AI In Healthcare
Explore the foundations, applications, and future of AI in Healthcare, illustrating its significant impact on technology and industry. Deep dive into real-world case studies and emerging trends.
Artificial Intelligence (AI) in healthcare refers to the use of complex algorithms and software to emulate human cognition in the analysis, interpretation, and comprehension of complicated medical and healthcare data. Specifically, AI is adept at recognizing patterns and making decisions based on data input, making it a valuable tool in diagnostics, patient care, and data management in healthcare.
The importance of AI in today's healthcare industry cannot be overstated. With its ability to analyze large amounts of data quickly and accurately, AI can enhance diagnostic precision, predict patient outcomes, and improve overall patient care. From automated reminders for medication to predicting the likelihood of chronic diseases, AI is steadily transforming the healthcare landscape.
Build powerful workflows with Meegle for free!
Tracing the trajectory: the evolution of ai in healthcare
AI has come a long way since its inception, and its journey in healthcare is equally fascinating. From rudimentary applications in the mid-20th century, such as basic machine learning algorithms and decision-making systems, AI has evolved into sophisticated neural networks capable of mimicking human thought processes.
Some significant milestones include the development of expert systems in the 1970s, such as MYCIN and DENDRAL, which marked the beginning of AI's integration into healthcare. The advent of machine learning and deep learning methodologies in the 21st century has further propelled AI's capabilities in health diagnostics, predictive analytics, and personalized medicine.
Keep Reading
Behind the scenes: technologies and methodologies powering ai in healthcare
AI in healthcare is driven by a range of technologies and methodologies. Machine learning, a subset of AI, enables computers to learn from and interpret data without explicit programming. Deep learning, a further subset of machine learning, uses neural networks with many layers (deep structures) to learn complex patterns in large amounts of data.
Specific AI technologies used in healthcare include natural language processing (used in patient-health record data extraction), computer vision (used in radiology imaging), and predictive analytics (used in disease prediction and prevention). As technology progresses, AI tools are becoming more capable, adaptable, and scalable in healthcare.
In the field: ai in healthcare case studies
Let's consider some real-world examples of AI in healthcare. PathAI, a Boston-based company, uses AI to improve the accuracy of pathology diagnoses. Their system assists pathologists in identifying minute changes in tissue samples that can indicate the early onset of diseases like cancer.
On another front, Google's DeepMind developed an AI system that can identify over 50 eye diseases as accurately as a trained doctor. It analyses 3D scans of the eye, providing referrals that match the recommendations of world-leading expert clinicians.
Keep Reading
Navigating the terrain: challenges and limitations of ai in healthcare
Despite its potential, AI in healthcare faces several challenges. These include data privacy concerns, lack of standardized regulations, and potential job displacement among health workers due to automation.
AI faces ethical issues as well, such as the risk of biases in AI algorithms that could lead to unfair patient treatment. There are also concerns about the lack of transparency or 'explainability' of AI decisions, which makes it difficult for humans to understand or challenge these decisions.
Predicting the unpredictable: the future of ai in healthcare
AI holds immense potential for the future of healthcare. With advancements in technology, we can expect AI to become an integral part of disease detection, diagnosis, and treatment. AI might also enable personalized medicine at an unprecedented scale, tailoring treatments to individual patients' genetic makeup and lifestyle habits.
However, the future of AI also brings uncertainties. As AI continues to evolve and integrate itself into healthcare, it will be crucial for stakeholders to navigate the challenges, optimize its benefits, and mitigate its potential downsides.
Keep Reading
Measuring the change: economic and social impact of ai in healthcare
The integration of AI into healthcare has significant economic and social implications. Economically, AI has the potential to save the healthcare industry billions of dollars by reducing unnecessary hospital visits, increasing the efficiency of administrative tasks, and improving the accuracy of diagnoses.
Socially, while AI could potentially replace some jobs in healthcare, it could also create new roles that require a blend of medical knowledge and technical skills. Furthermore, the increased efficiency brought about by AI could free up more time for healthcare professionals to spend on patient care.
Treading carefully: regulatory and ethical considerations in ai in healthcare
As AI continues to permeate the healthcare sector, it is imperative to consider regulatory and ethical considerations. These include data privacy and security, informed consent, liability for AI decisions, and the potential for algorithmic bias.
A robust regulatory framework is needed to ensure that AI is used responsibly in healthcare. This includes clear guidelines for data sharing, strict data privacy and security measures, and regulations to ensure that AI systems are transparent and accountable.
Keep Reading
Summing up: the road ahead for ai in healthcare
The journey of AI in healthcare is only beginning. As technology advances, AI's applications and influence in healthcare will continue to grow. However, the path forward is not without challenges. It will be crucial for healthcare professionals and policymakers to navigate these challenges strategically to harness AI's full potential in healthcare.
