AI In Manufacturing Industries
Explore the foundations, applications, and future of AI in Manufacturing Industries, illustrating its significant impact on technology and industry. Deep dive into real-world case studies and emerging trends.
Defining AI in the Manufacturing Context
Artificial Intelligence, or AI, is a broad term that encompasses various technologies designed to mimic human intelligence. In the context of manufacturing, AI refers to the implementation of machine learning, robotics, and data analytics to streamline production processes. Machine learning, a subset of AI, involves algorithms that enable machines to learn and make predictions based on data. Robotics refers to the use of robots to automate tasks, enhancing precision and efficiency. Data analytics, on the other hand, involves analyzing vast amounts of data to derive actionable insights, helping manufacturers make informed decisions.
The Growing Relevance of AI in Manufacturing
The relevance of AI in manufacturing cannot be overstated. As global competition intensifies, manufacturers are under pressure to produce high-quality products while minimizing costs. AI technologies have emerged as vital tools in achieving these goals. For instance, machine learning algorithms can predict equipment failures, allowing for predictive maintenance that reduces downtime and extends the life of machinery. Robotics can automate repetitive tasks, freeing human workers to focus on more complex and value-added activities. Furthermore, data analytics enables manufacturers to optimize supply chains, reduce waste, and improve overall efficiency. According to a report by McKinsey, AI-driven manufacturing can potentially boost productivity by up to 40%, underscoring its transformative potential.
Article Scope and Focus Areas
This article aims to provide a comprehensive overview of AI in manufacturing industries. We will explore key technologies and methodologies driving AI adoption, including predictive maintenance, computer vision, and autonomous robotics. Real-world case studies from industry leaders like Siemens, Tesla, and BMW will illustrate AI's practical applications. We'll also discuss the challenges and limitations manufacturers face when implementing AI, as well as ethical and regulatory considerations. Finally, we'll look ahead to emerging trends and future directions, offering insights into how AI will continue to shape the manufacturing landscape. By the end of this article, readers will have a deeper understanding of AI's impact on manufacturing and the strategies necessary to harness its full potential.
Build powerful workflows with Meegle for free!
Historical background of ai in manufacturing industries
Tracing the Evolution of AI in Manufacturing
The history of AI in manufacturing is a testament to technological evolution and innovation over the decades. It begins with the early automation systems of the 1950s and 1960s, which laid the groundwork for modern AI by introducing basic mechanization and computer-aided processes. These early systems, although rudimentary by today's standards, marked the beginning of a shift towards more efficient production lines.
Significant Milestones and Technological Breakthroughs
One of the most significant milestones in the evolution of AI in manufacturing was the introduction of computer-aided manufacturing (CAM) in the late 20th century. CAM systems allowed for the precise control of machinery, significantly enhancing production capabilities. The advent of the internet and advancements in computing power further accelerated AI's development, paving the way for the integration of AI into production lines. The emergence of smart factories—where interconnected systems work seamlessly together—marked another crucial advancement. These factories leverage AI technologies to optimize every aspect of production, from supply chain management to quality control.
Pioneers and Influential Figures in AI Development
The journey of AI in manufacturing would not have been possible without the contributions of pioneers and influential figures in the field. Innovators like Joseph Engelberger, often regarded as the father of robotics, played a pivotal role in introducing industrial robots to manufacturing. This innovation revolutionized assembly lines, making them faster and more efficient. Similarly, companies such as General Motors and Toyota have been at the forefront of AI adoption, investing heavily in research and development to push the boundaries of what AI can achieve in manufacturing.
Shaping the Manufacturing Landscape
The historical evolution of AI in manufacturing highlights a trajectory of continuous improvement and adaptation. From early automation to the sophisticated AI systems of today, each technological breakthrough has contributed to shaping the modern manufacturing landscape. The journey is far from over, as ongoing research and development efforts continue to drive AI adoption, promising even more transformative changes in the years to come.
