Predictive Analytics In AI
Explore the foundations, applications, and future of Predictive Analytics in AI, illustrating its significant impact on technology and industry. Deep dive into real-world case studies and emerging trends.
Predictive Analytics in AI refers to the use of data, statistical algorithms, and AI techniques to predict future outcomes based on historical data. It's a category of data analytics aimed at making predictions about future outcomes based on historical data and analytics techniques such as statistical modeling and machine learning. The central element of predictive analytics is the predictor, a variable that can be measured for an individual or entity to predict future behavior.
For instance, an insurance company might use predictive analytics to determine the likelihood of a claim being fraudulent. By analyzing past data, the AI can identify patterns and trends that might suggest fraudulent activity.
Build powerful workflows with Meegle for free!
The evolution of predictive analytics in ai: a historical perspective
The origin of Predictive Analytics in AI traces back to the advent of computers. Early attempts were primitive, heavily relying on basic statistical algorithms. However, the exponential growth of computational power and data availability propelled the evolution of predictive analytics.
One significant milestone was the development of machine learning algorithms. These algorithms allowed computers to learn from data, significantly improving the accuracy of predictions. With the advent of deep learning, predictive analytics entered a new era, where AI could make predictions with unprecedented accuracy.
Keep Reading
Exploring the core technologies and methodologies of predictive analytics in ai
The power of predictive analytics lies in its blend of several technologies and methodologies. These include statistical modeling, machine learning, data mining, and AI. Each plays a critical role in harnessing the power of data to make accurate predictions.
Statistical modeling provides the foundation, enabling the identification of patterns in data. Machine learning takes this a step further, allowing AI to learn from the data. Data mining techniques help to discover hidden patterns, while AI brings it all together, making sense of the data and generating predictions.
Success stories: how predictive analytics in ai is making an impact
The impact of Predictive Analytics in AI is already evident in several sectors. For instance, in healthcare, AI is used to predict disease outbreaks. By analyzing data from various sources, AI can identify patterns and trends that indicate an impending outbreak, enabling preventative measures.
In retail, AI is transforming the shopping experience. For instance, Amazon uses predictive analytics to anticipate customer needs and preferences, offering personalized recommendations. This not only improves customer satisfaction but also drives revenue growth.
Keep Reading
Challenges and limitations: navigating the complexities of predictive analytics in ai
Despite its potential, Predictive Analytics in AI is not without its challenges. One major hurdle is data quality. For AI to make accurate predictions, it needs high-quality data. However, data can often be incomplete, inconsistent, or biased, affecting the accuracy of the predictions.
Additionally, there are ethical concerns. AI decisions based on predictive analytics can impact people's lives, raising questions about accountability and fairness. For instance, if an AI system denies a loan application based on its prediction, who is responsible if the prediction is wrong?
Looking ahead: the future of predictive analytics in ai
Predictive Analytics in AI is poised for significant growth in the coming years. With advances in technology and more data becoming available, AI's predictive capabilities will only improve.
One emerging trend is the use of AI in predictive maintenance. By analyzing data from machinery, AI can predict when a breakdown is likely to occur, enabling timely intervention and preventing costly downtime.
Related:
Rapid Application DevelopmentKeep Reading
Assessing the economic and social implications of predictive analytics in ai
The rise of Predictive Analytics in AI has far-reaching economic and social implications. Economically, it promises to drive efficiency and productivity, leading to job creation in sectors such as data analysis and AI development. However, it could also lead to job displacement in areas rendered obsolete by AI.
Socially, while Predictive Analytics in AI can make life more convenient, it also raises privacy concerns. As AI becomes more integrated into our lives, issues around data collection and use will become increasingly prominent.
Navigating the regulatory landscape and ethical dilemmas of predictive analytics in ai
As Predictive Analytics in AI becomes more prevalent, it's critical to navigate the regulatory landscape effectively. In many jurisdictions, laws and regulations governing AI and data use are still evolving, creating a complex legal environment.
Ethically, the use of Predictive Analytics in AI raises numerous dilemmas. For instance, how can we ensure AI decisions based on predictions are fair and unbiased? How do we balance the benefits of predictive analytics with the need to protect privacy?
Wrapping up: the transformative potential of predictive analytics in ai
Predictive Analytics in AI holds immense potential. By harnessing the power of data, AI can make predictions with unparalleled accuracy, driving innovation across countless sectors. However, realizing this potential requires navigating complex challenges, from data quality issues to ethical dilemmas.
