Generative Adversarial Networks (GANs)
Explore the foundations, applications, and future of Generative Adversarial Networks (GANs), illustrating its significant impact on technology and industry. Deep dive into real-world case studies and emerging trends.
Build powerful workflows with Meegle for free!
Unraveling the basics of generative adversarial networks (gans)
When you delve into the sphere of Artificial Intelligence (AI), you'll encounter Generative Adversarial Networks (GANs), a technology that has become a significant player in the AI landscape. At its core, GANs are a class of machine learning systems invented by Ian Goodfellow in 2014. It consists of two parts, namely, the "generator," which produces data instances, and the "discriminator," which evaluates them for authenticity. The interplay between these two components enables the creation of new, synthetic instances of data that can pass for real data.
An illustrative example of GANs at work would be creating a 'fake' image of a cat that looks as realistic as real cat photos. This 'forgery' of data is, in fact, a key feature of GANs and also where its power of innovation lies. By generating lifelike, synthetic data, GANs can be harnessed to create diverse applications, from generating artwork to simulating realistic video game environments.
Tracing the historical journey of generative adversarial networks (gans)
The inception of GANs dates back to 2014 when they were introduced by Ian Goodfellow and his colleagues from the University of Montreal as a novel approach to unsupervised learning. Goodfellow's original idea was sparked by a friendly debate at a bar about the possibility of machines generating new data.
The subsequent years marked significant advances in GANs, with the emergence of variants like Deep Convolutional GANs (DCGANs), CycleGANs, and Conditional GANs, each with its unique capabilities. For instance, DCGANs are known for creating sharp, high-quality images, while CycleGANs can translate images from one domain to another without paired data.
This historical account of GANs is not just about understanding its development but also about appreciating its potential to continuously evolve and revolutionize the AI landscape.
Unpacking the key methodologies and technologies in generative adversarial networks (gans)
GANs operate on a unique mechanism that sets them apart from other machine learning algorithms. They involve two main components: a generator and a discriminator, which work in opposition to each other. The generator creates fake data to pass off as real, while the discriminator tries to detect the forgery.
This adversarial process is akin to a forger trying to create a counterfeit painting and an art investigator trying to catch the forger in the act. The generator and discriminator are each a neural network, and they train together. The generator learns to produce more realistic data while the discriminator becomes better at distinguishing the bogus from the genuine.
To illustrate, consider the Super Resolution GAN (SRGAN). It employs GANs to convert low-resolution images into high-resolution versions. The generator up-scales the image, while the discriminator determines whether the resulting image is a high-resolution original or a up-scaled version.
Keep Reading
Case studies: witnessing generative adversarial networks (gans) at work
GANs' ability to generate realistic synthetic data has found applications in varied sectors. Let's explore some case studies to understand their practical implications better.
In the healthcare sector, GANs are being used to create synthetic medical images for research. With patient data privacy being a significant concern, the synthetic data generated by GANs helps overcome this challenge. For instance, NVIDIA's GAN called GANs for Medical Imaging (GANMI) is used to generate synthetic brain MRI images.
In the field of art, GANs have been used to create new pieces of artwork. An example is the "Portrait of Edmond de Belamy," a painting created by a GAN and sold at an auction for over $400,000. This artwork was generated by a GAN trained on a dataset of historical portraits.
Discussing the challenges and limitations of generative adversarial networks (gans)
While GANs are innovative, they're not without their challenges and limitations. The training process is often unstable, and there's no definitive metric to evaluate their performance.
GANs also face ethical issues. Their ability to generate realistic 'fake' data raises concerns about potential misuse, such as creating deepfakes for spreading misinformation. The development of deepfakes, which are realistic AI-generated videos of people, has sparked debates on data authenticity and consent.
Another challenge is the 'mode collapse' where the generator starts producing limited varieties of samples, thereby reducing the diversity of generated data. The discriminator can also become too powerful, causing the generator to fail in producing realistic data.
Keep Reading
Envisioning the future of generative adversarial networks (gans)
The future of GANs holds immense possibilities. With ongoing research and development, GANs are expected to become more stable and efficient, making them suitable for a wider range of applications. They could be used for generating synthetic data in fields where data is scarce or hard to collect, like space exploration or deep-sea studies.
Furthermore, the advancements in GANs could lead to more sophisticated deepfake detection tools, helping combat the misuse of this technology. As we continue to navigate the potentials and pitfalls of GANs, it's evident that they'll play a crucial role in shaping the future of AI.
Analyzing the economic and social impact of generative adversarial networks (gans)
GANs' economic and social impacts are diverse. Economically, they can drive cost-efficiency by generating synthetic data for training AI models, reducing the need for expensive data collection and labeling.
However, as with any disruptive technology, GANs could also lead to job displacement. Roles that involve data collection could become redundant with the availability of synthetic data. Yet, they could also create new jobs, such as monitoring and managing AI systems.
Socially, GANs can revolutionize content creation in entertainment and gaming, enhancing user experiences. On the other hand, their potential misuse in creating deepfakes raises serious social concerns around privacy and misinformation.
