Federated Learning
Explore the foundations, applications, and future of Federated Learning, illustrating its significant impact on technology and industry. Deep dive into real-world case studies and emerging trends.
Federated Learning is a groundbreaking technique in machine learning that enables devices to learn from data while maintaining data privacy. It operates on the principle of distributed learning, where models are trained across multiple decentralized devices or servers holding local data samples, without exchanging them. This innovative approach has significant implications in the tech industry, particularly in the era of Big Data and Internet of Things (IoT), where data privacy regulations and concerns are paramount.
In the current tech landscape, Federated Learning stands as a beacon of hope, promising to advance machine learning algorithms without compromising on data privacy. It's a mutually beneficial solution that allows tech companies to improve their products and services using machine learning, while ensuring the individuals' data is not exposed or misused, thus maintaining trust and compliance with data privacy regulations.
Build powerful workflows with Meegle for free!
The journey of federated learning: a historical perspective
Although Federated Learning may seem like a recent development, its roots can be traced back to the late 1990s. The concept evolved through the years, hand in hand with advancements in machine learning and the growing need for privacy-preserving methods of data analysis.
One of the significant milestones in the journey of Federated Learning was the introduction of the concept by Google in 2016. This was a significant shift from traditional cloud-based machine learning methods, offering a solution that prioritizes both learning efficiency and data privacy. Google's Gboard, the virtual keyboard app, was one of the first real-world applications of Federated Learning.
Unraveling the technicalities: key technologies and methodologies in federated learning
Federated Learning employs various technologies and methodologies to achieve its goal of decentralized learning. The primary technology behind Federated Learning is the use of local data and local models. Each participating device in the Federated Learning network has a local model that learns from the local data, which means the data does not need to be sent to a central server.
The evolution of these technologies has a profound impact on the field. For example, advancements in edge computing and decentralized networks have significantly improved the efficiency and feasibility of Federated Learning. These technologies have enabled faster learning, better model performance, and greater privacy preservation.
Keep Reading
Federated learning in the real world: case studies
One concrete example of Federated Learning in action is Google's Gboard. Gboard uses Federated Learning to improve its predictive text functionality without sending your typing data to Google. Instead, the learning happens locally on your device, and only the model updates are shared with Google, not what you typed.
Another case study comes from the healthcare industry. Owkin, a startup focused on AI and medicine, uses Federated Learning to train AI models on data from multiple hospitals while keeping the data within the original institution. This approach not only preserves data privacy but also allows the application of AI in medicine to access more diverse datasets, improving the accuracy and relevance of the models.
The roadblocks: challenges and limitations of federated learning
As with any emerging technology, Federated Learning also faces its share of challenges and limitations. These include issues related to communication overhead, system heterogeneity, and model personalization.
From an ethical perspective, although Federated Learning strengthens data privacy, it also raises new questions about data ownership and control. For instance, who owns the models trained by Federated Learning? How can misuse of these models be prevented? These are some of the ethical dilemmas that technology stakeholders need to address.
Keep Reading
Looking ahead: the future of federated learning
The future of Federated Learning looks promising. It is anticipated to play a significant role in the development of AI applications across various industries, from healthcare to finance, and from education to transportation.
Moreover, advancements in technologies like 5G and edge computing are expected to further enhance the efficiency and applications of Federated Learning. As these technologies mature, they will create a more conducive environment for Federated Learning, potentially leading to its widespread adoption.
Impacts beyond technology: economic and social implications of federated learning
Federated Learning not only has technological implications but also economic and social ones. It can potentially lead to job creation in the tech industry as companies seek experts in this field. However, it could also lead to job displacement as machine learning becomes more efficient and automated.
On the social front, Federated Learning can help build public trust in tech companies by showing their commitment to data privacy. However, the technology also needs to be used responsibly to avoid misuse and potential backlash.
Keep Reading
Navigating the legal terrain: regulatory and ethical considerations of federated learning
From a legal perspective, Federated Learning falls under the broader umbrella of data privacy regulations. Since it does not involve data sharing, it can help companies comply with data privacy laws.
However, Federated Learning also raises new ethical considerations. For instance, how to ensure the models trained by Federated Learning are used ethically? This is a developing area of research and policy-making, and tech companies need to stay abreast of these considerations as they implement Federated Learning.
In a nutshell: conclusion
Federated Learning presents a promising way forward for machine learning and data privacy. It offers a mutually beneficial solution for tech companies and individuals, and its applications are wide-ranging and impactful. As we look towards a future where data privacy and machine learning go hand in hand, Federated Learning will undoubtedly play a pivotal role.