Build powerful workflows with Meegle for free!
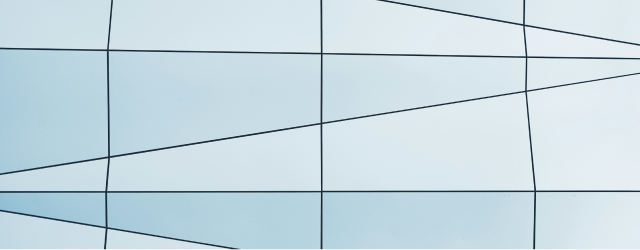
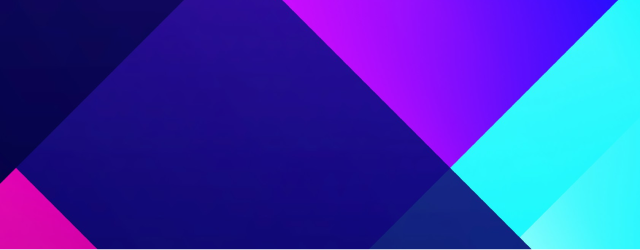
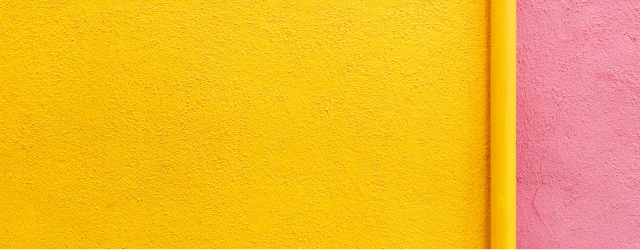
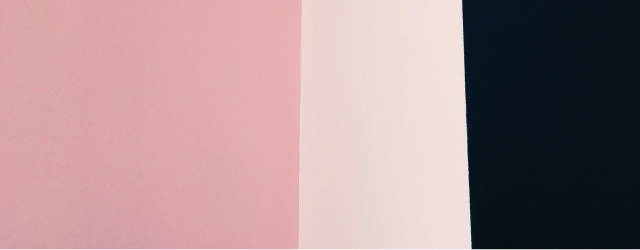
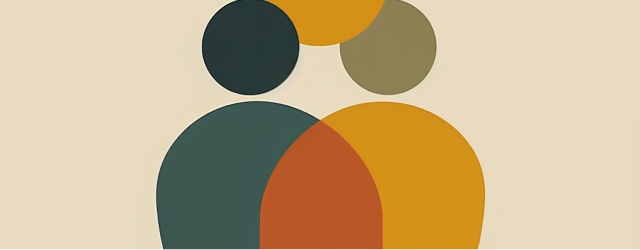
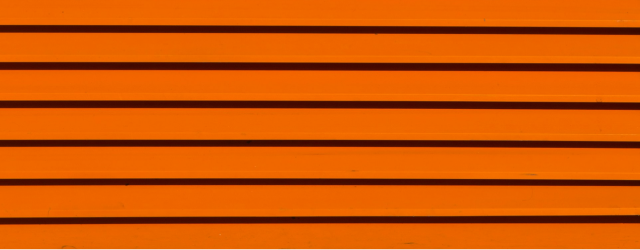
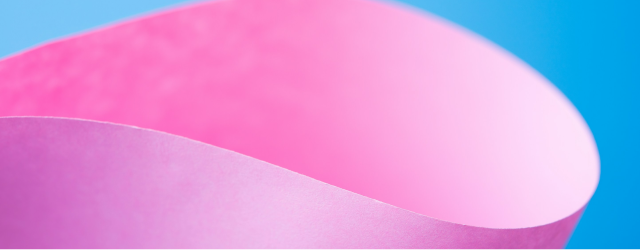
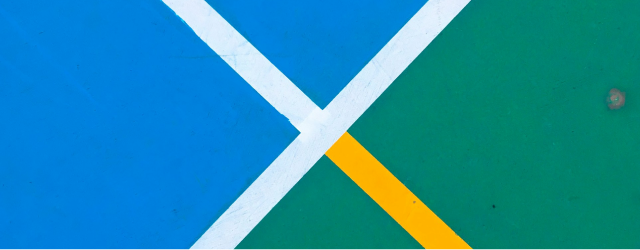