Keep Reading
Navigating the regulatory and ethical terrain of generative adversarial networks (gans)
The regulatory landscape for GANs is still developing. The use of deepfakes, a significant application of GANs, has already drawn regulatory attention. Several countries are considering laws against the malicious use of deepfakes.
GANs also present ethical dilemmas. They challenge concepts of authenticity and intellectual property. If a GAN creates a piece of artwork, who owns it? These are some of the questions that will need to be addressed as we continue to integrate GANs into our society.
Wrapping up: the journey and impact of generative adversarial networks (gans)
In conclusion, GANs have come a long way since their inception in 2014. Despite challenges and limitations, their ability to generate realistic synthetic data has opened up new avenues in AI and beyond. As we continue to explore this technology, we must navigate the complex ethical and regulatory terrain it presents. The journey of GANs is a testament to the transformative power of AI, and we can expect them to continue to shape our future in ways yet unimagined.
Keep Reading
Build powerful workflows with Meegle for free!
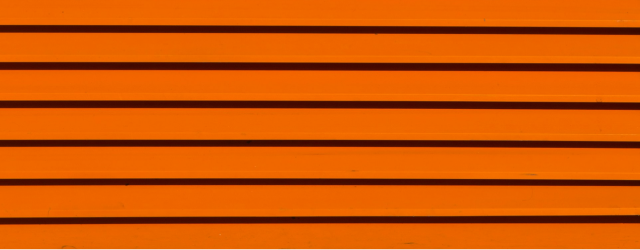
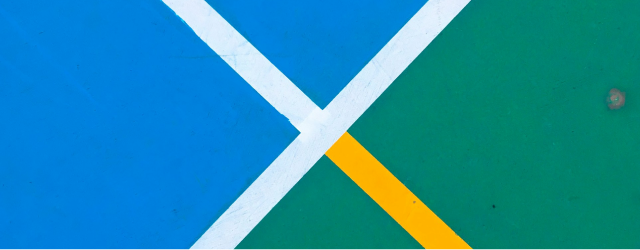
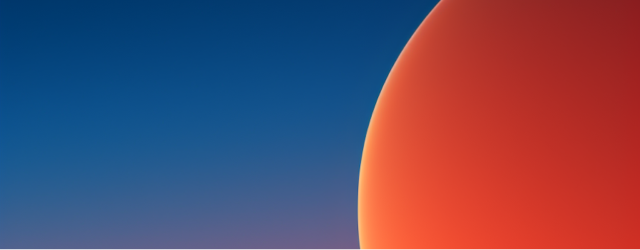
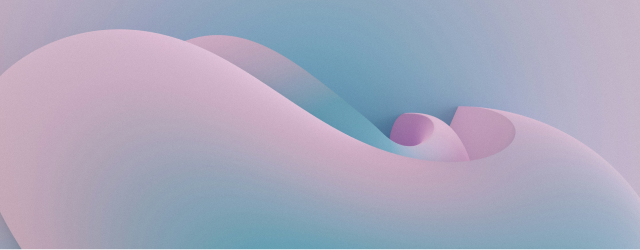
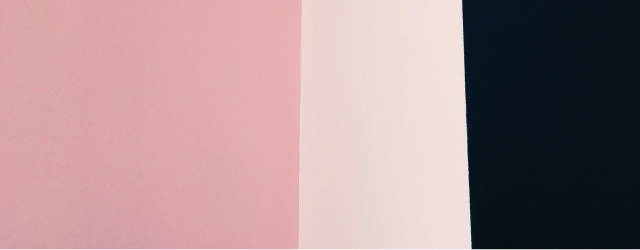
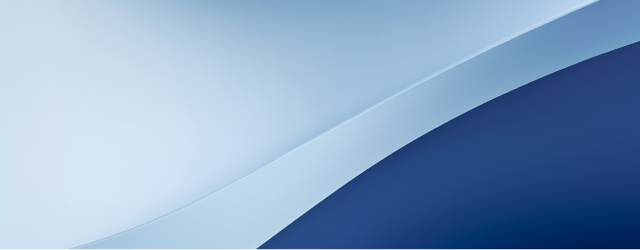
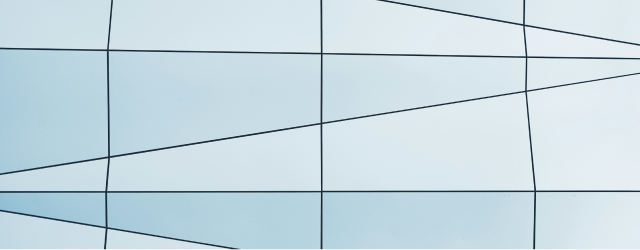
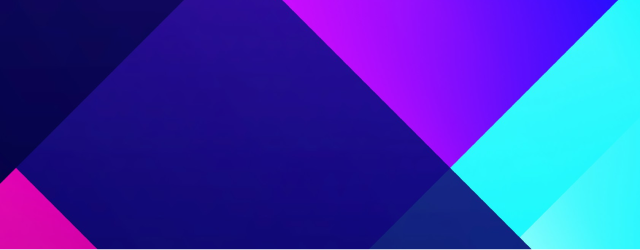