Keep Reading
Key technologies and methodologies in ai in manufacturing industries
Exploring Core AI Technologies in Manufacturing
In the realm of manufacturing, several AI technologies stand out for their potential to transform processes and increase efficiency. Predictive maintenance, for example, utilizes machine learning algorithms to analyze data from machinery and predict potential failures before they occur. This proactive approach not only reduces downtime but also extends the life of equipment, offering significant cost savings. Another critical technology is computer vision, which enables machines to "see" and interpret visual information. This capability is invaluable for quality control, where AI systems can detect defects with greater accuracy than human inspectors.
Methodologies Driving AI Adoption
The methodologies underpinning AI technologies in manufacturing are equally important. Deep learning, a subset of machine learning, involves training neural networks to recognize patterns in data, enabling advanced capabilities like image and speech recognition. Neural networks, inspired by the human brain's structure, are instrumental in developing AI systems that can learn and adapt over time. The integration of the Internet of Things (IoT) further enhances AI's potential by connecting devices and systems, allowing for real-time data collection and analysis. IoT integration is particularly beneficial in smart factories, where interconnected systems work together to optimize production.
Evolving Technologies and Industry Implications
The landscape of AI technologies in manufacturing is continuously evolving, with new innovations and advancements emerging regularly. For instance, advancements in natural language processing (NLP) are enabling more intuitive human-machine interactions, facilitating better decision-making and process optimization. As these technologies evolve, their implications for the industry are profound. Manufacturers can achieve unprecedented levels of precision, speed, and customization, allowing them to meet changing consumer demands and remain competitive in a global market.
Continuous Evolution and Industry Impact
The continuous evolution of AI technologies in manufacturing is shaping the industry's future, offering new opportunities and challenges. By staying abreast of technological developments and adopting the right methodologies, manufacturers can harness AI's full potential to drive growth and innovation. As AI technologies continue to mature, their impact on manufacturing will only become more significant, redefining how products are designed, produced, and delivered.
Case studies: ai in manufacturing industries in action
Example 1: Predictive Maintenance at Siemens
Siemens, a global leader in industrial manufacturing, has been at the forefront of AI adoption, particularly in the area of predictive maintenance. By leveraging advanced machine learning algorithms, Siemens has developed systems that analyze data from sensors embedded in machinery to predict maintenance needs. This proactive approach has significantly reduced unexpected equipment failures and downtime, leading to substantial cost savings. For instance, Siemens reported a reduction in maintenance costs by up to 30% and a decrease in unplanned downtime by 20%. These improvements not only enhance operational efficiency but also extend the lifespan of critical machinery, providing a competitive edge in the industry.
Example 2: Autonomous Robotics in Tesla’s Production Line
Tesla, known for its innovation in the automotive industry, has embraced AI-driven robotics to revolutionize its production line. By incorporating autonomous robots equipped with AI capabilities, Tesla has achieved remarkable improvements in manufacturing speed and precision. These robots can perform complex tasks such as assembling car components, painting, and quality inspection with minimal human intervention. The result is a streamlined production process that reduces errors and increases output. Tesla's implementation of AI-driven robotics has not only enhanced production efficiency but also set a new standard for automation in the automotive industry, showcasing the transformative potential of AI technologies.
Example 3: Quality Control with Computer Vision at BMW
BMW, a leading automotive manufacturer, has harnessed the power of AI-based computer vision to enhance quality control processes. By deploying AI systems capable of analyzing images and detecting defects, BMW ensures that only the highest-quality products reach customers. These AI systems can identify even the smallest imperfections that might be overlooked by human inspectors, improving overall product quality and reducing waste. BMW's use of computer vision in quality control exemplifies the effectiveness of AI technologies in enhancing precision and reliability in manufacturing, ultimately leading to improved customer satisfaction and brand reputation.
Keep Reading
Challenges and limitations of ai in manufacturing industries
Data Quality Issues and High Implementation Costs
One of the most significant challenges manufacturers face when implementing AI is ensuring data quality. AI systems require large volumes of high-quality data to function effectively, but data collected from manufacturing processes can often be incomplete, inconsistent, or noisy. Addressing these data quality issues is crucial for accurate AI predictions and outcomes. Additionally, the high costs associated with AI implementation, including technology acquisition, infrastructure upgrades, and workforce training, can be prohibitive for some manufacturers, particularly small and medium-sized enterprises (SMEs).
