Deep Learning Vs. Machine Learning
Explore the foundations, applications, and future of Deep Learning vs. Machine Learning, illustrating its significant impact on technology and industry. Deep dive into real-world case studies and emerging trends.
Deep Learning and Machine Learning are subsets of artificial intelligence (AI), an expansive field that aims to emulate human intelligence in machines. But, they are different in their approach and complexity. Machine Learning enables computers to learn from and interpret data, adjusting their operations accordingly without being explicitly programmed to perform specific tasks. On the other hand, Deep Learning, a more complex and sophisticated subset of Machine Learning, uses artificial neural networks to simulate human decision-making and learning capabilities.
Build powerful workflows with Meegle for free!
Historical progression of deep learning and machine learning
Deep Learning and Machine Learning have their roots in the mid-20th century when the pioneers of computing began to explore how machines could learn from data. The 'Perceptron,' a machine capable of 'learning,' was the first significant milestone, marking the birth of Machine Learning. The development of the 'Backpropagation' algorithm in the 1970s enabled more efficient training of these models, laying the foundation for Deep Learning. The evolution of these technologies has been fueled by advancements in computer processing capabilities and the proliferation of big data.
Keep Reading
Core technologies and methodologies in deep learning and machine learning
Machine Learning uses various algorithms, including regression, decision trees, and clustering, to make predictions or decisions without being explicitly programmed to perform the task. Deep Learning, on the other hand, employs artificial neural networks, specifically deep neural networks, to interpret data and make decisions. These networks are composed of multiple layers of nodes or 'neurons,' each performing a specific task and contributing to the network's overall output.
Case studies: deep learning and machine learning in practice
Real-world applications of Deep Learning and Machine Learning are vast and varied, spanning sectors such as healthcare, finance, and transportation. For instance, Deep Learning is used in medical imaging to detect diseases, while Machine Learning algorithms power financial forecasting models. These practical applications underscore the transformative potential of these technologies.
Keep Reading
Challenges and constraints of deep learning and machine learning
Despite their potential, the implementation of Deep Learning and Machine Learning is not without challenges. These range from the technical – such as the need for large amounts of data and computational power – to ethical concerns about privacy and accountability. Furthermore, there is a need for a robust regulatory framework to govern the use of these technologies and mitigate their potential risks.
Future prospects of deep learning and machine learning
With advancements in technology and a growing understanding of their potential, Deep Learning and Machine Learning are poised to revolutionize various sectors. Emerging trends include the integration of these technologies into edge devices and the development of explainable AI models that offer greater transparency in decision-making.
Socio-economic impact of deep learning and machine learning
The economic benefits of Deep Learning and Machine Learning are significant, with potential applications in sectors such as healthcare and finance poised to generate substantial cost savings. However, there are concerns about job displacement due to automation. Moreover, the social implications of these technologies – including their potential impact on privacy and the digital divide – warrant careful consideration.
Regulatory and ethical dynamics of deep learning and machine learning
The rise of Deep Learning and Machine Learning has sparked a need for a comprehensive regulatory framework. This need is complicated by the global nature of these technologies and the lack of a shared understanding of their ethical implications. Key issues include data privacy, algorithmic accountability, and the potential for bias in AI systems.
Conclusion
Deep Learning and Machine Learning hold immense potential to revolutionize various sectors and transform our interaction with the digital world. However, realizing this potential will require addressing a range of technical, ethical, and regulatory challenges. As we continue to navigate the AI era, it is crucial to understand these technologies' complexities, impacts, and implications for the future.
Build powerful workflows with Meegle for free!
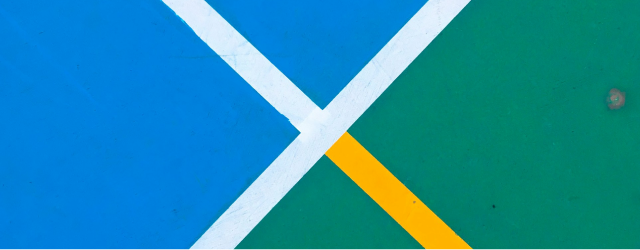
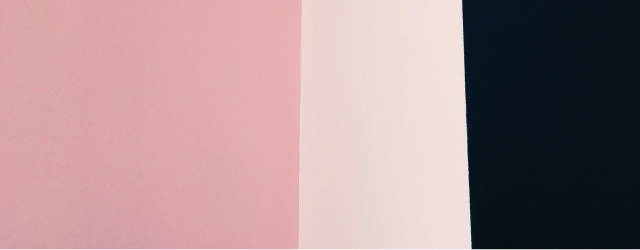
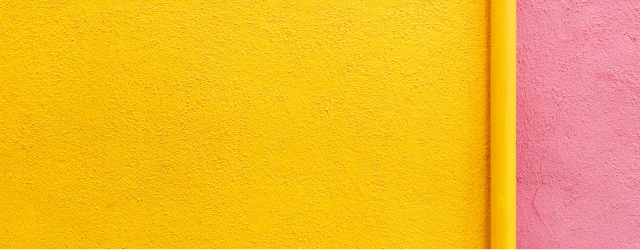
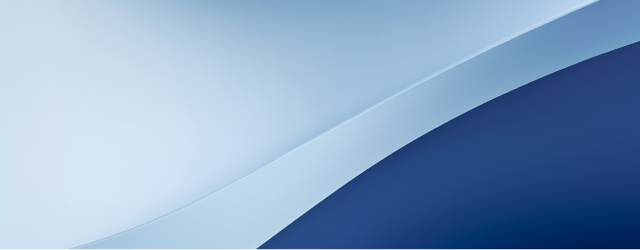
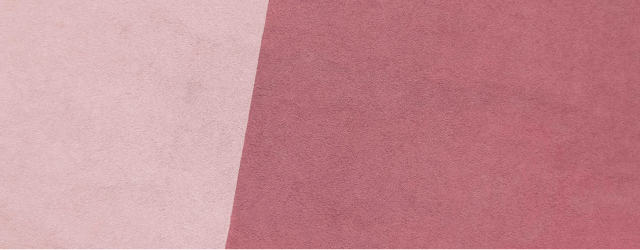
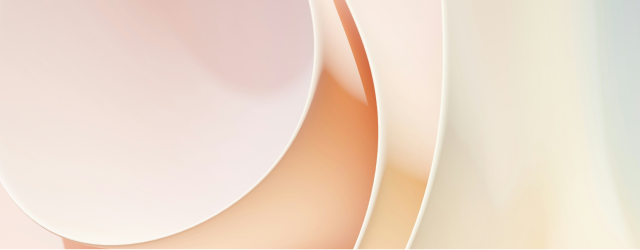
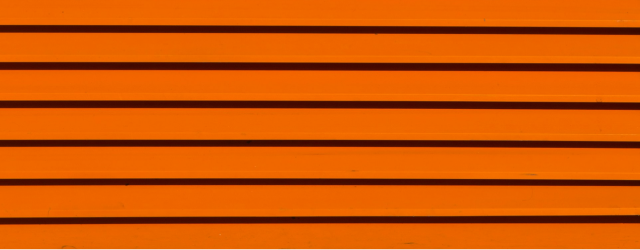