Reinforcement Learning
Explore the foundations, applications, and future of Reinforcement Learning, illustrating its significant impact on technology and industry. Deep dive into real-world case studies and emerging trends.
Reinforcement Learning (RL) is a branch of machine learning that involves an agent learning how to behave in an environment by performing actions and receiving rewards or penalties. The agent learns to make decisions by trying different actions and observing the results, gradually improving its performance over time.
In the current technological landscape, RL holds significant value for its ability to automate complex decision-making processes. This can lead to improved efficiency and accuracy in a variety of applications, from autonomous vehicles to recommendation systems. For project managers, understanding RL can provide a competitive advantage by fostering innovation and efficiency.
Build powerful workflows with Meegle for free!
Exploring the historical backdrop of reinforcement learning
The concept of RL can be traced back to the field of psychology and the study of how organisms learn from their experiences. Over time, this concept was adopted and refined by computer scientists, leading to the development of modern RL algorithms.
A significant milestone in the history of RL was the development of the Q-learning algorithm in the 1980s. This algorithm allowed an agent to learn an optimal policy in complex environments, marking a significant step forward for the field.
Understanding key technologies and methodologies in reinforcement learning
RL utilizes several key technologies, including neural networks, deep learning, and artificial intelligence. These technologies enable the RL agent to process complex data and learn from its experiences effectively.
Methodologies in RL involve setting up the learning environment, defining the reward structure, and implementing the learning algorithm. Different methodologies may be used depending on the specific problem being addressed and the desired outcomes.
Case studies: reinforcement learning in real-world scenarios
Several real-world case studies illustrate the practical applications of RL. For example, RL has been used by Google's DeepMind to train a computer program to play the board game Go, resulting in a program that can beat human world champions.
In another case, RL was used by an e-commerce company to optimize its recommendation system, leading to increased sales and customer satisfaction.
Navigating challenges and limitations of reinforcement learning
Despite its potential, RL also comes with several challenges and limitations. For instance, defining the right reward structure can be difficult, and training an RL agent can be time-consuming and resource-intensive.
In addition, ethical concerns have been raised about the use of RL, particularly in relation to autonomous decision-making systems.
Anticipating the future of reinforcement learning
The future of RL is likely to be shaped by ongoing advancements in technology and methodology. Emerging trends include the use of RL in combination with other types of machine learning, and the development of more efficient and effective learning algorithms.
As RL technology continues to improve, it's likely that its use will become more widespread across various industries.
Keep Reading
Evaluating economic and social impact of reinforcement learning
The economic implications of RL are significant, with the potential to automate many jobs and create new ones. However, there is also the risk of job displacement, particularly in industries that are heavily reliant on manual labor.
In terms of social implications, public perception of RL varies widely, with some people expressing concern about the potential misuse of technology and others highlighting the benefits that RL can bring.
Regulatory and ethical considerations of reinforcement learning
The use of RL is subject to various regulatory requirements, which can vary depending on the specific application and jurisdiction. These regulations can impact how RL is developed and deployed.
Ethical considerations are also crucial, particularly in relation to the use of RL in decision-making systems. These systems must be designed to operate in a way that is fair, transparent, and respects individual rights.
Keep Reading
Conclusion: reinforcement learning and its potential impact
RL is a powerful tool with significant potential for innovation in various industries. However, its use also comes with challenges and ethical considerations that must be addressed.
For project managers, understanding RL can provide a competitive advantage, fostering innovation and efficiency in project management.
Step-by-step guide: implementing reinforcement learning in project management
- Define the problem and the desired outcomes.
- Set up the learning environment.
- Define the reward structure.
- Select and implement the learning algorithm.
- Train the RL agent.
- Evaluate the agent's performance and fine-tune as necessary.
Keep Reading
Do's and don'ts when implementing reinforcement learning
Do's | Don'ts |
---|---|
Do define a clear problem and desired outcomes | Don't rush into implementation without proper planning |
Do select an appropriate learning environment | Don't neglect to consider the ethical implications |
Do evaluate the agent's performance regularly | Don't ignore potential regulatory requirements |
Build powerful workflows with Meegle for free!