Step-by-step guide: implementing ai in healthcare
Implementing AI in healthcare involves several steps. These include understanding the basics of AI, identifying opportunities for AI implementation, choosing the right technologies, overcoming challenges associated with AI adoption, and evaluating the success of AI initiatives.
Keep Reading
Do’s and don’ts when implementing ai in healthcare
Implementing AI in healthcare requires careful planning and execution. Here are some guidelines to consider during this process:
- Do conduct a thorough assessment of your organization's readiness for AI adoption.
- Don't ignore the ethical and regulatory considerations associated with AI in healthcare.
- Do provide adequate training for healthcare professionals who will be using AI tools.
Frequently asked questions (faqs) on ai in healthcare
There are several common questions about AI in healthcare. These include understanding what AI is, how it's being used in healthcare, examples of AI applications in healthcare, the challenges associated with implementing AI in healthcare, and the future of AI in healthcare. In the subsequent sections, we will delve into these questions and provide comprehensive answers.
Build powerful workflows with Meegle for free!
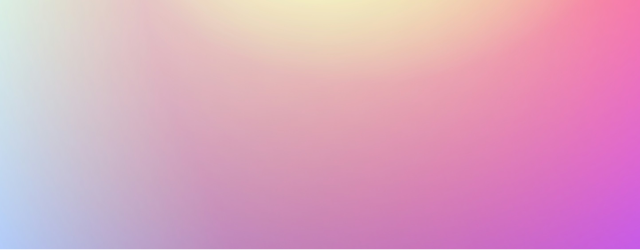
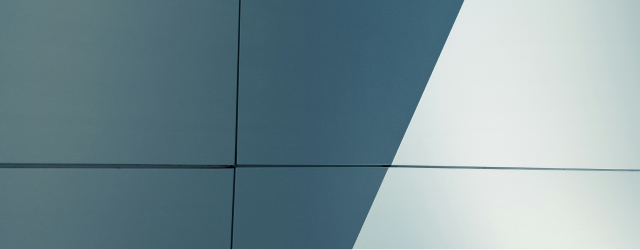
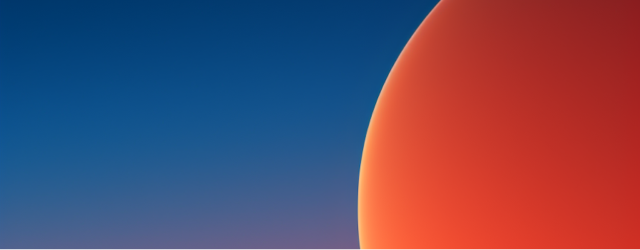
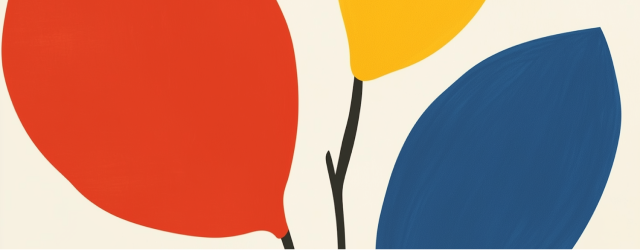
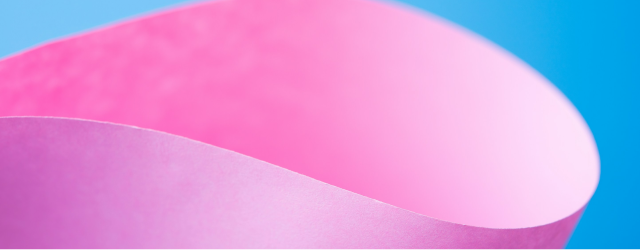
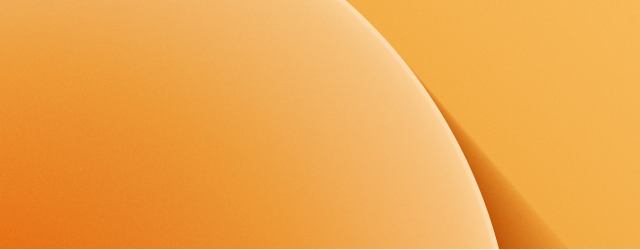
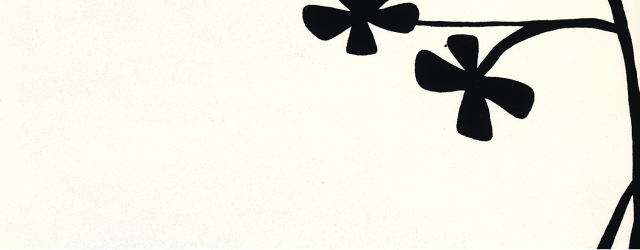
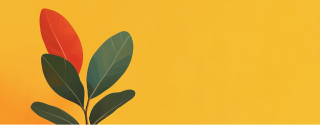