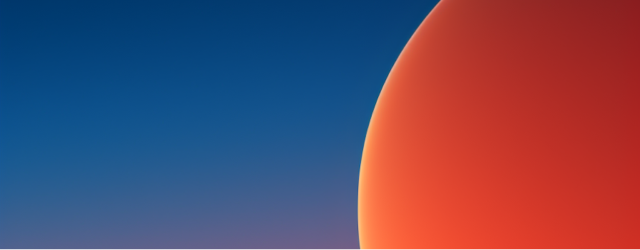
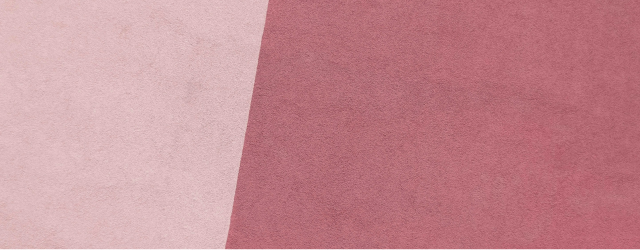
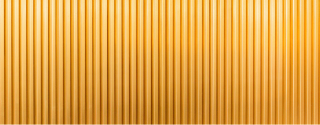
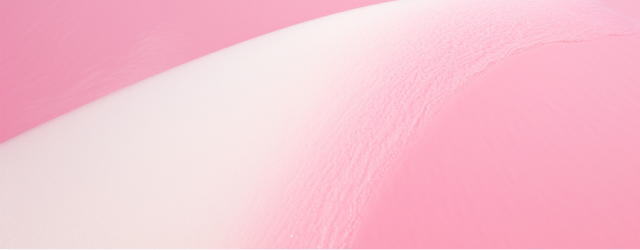
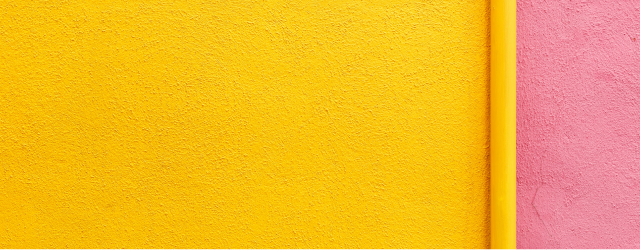
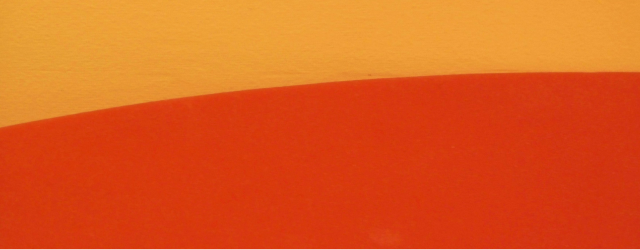