Related:
Lead to Cash TemplateKeep Reading
Faqs on federated learning
What is Federated Learning?
Federated Learning is a technique in machine learning that enables devices to learn from data while maintaining data privacy. It operates on the principle of distributed learning, where models are trained across multiple decentralized devices or servers holding local data samples, without exchanging them.
How does Federated Learning work?
Federated Learning works by using local data and local models. Each participating device in the Federated Learning network has a local model that learns from the local data, which means the data does not need to be sent to a central server. The learning happens locally on the device, and only the model updates are shared, not the data itself.
How is Federated Learning implemented in different industries?
Federated Learning is implemented in various industries such as technology, healthcare, finance, and education. For example, Google's Gboard uses Federated Learning to improve its predictive text functionality, and the healthcare startup Owkin uses it to train AI models on data from multiple hospitals while keeping the data within the original institution.
What are the challenges in developing and implementing Federated Learning?
Challenges in developing and implementing Federated Learning include communication overhead, system heterogeneity, and model personalization. Ethical and legal considerations also pose challenges, such as questions about data ownership and control, and ensuring the responsible use of the models trained by Federated Learning.
What is the future of Federated Learning?
The future of Federated Learning is expected to be influential in the development of AI applications across various industries. Advancements in technologies like 5G and edge computing are expected to enhance its efficiency and applications further. However, the technology's successful future also depends on navigating its challenges and ethical considerations effectively.
Build powerful workflows with Meegle for free!
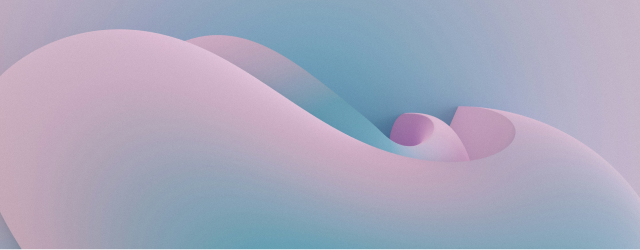
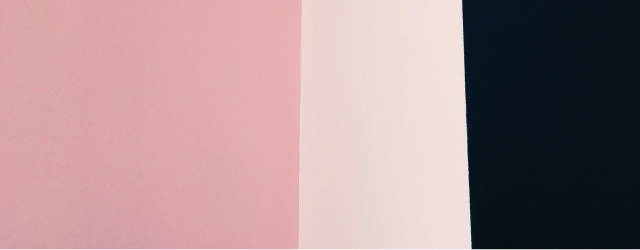
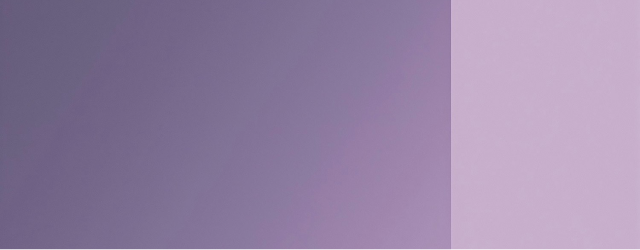
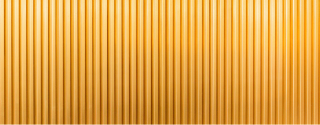
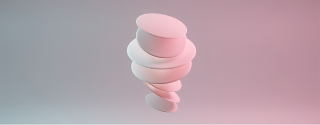
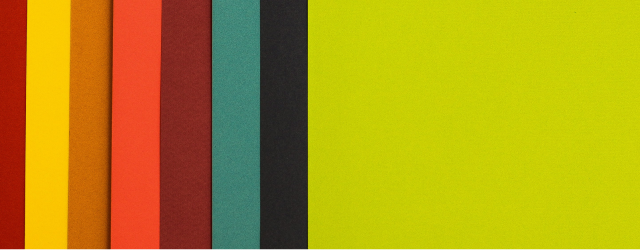
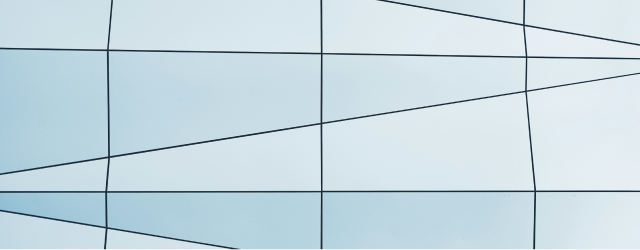