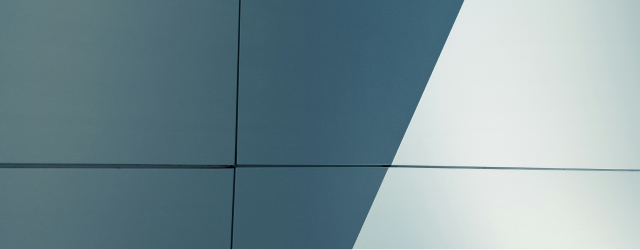
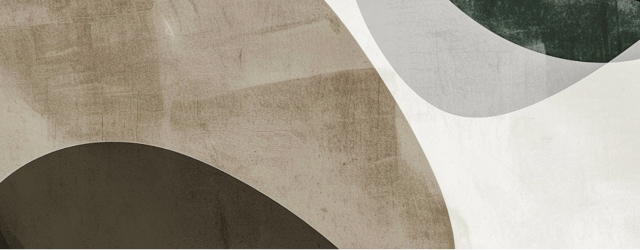
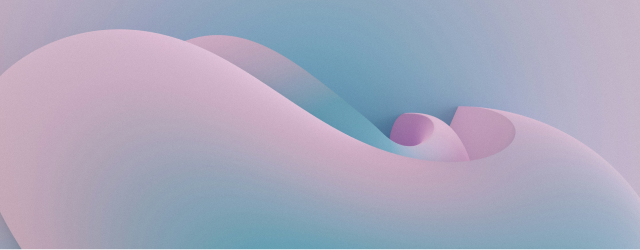
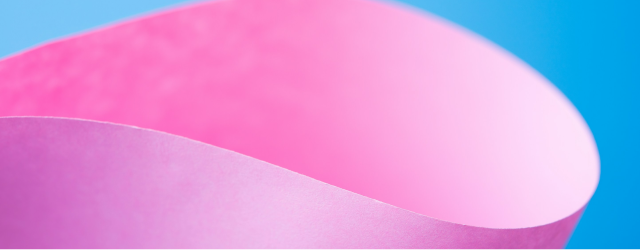
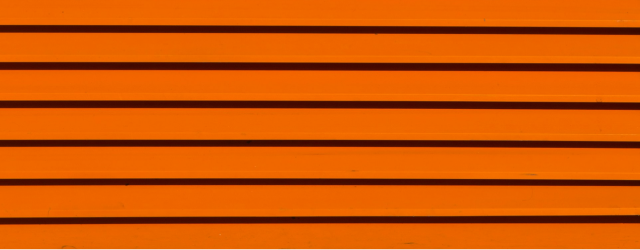
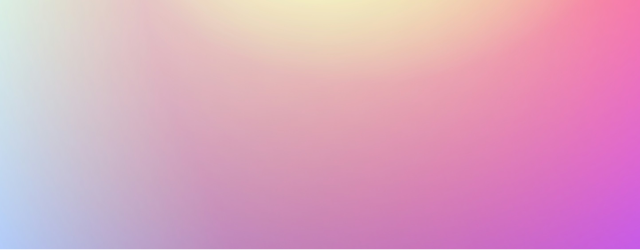
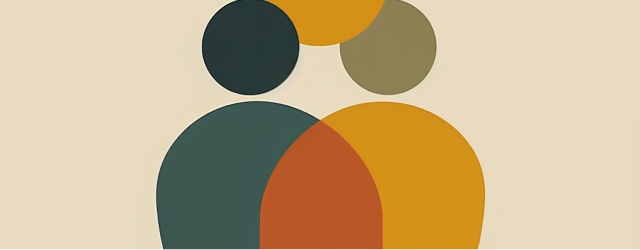
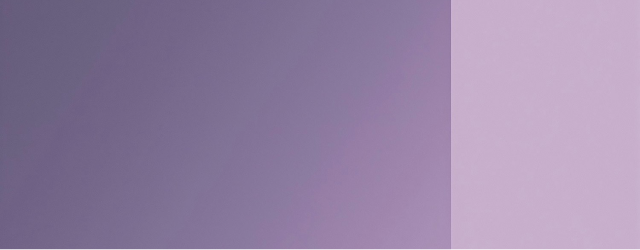