Lack of Skilled Workforce
The successful implementation of AI in manufacturing also depends on the availability of skilled personnel. The demand for data scientists, AI specialists, and engineers proficient in AI technologies far exceeds the current supply, creating a talent gap. Manufacturers must invest in workforce development, offering training and education programs to equip employees with the necessary skills to work with AI systems. Collaboration with educational institutions and industry partners can help bridge this skills gap and ensure a steady pipeline of talent.
Potential Biases in AI Models
AI systems are only as good as the data they are trained on, and biased data can lead to biased outcomes. This is particularly concerning in manufacturing, where biased AI models can result in suboptimal decisions, affecting product quality and customer satisfaction. Manufacturers need to ensure that data used to train AI models is representative, diverse, and free from biases. Regular audits and reviews of AI systems can help identify and mitigate potential biases, ensuring fair and accurate outcomes.
Integration Hurdles in Legacy Systems
Many manufacturing companies operate with legacy systems that were not designed to integrate with modern AI technologies. This presents a significant hurdle, as these systems may lack the necessary connectivity, interoperability, or data handling capabilities required for AI implementation. Manufacturers need to assess their existing systems and infrastructure and make necessary upgrades or replacements to facilitate AI integration. This process can be complex and costly, requiring strategic planning and resource allocation.
Addressing Ethical Concerns
Ethical concerns related to AI implementation in manufacturing include privacy, job displacement, and decision-making transparency. Manufacturers must navigate these issues carefully, ensuring that AI systems are implemented responsibly and ethically. Establishing clear guidelines and protocols for data privacy, involving stakeholders in decision-making processes, and maintaining transparency in AI operations are crucial steps in addressing ethical concerns and building trust with employees and customers.
Keep Reading
Future of ai in manufacturing industries
Emerging Trends and Innovations
The future of AI in manufacturing is filled with exciting possibilities as emerging trends and innovations promise to reshape the industry. One such trend is AI-driven supply chain optimization, where AI algorithms analyze vast amounts of data to enhance supply chain efficiency, reduce costs, and improve delivery times. The concept of digital twins, virtual replicas of physical assets, is gaining traction, allowing manufacturers to simulate and optimize processes before implementing them in the real world. Adaptive learning systems, which enable AI to continuously learn and improve from new data, are also on the horizon, offering the potential for enhanced decision-making and process optimization.
Predicting AI's Future Impact
The future impact of AI on manufacturing processes, business models, and workforce dynamics is expected to be profound. AI technologies will enable manufacturers to achieve unprecedented levels of precision, speed, and customization, allowing them to meet changing consumer demands and remain competitive in a global market. Business models will evolve as manufacturers leverage AI to create new revenue streams, such as offering AI-driven services or products. As AI systems take on repetitive and mundane tasks, the workforce will need to adapt, with a greater emphasis on creativity, problem-solving, and collaboration.
Ongoing Research and Development Efforts
Ongoing research and development efforts are driving the continuous advancement of AI technologies, pushing the boundaries of what is possible in manufacturing. Researchers are exploring new methodologies, algorithms, and applications to enhance AI capabilities and address current challenges. Industry collaborations and partnerships with academic institutions are fostering innovation and knowledge sharing, accelerating AI adoption and integration. As these efforts continue, the potential for AI to transform manufacturing will only grow, offering new opportunities for manufacturers to enhance efficiency, productivity, and innovation.
Economic and social impact of ai in manufacturing industries
Examining Economic Benefits
The economic impact of AI in manufacturing is significant, offering numerous benefits that extend beyond increased productivity and cost savings. AI technologies enable manufacturers to optimize production processes, reduce waste, and improve product quality, leading to a competitive advantage in the market. According to a report by Accenture, AI could potentially increase labor productivity in manufacturing by up to 40% by 2035, contributing to economic growth and prosperity. Additionally, AI-driven innovations can lead to the development of new products and services, creating new revenue streams and business opportunities.
Discussing Job Displacement vs. Job Creation
The introduction of AI in manufacturing has sparked debates about its impact on employment, with concerns about job displacement and automation replacing human workers. While it is true that AI can automate certain tasks, it also creates opportunities for job creation and workforce transformation. As AI takes over repetitive and mundane tasks, the demand for skilled workers in areas such as data analysis, AI system management, and complex problem-solving will increase. Manufacturers must invest in workforce reskilling and upskilling to equip employees with the skills needed to thrive in an AI-driven environment, ensuring a smooth transition and minimizing job displacement.
Analyzing Broader Social Implications
The social implications of AI in manufacturing extend beyond the workplace, impacting broader societal dynamics. AI technologies have the potential to democratize access to advanced manufacturing capabilities, enabling smaller companies and startups to compete with larger players. This democratization of technology can drive innovation and economic growth, fostering a more inclusive and competitive industry. However, it also raises concerns about data privacy, ethical considerations, and the balance between human and machine control. Manufacturers must navigate these social implications carefully, ensuring that AI technologies are deployed responsibly and ethically to maximize their benefits while minimizing potential risks.
Keep Reading
Regulatory and ethical considerations of ai in manufacturing industries
Overview of the Regulatory Environment
The regulatory environment governing AI use in manufacturing is complex and evolving, as policymakers strive to balance innovation with safety and compliance. Manufacturers must adhere to a range of regulations and standards related to AI implementation, data privacy, and safety protocols. Compliance with these regulations is essential to ensure that AI systems are deployed responsibly and ethically, minimizing potential risks and liabilities. Engaging with regulatory bodies and industry associations can help manufacturers stay informed about evolving requirements and ensure compliance.
Highlighting Ethical Dilemmas
The deployment of AI in manufacturing raises several ethical dilemmas that manufacturers must address. One such dilemma is AI accountability, as the complexity of AI systems can make it challenging to determine responsibility for decisions and outcomes. Data privacy is another critical concern, as AI systems rely on vast amounts of data that must be collected, stored, and processed securely. Manufacturers must also consider the balance between human and machine control, ensuring that AI systems are designed to complement human capabilities rather than replace them. Establishing clear ethical guidelines and protocols is essential to navigate these dilemmas responsibly.
Strategies for Responsible AI Deployment
Ensuring responsible AI deployment in manufacturing requires a multifaceted approach that involves stakeholder engagement, transparent communication, and adherence to ethical guidelines. Manufacturers should involve diverse stakeholders, including employees, customers, regulators, and industry experts, in the decision-making process to ensure that AI systems align with ethical values and societal expectations. Transparency in AI operations, including providing clear explanations of AI decision-making processes, can build trust and confidence. Additionally, manufacturers should establish ethical guidelines and protocols that prioritize data privacy, accountability, and fairness, ensuring that AI systems are deployed in a manner that maximizes benefits while minimizing potential risks.
Conclusion
The transformative potential of AI in manufacturing is undeniable, offering unprecedented opportunities for innovation, efficiency, and productivity. Throughout this article, we have explored the impact of AI on manufacturing processes, highlighted key technologies and methodologies, and examined real-world case studies from industry leaders. We have also discussed the challenges and limitations manufacturers face when implementing AI, as well as the ethical and regulatory considerations that must be addressed. Looking ahead, the future of AI in manufacturing is promising, with emerging trends and innovations set to redefine the industry. To fully harness AI's potential, manufacturers must navigate challenges responsibly, invest in workforce development, and prioritize ethical deployment. By doing so, they can leverage AI to drive growth, enhance competitiveness, and shape the future of manufacturing.
Keep Reading
Do's and don'ts of ai implementation in manufacturing
Do's | Don'ts |
---|---|
Invest in quality data and infrastructure. | Ignore the importance of workforce training. |
Start with pilot projects to test AI applications. | Overlook ethical considerations and regulations. |
Continuously monitor and optimize AI systems. | Rely entirely on AI without human oversight. |
Foster a culture of innovation and learning. | Underestimate the costs and challenges involved. |
This comprehensive article provides a deep dive into the role and impact of AI in manufacturing industries, offering valuable insights and guidance for professionals and project managers. By understanding the potential and challenges of AI technologies, manufacturers can strategically leverage AI to drive growth and innovation, shaping the future of the industry.
Build powerful workflows with Meegle for free!
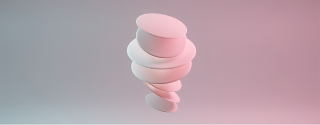
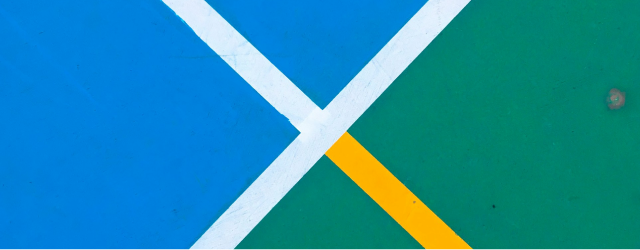
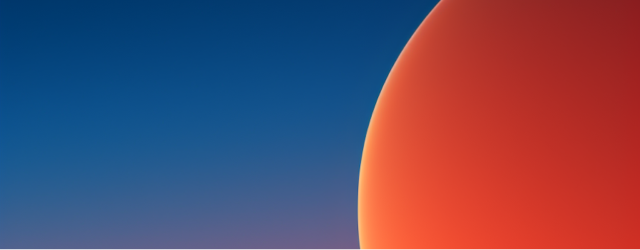
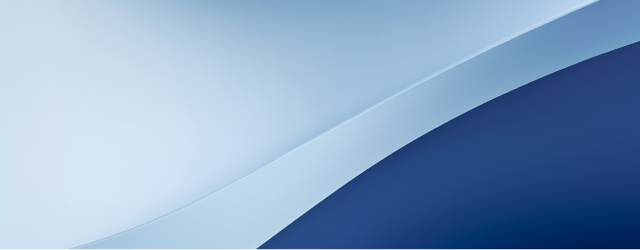
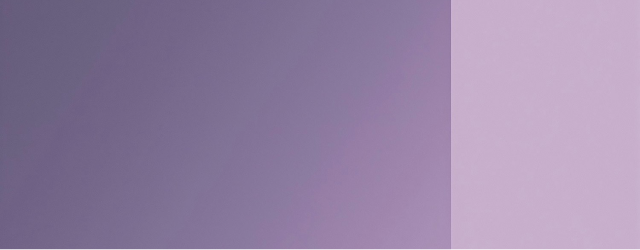
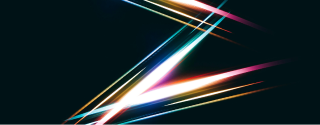
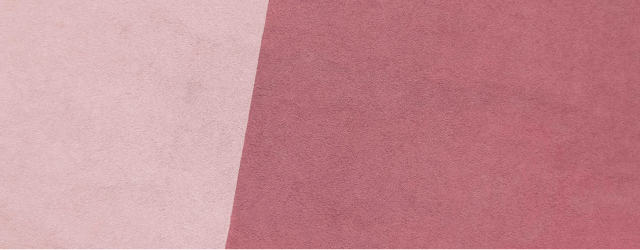
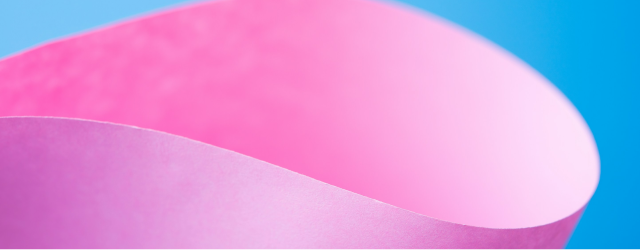